A Diverse Large-Scale Building Dataset and a Novel Plug-and-Play Domain Generalization Method for Building Extraction
arxiv(2023)
摘要
In this article, we introduce a new building dataset and propose a novel domain generalization method to facilitate the development of building extraction from high-resolution remote sensing images. The problem with the current building datasets involves that they lack diversity to train a practical learning model with good generalization ability, and the quality of the labels is unsatisfactory. To address these issues, we built a diverse, large-scale, and high-quality building dataset named the WHU-Mix building dataset, which is more practice-oriented. The WHU-Mix building dataset consists of a training/validation set containing 43 727 diverse images collected from all over the world, and a test set containing 8402 images from five other cities on five continents. In addition, to further improve the generalization ability of a building extraction model, we propose a domain generalization method named batch style mixing (BSM), which can be embedded as an efficient plug-and-play module in the front-end of a building extraction model, providing the model with a progressively larger data distribution to learn data-invariant knowledge. The experiments conducted in this article confirmed the potential of the WHU-Mix building dataset to improve the performance of a building extraction model, resulting in a 6-36% improvement in mean intersection over union (mIoU), compared to the other existing datasets. The adverse impact of the inaccurate labels in the other datasets can cause about 20% intersection over union decrease. The experiments also confirmed the high performance of the proposed BSM module in enhancing the generalization ability and robustness of a model, exceeding the baseline model without domain generalization by 13% and the recent domain generalization methods by 4-15% in mIoU. The WHU-Mix dataset is available at http://gpcv.whu.edu.cn/data.
更多查看译文
关键词
Buildings,Data models,Training,Deep learning,Urban areas,Remote sensing,Data mining,Building extraction,deep learning,domain generalization,remote sensing building dataset
AI 理解论文
溯源树
样例
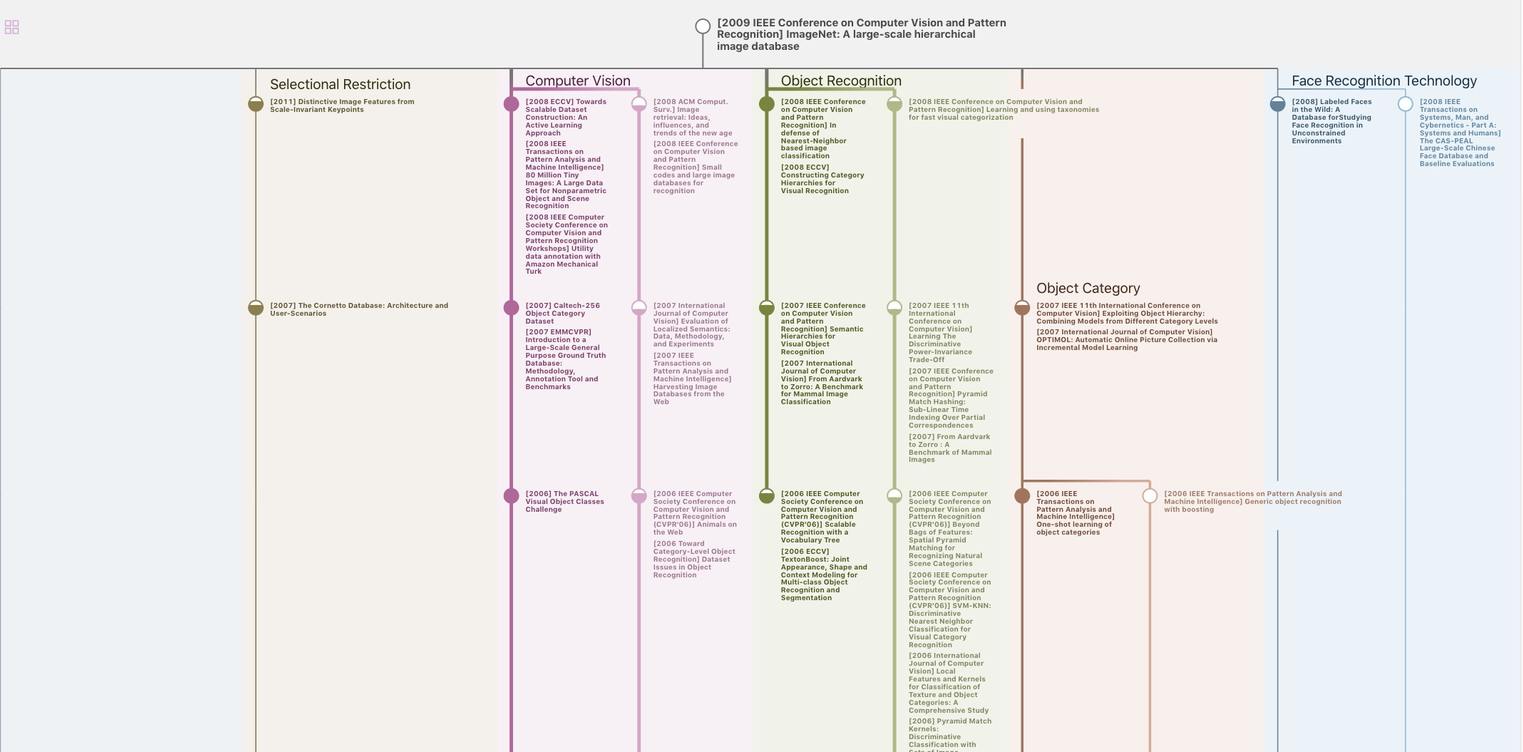
生成溯源树,研究论文发展脉络
Chat Paper
正在生成论文摘要