Canopy Height Mapping by Sentinel 1 and 2 Satellite Images, Airborne LiDAR Data, and Machine Learning
REMOTE SENSING(2022)
摘要
Continuous mapping of vegetation height is critical for many forestry applications, such as planning vegetation management in power transmission line right-of-way. Satellite images from different sensors, including SAR (Synthetic Aperture Radar) from Sentinel 1 (S1) and multispectral from Sentinel 2 (S2), can be used for producing high-resolution vegetation height maps at a broad scale. The main objective of this study is to assess the potential of S1 and S2 satellite data, both in a single and a multisensor approach, for modeling canopy height in a transmission line right-of-way located in the Atlantic Forest of Parana, Brazil. For integrating S1 and S2 data, we used three machine learning algorithms (LR: Linear Regression, CART: Classification and Regression Trees, and RF: Random Forest) and airborne LiDAR (Light Detection and Ranging) measurements as the reference height. The best models were obtained using the RF algorithm and 20 m resolution features from only S2 data (cross-validated RMSE of 4.92 m and R-2 of 0.58) or multisensor data (cross-validated RMSE of 4.86 m and R-2 of 0.60). Although the multisensor model presented the best performance, it was not statistically different from the single-S2 model. Thus, the use of only S2 to estimate canopy height has practical advantages, as it reduces the need to process SAR images and the uncertainties due to S1 noise or differences between the acquisition dates of S2 and S1.
更多查看译文
关键词
canopy height,vegetation structure,airborne laser scanning (ALS),multispectral remote sensing,synthetic aperture radar (SAR),multisensor modeling
AI 理解论文
溯源树
样例
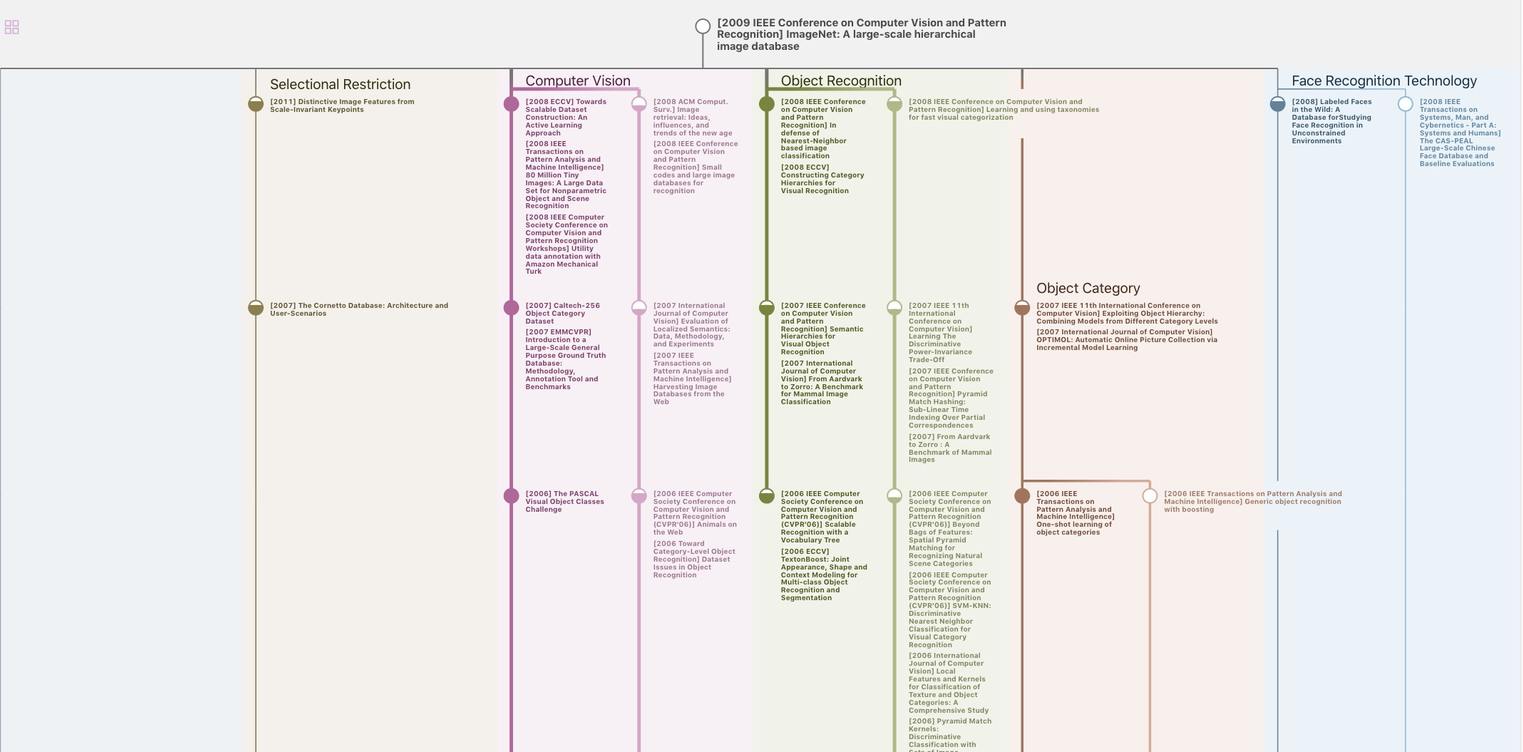
生成溯源树,研究论文发展脉络
Chat Paper
正在生成论文摘要