Premature ventricular contraction recognition using blind source separation and ensemble gaussian naive bayes weighted by analytic hierarchy process
Acta Scientiarum. Technology(2022)
摘要
Premature Ventricular Contractions (PVC) arrhythmias can be associated with sudden death and acute myocardial infarction, occurring in 50% of the population for Holter monitoring. PVC patterns are very hard to be recognized since their waveforms can be confused with other heartbeats, such as Right and Left Bundle Branch Blocks. This work proposes a new approach for PVC recognition, based on Gaussian Naive Bayes algorithm and AMUSE (Algorithm for Multiple Unknown Signal Extraction), which is a method for the blind source separation problem. This approach provides a set of attributes that are combined by Linear Discriminant Analysis, allowing the training of an ensemble learning. The Analytic Hierarchy Process weights each learned model according to its importance, obtained from the performance metrics. This approach has some advantages over baseline methods since it does not use a pre-processing stage and employs a simple machine learning model trained using only two parameters for each feature. Using a standard dataset for training and test phases, the proposed approach achieves 98.75% accuracy, 90.65% sensitivity, and 99.46% specificity. The best performance was 99.57% accuracy, 98.64% sensitivity, and 99.65% specificity for other datasets. In general, the proposed approach is better than 66% of the state-of-the-art methods concerning accuracy
更多查看译文
关键词
premature ventricular contraction recognition,blind source separation,ensemble gaussian,naive bayes
AI 理解论文
溯源树
样例
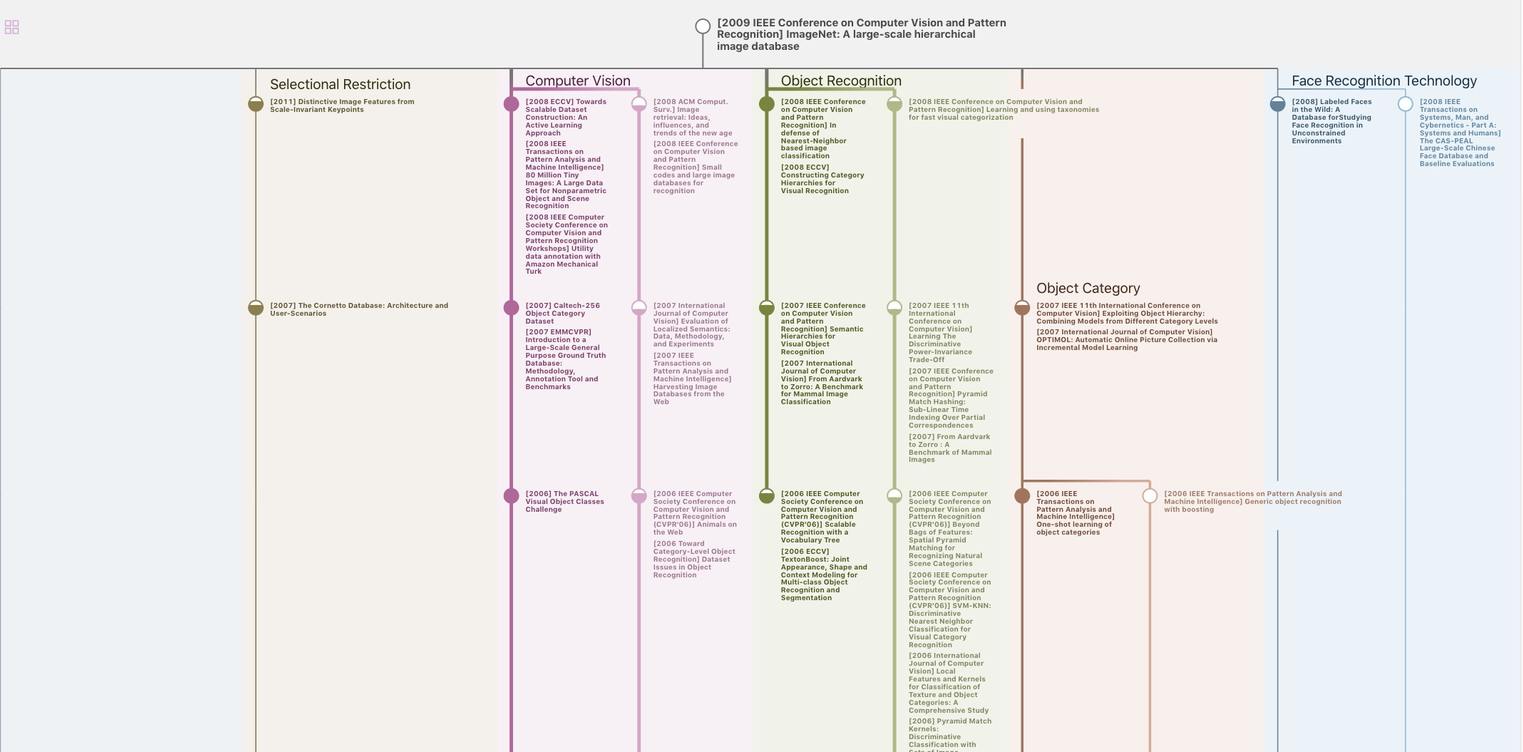
生成溯源树,研究论文发展脉络
Chat Paper
正在生成论文摘要