Sample-Efficient Kernel Mean Estimator with Marginalized Corrupted Data
KDD '22: Proceedings of the 28th ACM SIGKDD Conference on Knowledge Discovery and Data Mining(2022)
摘要
Estimating the kernel mean in a reproducing kernel Hilbert space is central to many kernel-based learning algorithms. Given a finite sample, an empirical average is used as a standard estimation of the target kernel mean. Prior works have shown that better estimators can be constructed by shrinkage methods. In this work, we propose to corrupt data examples with noise from known distributions and present a new kernel mean estimator, called the marginalized kernel mean estimator, which estimates kernel mean under the corrupted distributions. Theoretically, we justify that the marginalized kernel mean estimator introduces implicit regularization in kernel mean estimation. Empirically, on a variety of tasks, we show that the marginalized kernel mean estimator is sample-efficient and obtains much lower estimation errors than the existing estimators.
更多查看译文
关键词
marginalized corrupted data,sample-efficient
AI 理解论文
溯源树
样例
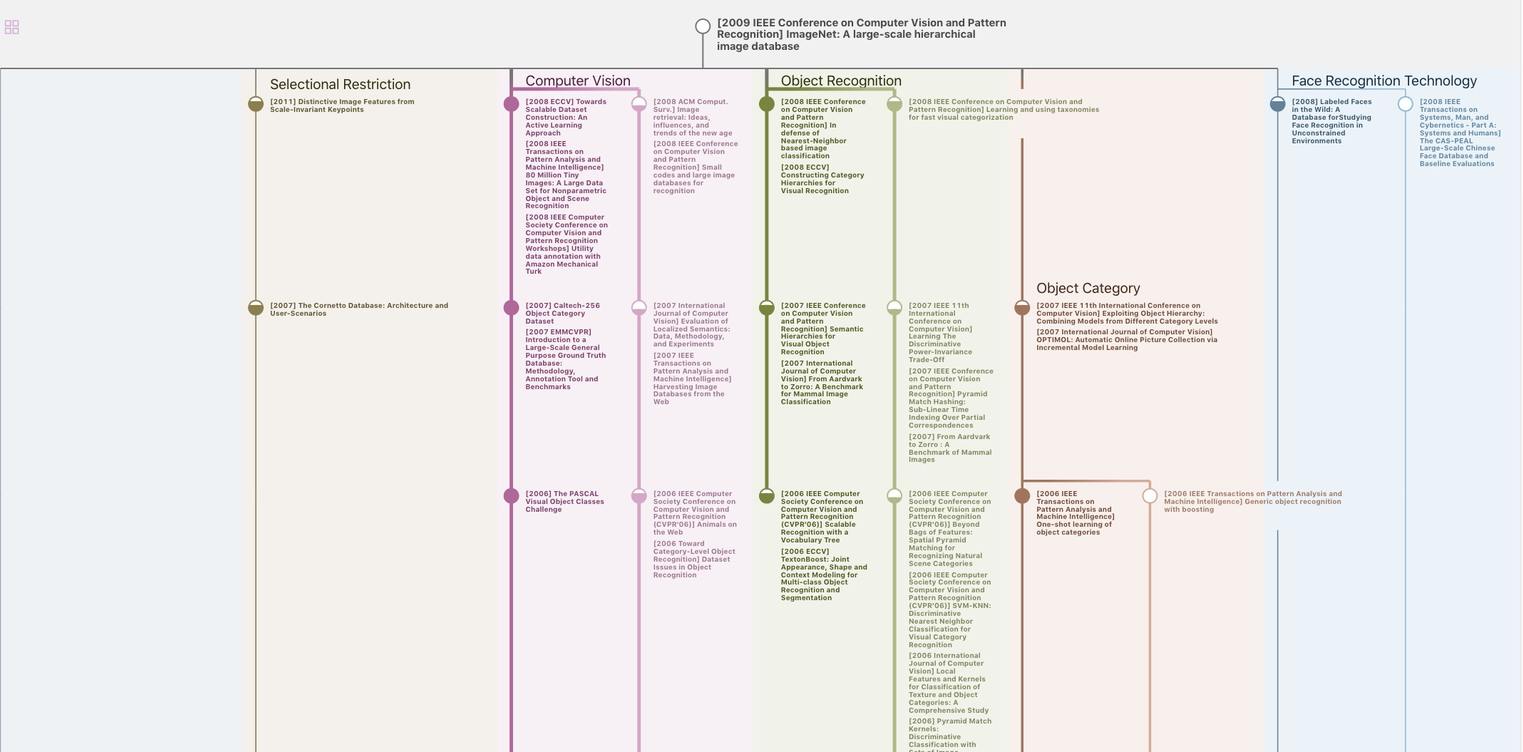
生成溯源树,研究论文发展脉络
Chat Paper
正在生成论文摘要