User-Guided Data Expansion Modeling to Train Deep Neural Networks With Little Supervision
IEEE GEOSCIENCE AND REMOTE SENSING LETTERS(2022)
摘要
Image segmentation is a challenging and essential task in remote sensing. Deep neural networks (DNNs) have successfully segmented images from different domains, but the models usually require time-consuming and expensive pixel-level data annotation. In this letter, we exploit a recent technique to learn features (an encoder) from a few markers placed by the user in relevant image regions, build an encoder-decoder model from a small set of regions delineated by click-based segmentation, and use that model to annotate the remaining pixels. Such user-guided data expansion modeling can be repeated as the encoder-decoder network improves, and by selecting well-annotated regions, the user considerably expands the pixel set to train DNNs with little supervision. We show the role of feature learning from image markers (FLIM) and that our data expansion model can significantly improve the generalization performance of a state-of-the-art DNN when segmenting buildings in aerial images of distinct cities.
更多查看译文
关键词
Image segmentation,Training,Annotations,Data models,Urban areas,Buildings,Semantics,Aerial image annotation,deep learning,semantic segmentation,semi-automatic data annotation
AI 理解论文
溯源树
样例
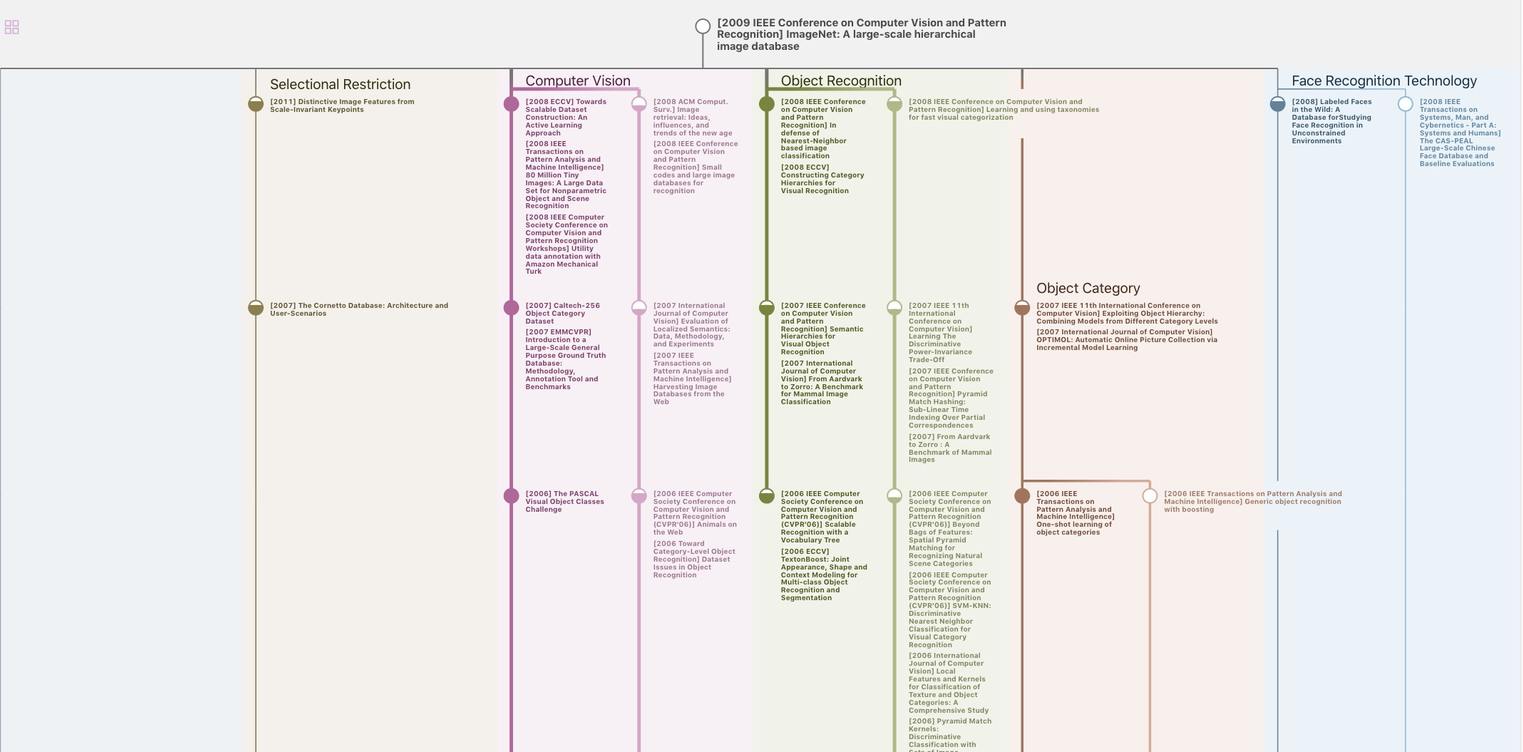
生成溯源树,研究论文发展脉络
Chat Paper
正在生成论文摘要