Learning Mappings between Equilibrium States of Liquid Systems Using Normalizing Flows
arXiv (Cornell University)(2022)
Abstract
Generative models are a promising tool to address the sampling problem in multi-body and condensed-matter systems in the framework of statistical mechanics. In this work, we show that normalizing flows can be used to learn a transformation to map different liquid systems into each other allowing at the same time to obtain an unbiased equilibrium distribution through a reweighting process. Two proof-of-principles calculations are presented for the transformation between Lennard-Jones systems of particles with different depths of the potential well and for the transformation between a Lennard-Jones and a system of repulsive particles. In both numerical experiments, systems are in the liquid state. In future applications, this approach could lead to efficient methods to simulate liquid systems at ab-initio accuracy with the computational cost of less accurate models, such as force field or coarse-grained simulations.
MoreTranslated text
Key words
normalizing flows,liquid systems,equilibrium states,mappings
AI Read Science
Must-Reading Tree
Example
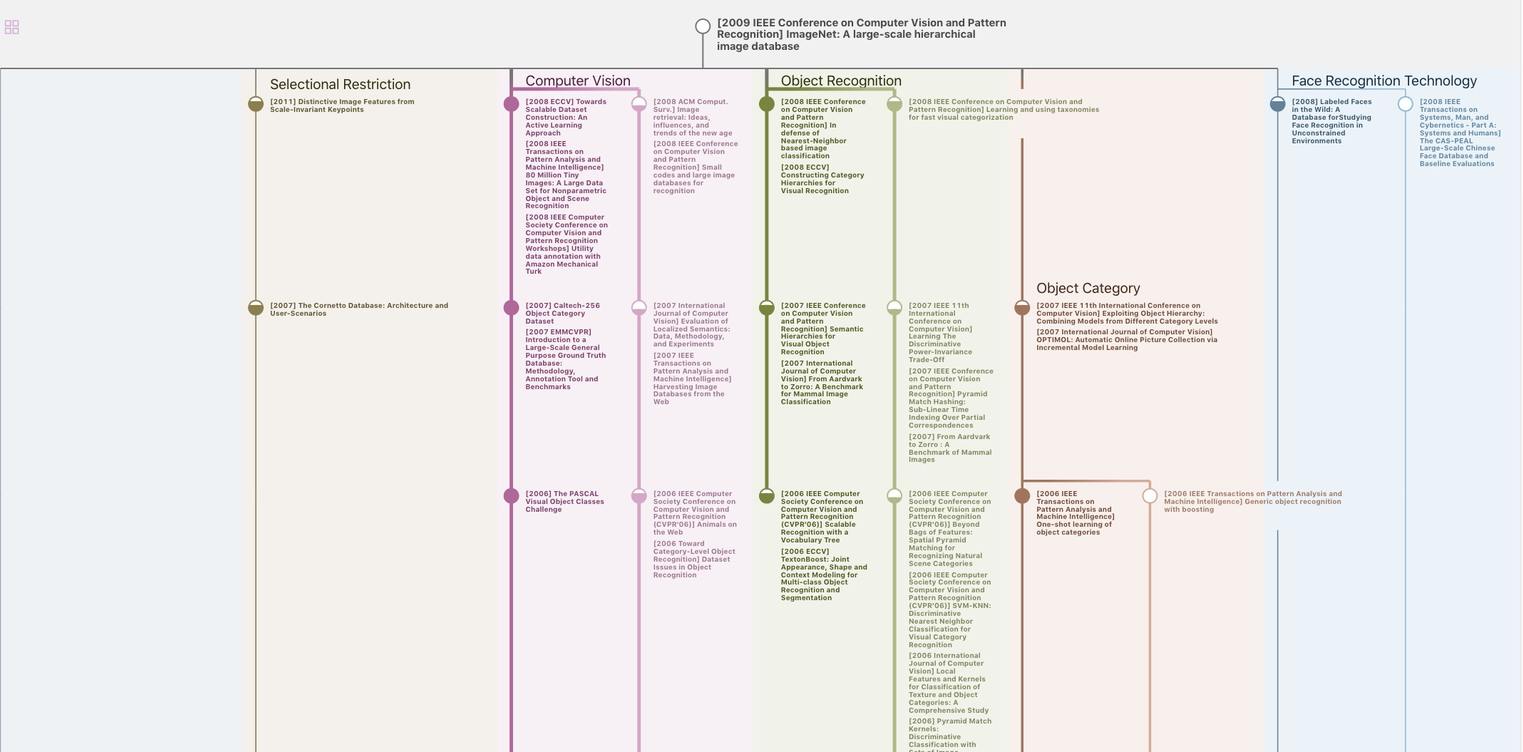
Generate MRT to find the research sequence of this paper
Chat Paper
Summary is being generated by the instructions you defined