Predicting the protein-ligand affinity from molecular dynamics trajectories
arxiv(2022)
摘要
Accurate protein-ligand binding affinity prediction is essential in drug design and many other molecular recognition problems. Despite many advances in affinity prediction based on machine learning techniques, they are still limited since the protein-ligand binding is determined by the dynamics of atoms and molecules. To this end, we curated an MD dataset containing 3,218 dynamic protein-ligand complexes and further developed Dynaformer, a graph-based deep learning framework. Dynaformer can fully capture the dynamic binding modes by considering various geometric characteristics of the interaction. Our method shows superior performance on the benchmark dataset over the methods hitherto reported. Moreover, we performed virtual drug-screening on heat shock protein 90 (HSP90) by integrating our model with structure-based docking. We benchmarked our performance against the conventional knowledge-based approach, demonstrating that our method can identify two lead compounds with submicromolar activity (0.207\textmu{}M and 0.517\textmu{}M). We anticipate that large-scale MD dataset and machine learning models will form a new synergy, providing a new route toward accelerated drug discovery.
更多查看译文
AI 理解论文
溯源树
样例
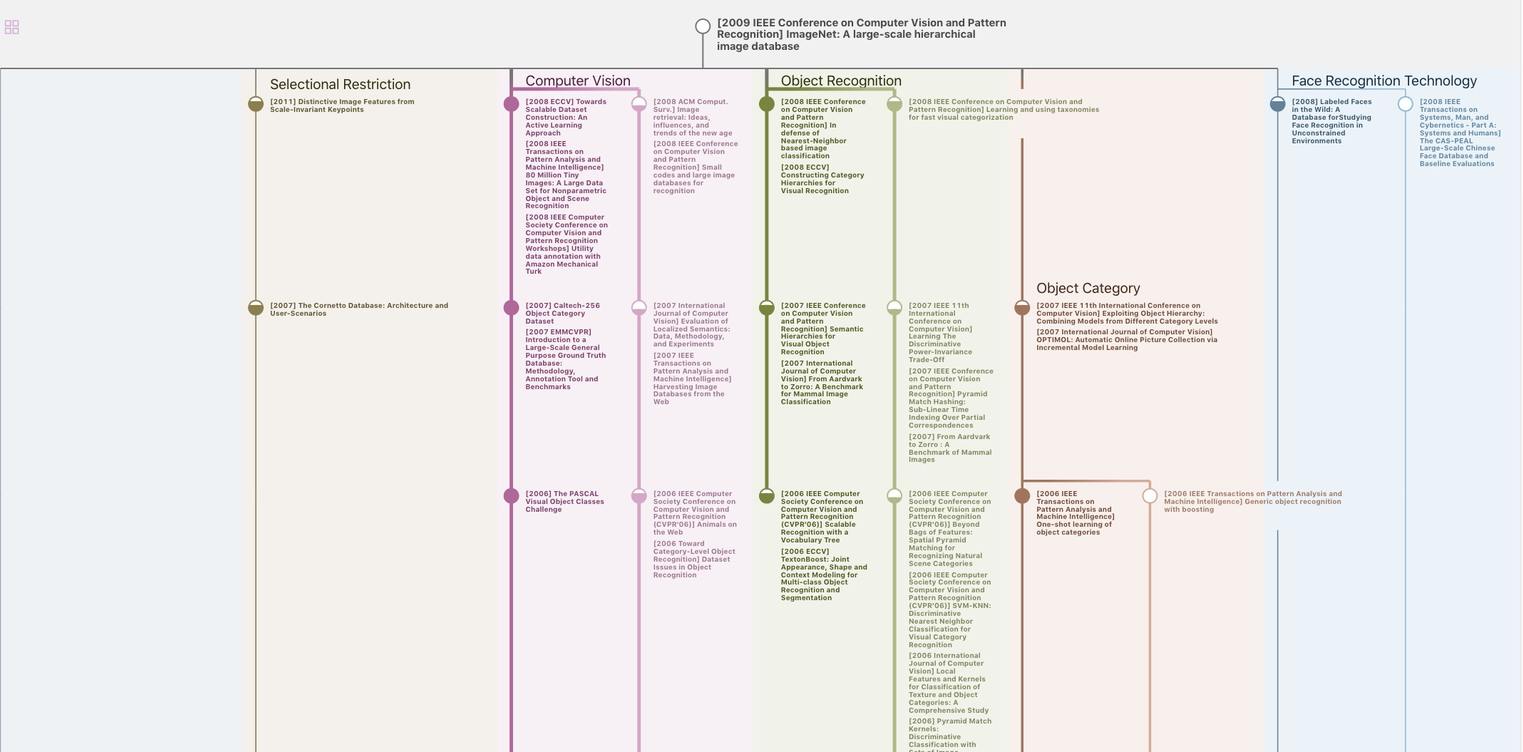
生成溯源树,研究论文发展脉络
Chat Paper
正在生成论文摘要