Blind Image Deblurring with Unknown Kernel Size and Substantial Noise
International Journal of Computer Vision(2024)
摘要
Blind image deblurring (BID) has been extensively studied in computer vision and adjacent fields. Modern methods for BID can be grouped into two categories: single-instance methods that deal with individual instances using statistical inference and numerical optimization, and data-driven methods that train deep-learning models to deblur future instances directly. Data-driven methods can be free from the difficulty in deriving accurate blur models, but are fundamentally limited by the diversity and quality of the training data—collecting sufficiently expressive and realistic training data is a standing challenge. In this paper, we focus on single-instance methods that remain competitive and indispensable. However, most such methods do not prescribe how to deal with unknown kernel size and substantial noise, precluding practical deployment. Indeed, we show that several state-of-the-art (SOTA) single-instance methods are unstable when the kernel size is overspecified, and/or the noise level is high. On the positive side, we propose a practical BID method that is stable against both, the first of its kind. Our method builds on the recent ideas of solving inverse problems by integrating physical models and structured deep neural networks, without extra training data. We introduce several crucial modifications to achieve the desired stability. Extensive empirical tests on standard synthetic datasets, as well as real-world NTIRE2020 and RealBlur datasets, show the superior effectiveness and practicality of our BID method compared to SOTA single-instance as well as data-driven methods. The code of our method is available at https://github.com/sun-umn/Blind-Image-Deblurring .
更多查看译文
关键词
Blind image deblurring,Blind deconvolution,Unknown kernel size,Unknown noise type,Unknown noise level,Deep image prior,Deep generative models,Untrained neural network priors
AI 理解论文
溯源树
样例
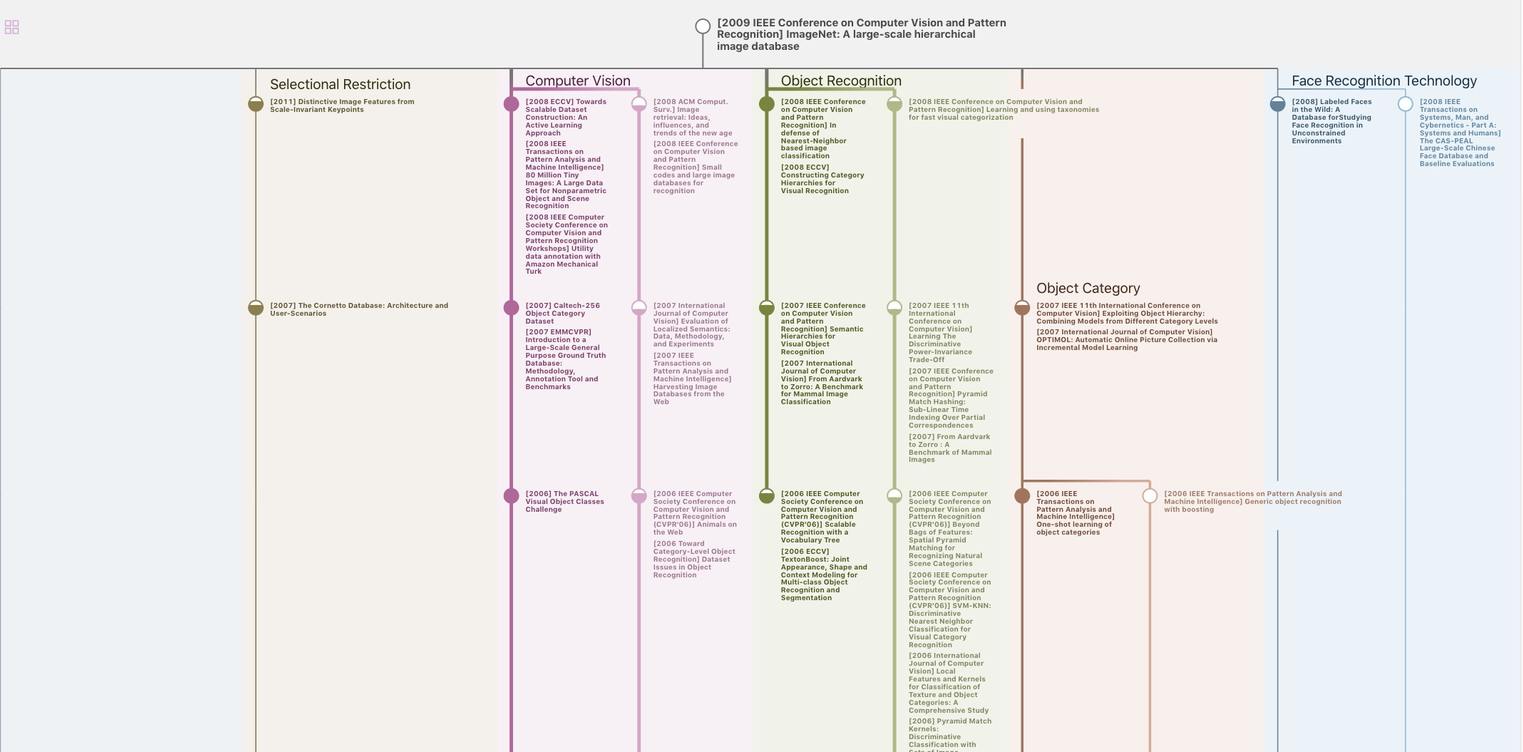
生成溯源树,研究论文发展脉络
Chat Paper
正在生成论文摘要