Socially Fair Center-Based and Linear Subspace Clustering
MACHINE LEARNING AND KNOWLEDGE DISCOVERY IN DATABASES: RESEARCH TRACK, ECML PKDD 2023, PT I(2023)
摘要
Center-based clustering (e.g., k-means, k-medians) and clustering using linear subspaces are the two most popular objectives for partitioning real-world data into smaller clusters. Both these objectives minimize the average cost of clustering over all the points. However, when the points belong to different sensitive demographic groups and the optimal clustering has a significantly different cost per point for different groups, it can cause fairness-related harms (e.g., different quality-of-service). To mitigate these harms, the socially fair clustering objective minimizes the cost of clustering per point for the worst-off group. In this work, we propose a unified framework to solve socially fair center-based and linear subspace clustering and give practical and efficient approximation algorithms for these problems. We perform extensive experiments to show that our algorithms closely match or outperform existing baselines on multiple benchmark datasets.
更多查看译文
AI 理解论文
溯源树
样例
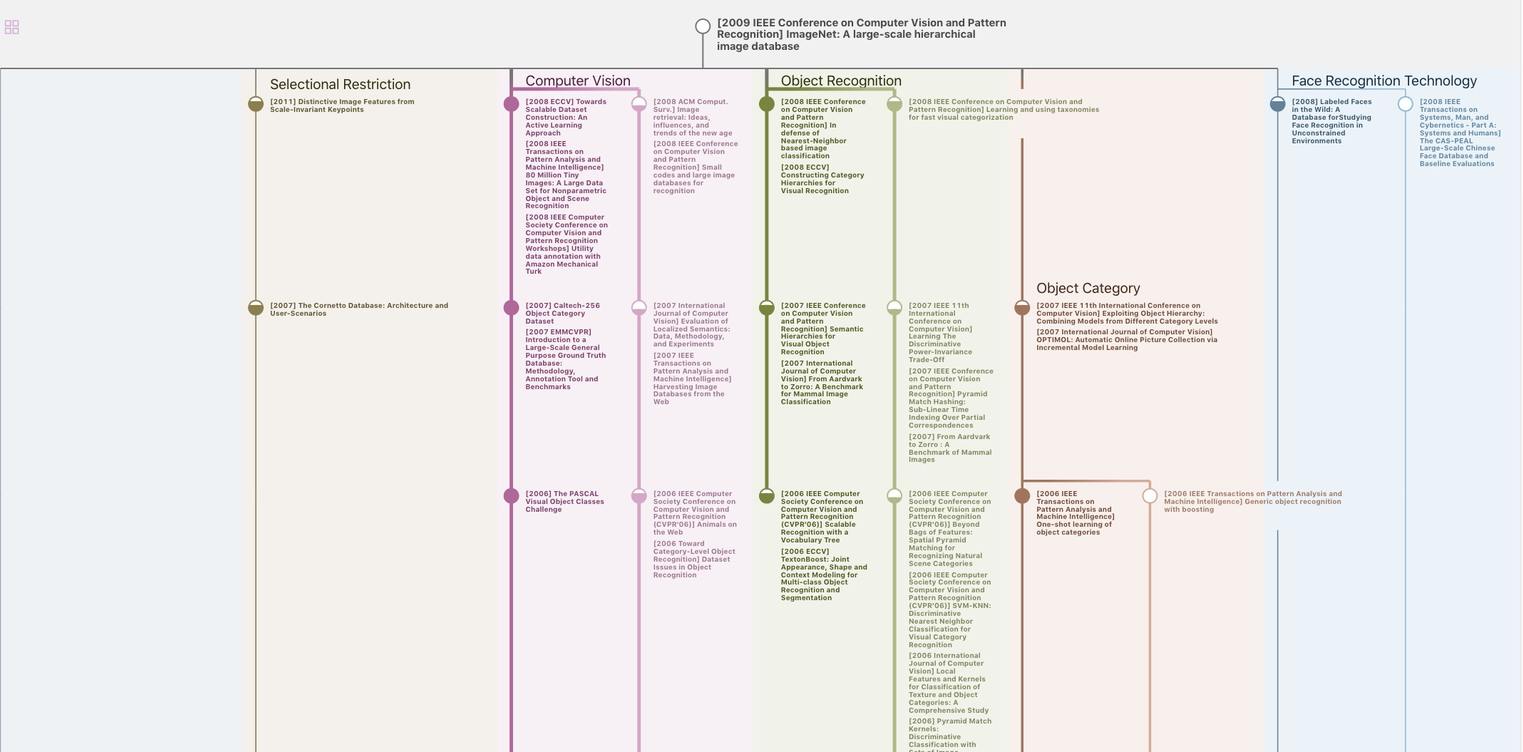
生成溯源树,研究论文发展脉络
Chat Paper
正在生成论文摘要