Myocardial Function Prediction after Coronary Artery Bypass Grafting Using MRI Radiomic Features and Machine Learning Algorithms
Journal of digital imaging(2022)
摘要
The main aim of the present study was to predict myocardial function improvement in cardiac MR (LGE-CMR) images in patients after coronary artery bypass grafting (CABG) using radiomics and machine learning algorithms. Altogether, 43 patients who had visible scars on short-axis LGE-CMR images and were candidates for CABG surgery were selected and enrolled in this study. MR imaging was performed preoperatively using a 1.5-T MRI scanner. All images were segmented by two expert radiologists (in consensus). Prior to extraction of radiomics features, all MR images were resampled to an isotropic voxel size of 1.8 × 1.8 × 1.8 mm3. Subsequently, intensities were quantized to 64 discretized gray levels and a total of 93 features were extracted. The applied algorithms included a smoothly clipped absolute deviation (SCAD)–penalized support vector machine (SVM) and the recursive partitioning (RP) algorithm as a robust classifier for binary classification in this high-dimensional and non-sparse data. All models were validated with repeated fivefold cross-validation and 10,000 bootstrapping resamples. Ten and seven features were selected with SCAD-penalized SVM and RP algorithm, respectively, for CABG responder/non-responder classification. Considering univariate analysis, the GLSZM gray-level non-uniformity-normalized feature achieved the best performance (AUC: 0.62, 95
更多查看译文
关键词
Cardiac MRI,Radiomics,Machine learning,Coronary artery bypass grafting
AI 理解论文
溯源树
样例
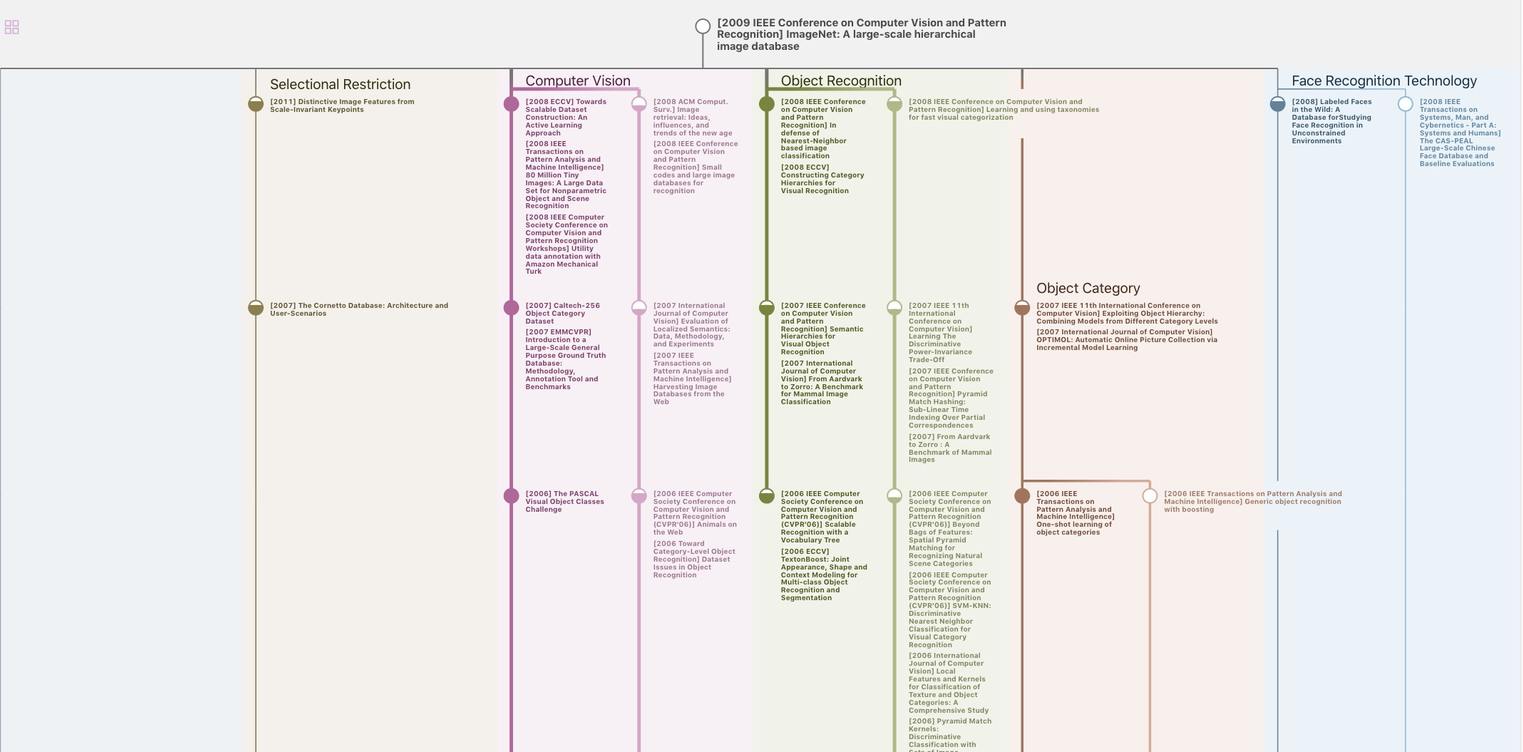
生成溯源树,研究论文发展脉络
Chat Paper
正在生成论文摘要