Low Voltage State Estimation Based Operation Analysis of Smart Distribution Assets
2022 8th International Youth Conference on Energy (IYCE)(2022)
关键词
Smart grid,Distribution system,State estimation,Observability,Smart asset
AI 理解论文
溯源树
样例
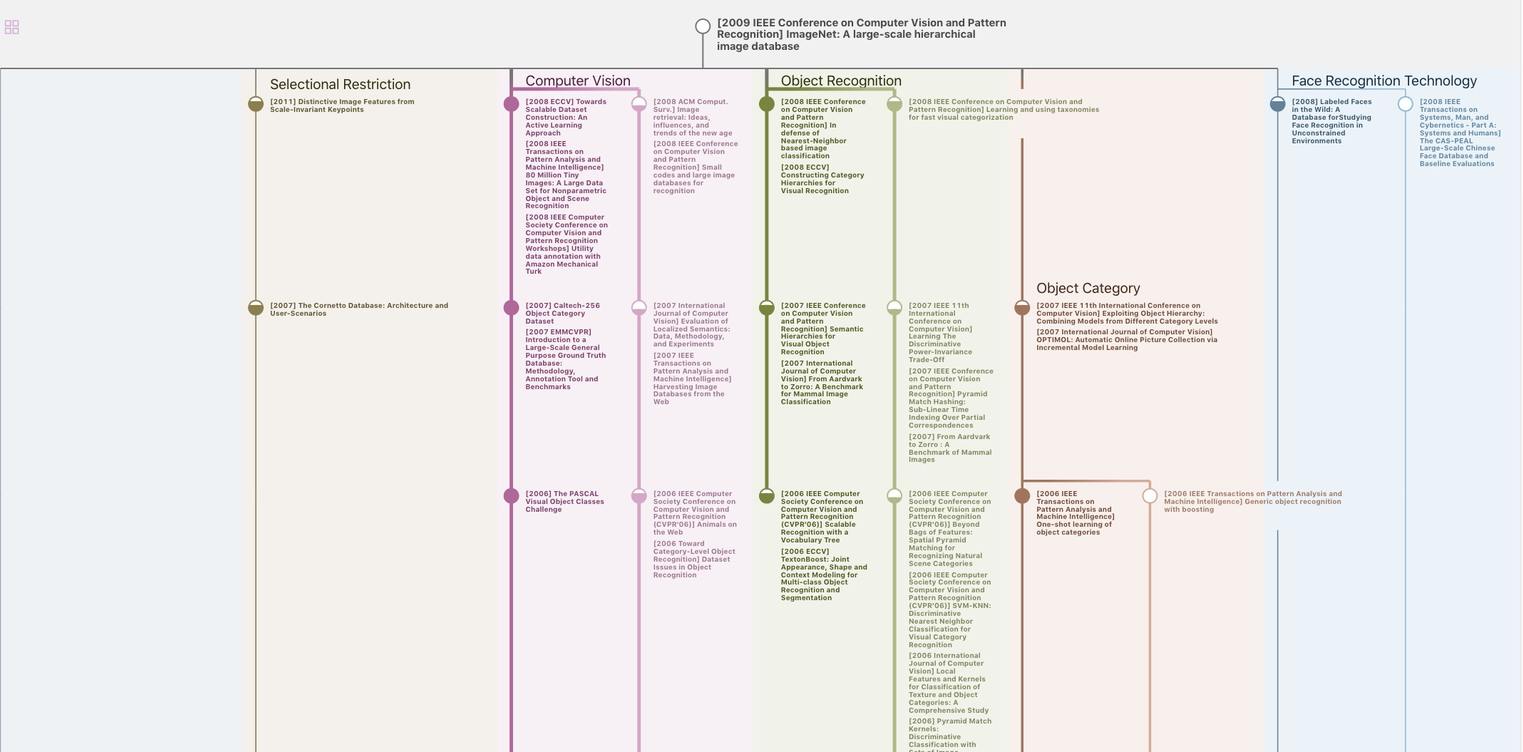
生成溯源树,研究论文发展脉络
Chat Paper
正在生成论文摘要