Alternating Layered Variational Quantum Circuits Can Be Classically Optimized Efficiently Using Classical Shadows
arxiv(2022)
摘要
Variational quantum algorithms (VQAs) are the quantum analog of classical neural networks (NNs). A VQA consists of a parameterized quantum circuit (PQC) which is composed of multiple layers of ansatzes (simpler PQCs, which are an analogy of NN layers) that differ only in selections of parameters. Previous work has identified the alternating layered ansatz as potentially a new standard ansatz in near-term quantum computing. Indeed, shallow alternating layered VQAs are easy to implement and have been shown to be both trainable and expressive. In this work, we introduce a training algorithm with an exponential reduction in training cost of such VQAs. Moreover, our algorithm uses classical shadows of quantum input data, and can hence be run on a classical computer with rigorous performance guarantees. We demonstrate 2--3 orders of magnitude improvement in the training cost using our algorithm for the example problems of finding state preparation circuits and the quantum autoencoder.
更多查看译文
关键词
layered variational quantum circuits
AI 理解论文
溯源树
样例
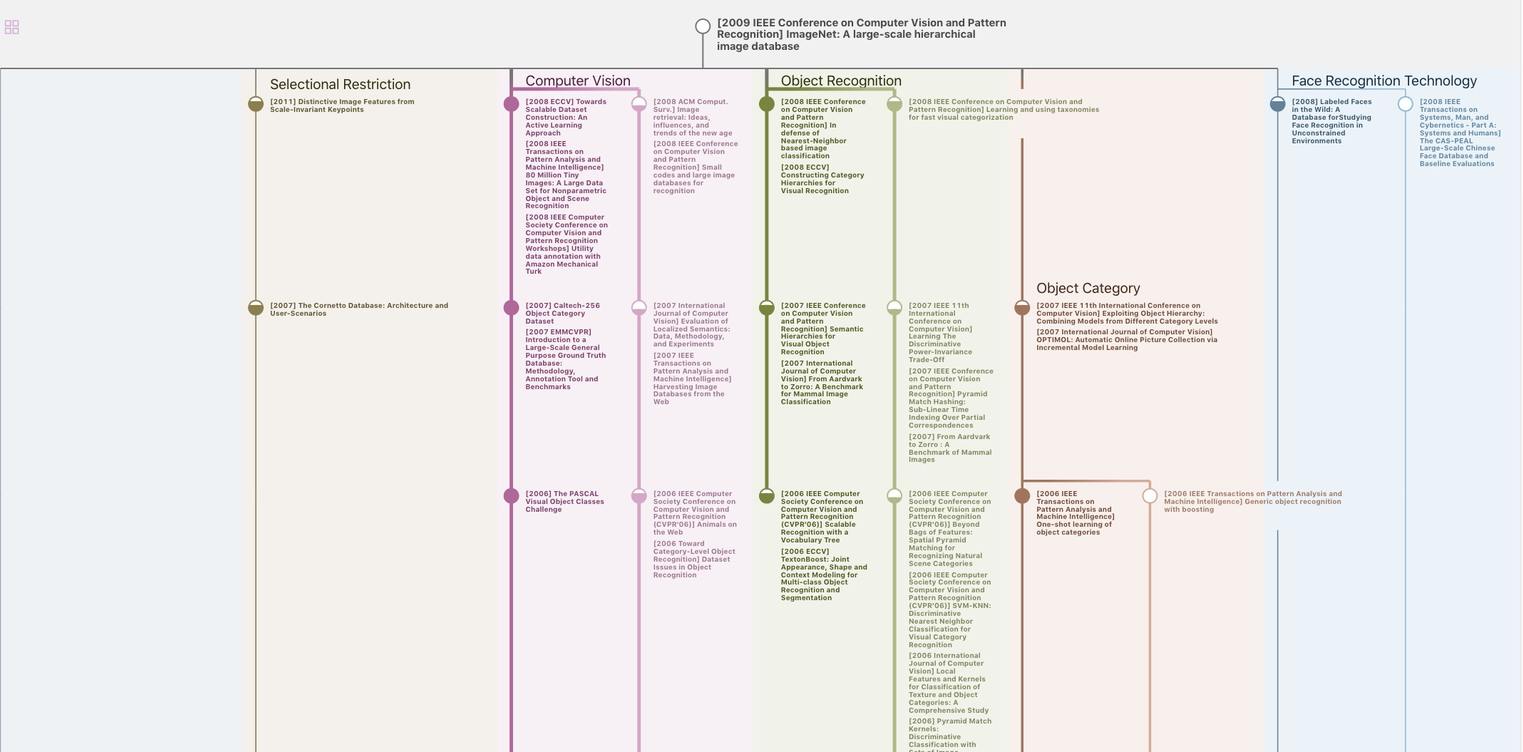
生成溯源树,研究论文发展脉络
Chat Paper
正在生成论文摘要