A dynamic feature selection and intelligent model serving for hybrid batch-stream processing
Knowledge-Based Systems(2022)
摘要
The increasing complexity of real-world applications, especially those related to the Internet of Things and cloud computing, highlights the importance of using hybrid batch-stream processing techniques to analyze big data. Hybrid processing combines the accuracy of batch processing and the speed of stream processing. Among the important challenges of this approach are selecting relevant and diverse features to build the base models and intelligently choosing which of those models to use in computing the final results. We present the H-DIFS architecture, a dynamic and intelligent feature selection approach to address these two challenges of hybrid batch-stream processing for anomaly detection. The proposed architecture employs a dynamic feature selection method based on the genetic algorithm that is fully compatible with the nature of hybrid processing and dynamically changes the models’ features over time based on the input data stream. Additionally, two components, the model evaluator and intelligent model recommender, allow for offering various batch and stream models and selecting from them based on the defined policies. The experimental results, on the datasets with various feature set sizes, indicate that the proposed architecture increases the speed of hybrid processing and anomaly detection by eliminating irrelevant and redundant features. Moreover, it increases detection accuracy by selecting appropriate models and aggregating their results.
更多查看译文
关键词
Dynamic feature selection,Model selection,Batch-stream hybrid processing,Genetic algorithm,Big data analytics
AI 理解论文
溯源树
样例
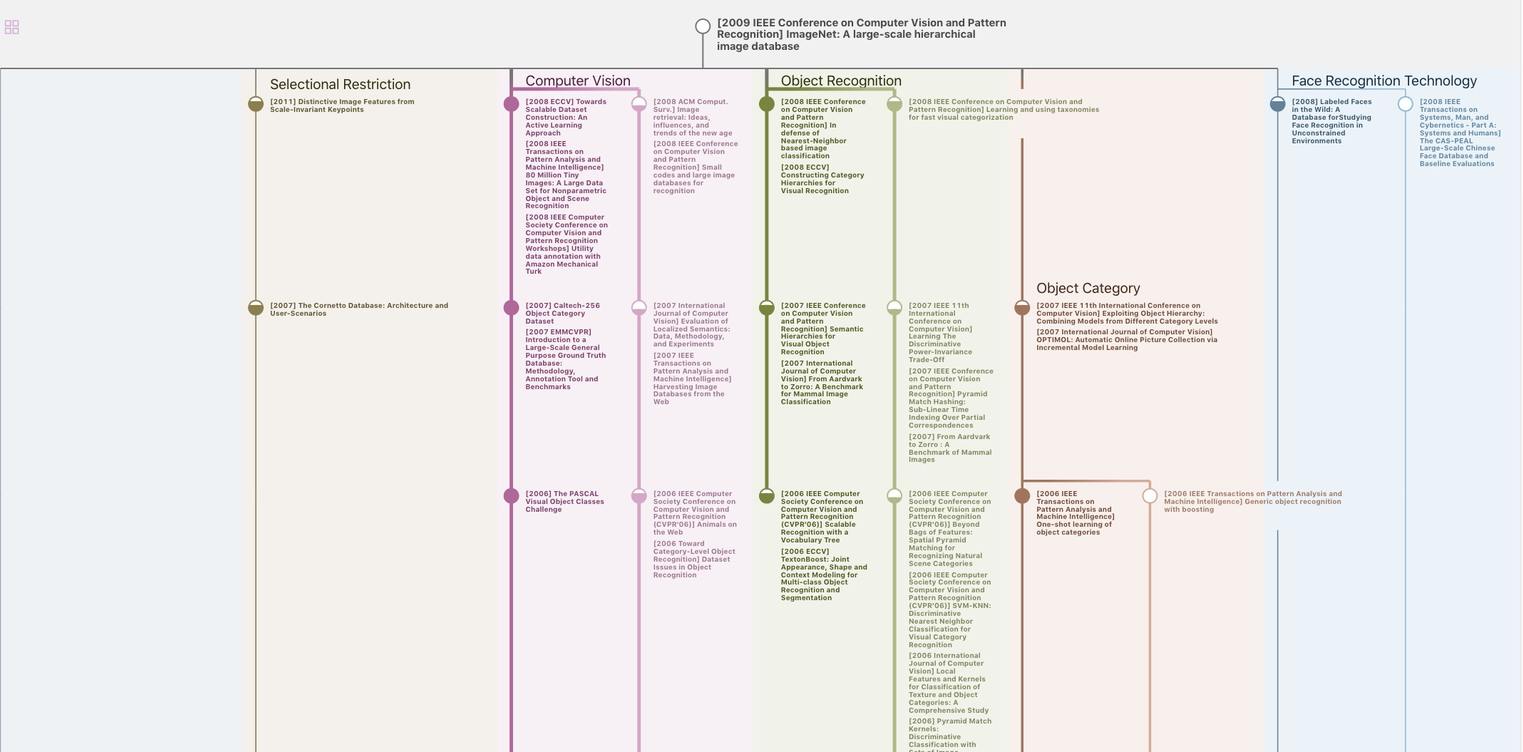
生成溯源树,研究论文发展脉络
Chat Paper
正在生成论文摘要