Adaptive Bitrate Quantization Scheme Without Codebook for Learned Image Compression
2022 IEEE/CVF Conference on Computer Vision and Pattern Recognition Workshops (CVPRW)(2022)
摘要
We propose a generic approach to quantization without codebook in learned image compression called one-hot max (OHM, Ω) quantization. It reorganizes the feature space resulting in an additional dimension, along which vector quantization yields one-hot vectors by comparing activations. Furthermore, we show how to integrate Ω quantization into a compression system with bitrate adaptation, i.e., full control over bitrate during inference. We perform experiments on both MNIST and Kodak and report on rate-distortion trade-offs comparing with the integer rounding reference. For low bitrates (< 0.4 bpp), our proposed quantizer yields better performance while exhibiting also other advantageous training and inference properties. Code is available at https://github.com/ifnspaml/OHMQ.
更多查看译文
关键词
learned image compression,codebook
AI 理解论文
溯源树
样例
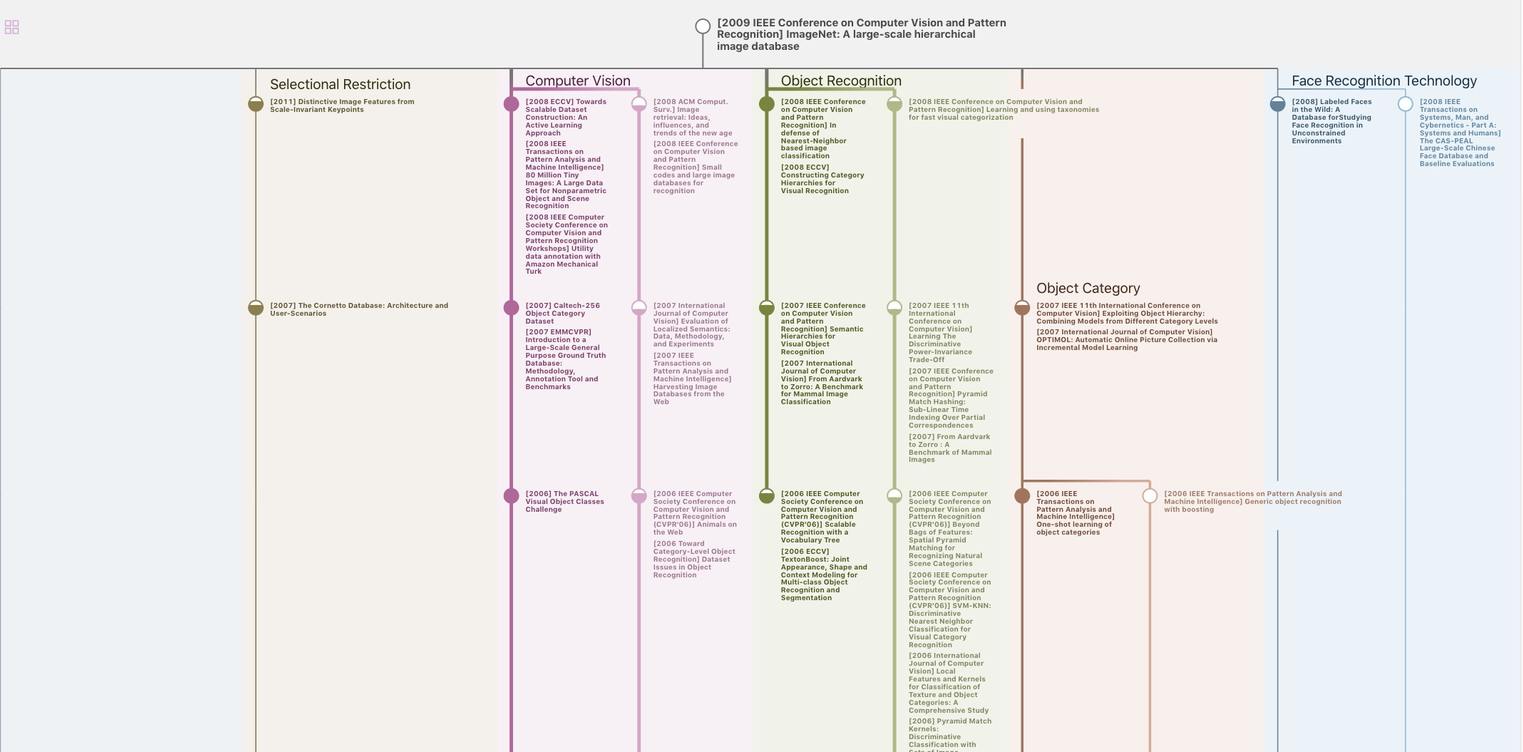
生成溯源树,研究论文发展脉络
Chat Paper
正在生成论文摘要