Autism spectrum disorder identification using multi‐model deep ensemble classifier with transfer learning
Expert Systems(2024)
摘要
AbstractIdentifying autism spectrum disorder (ASD) symptoms accurately is a challenging task. The traditional subjective diagnostic process of ASD relies on time‐consuming behavioural and psychological observations. In this study, we introduce an ensemble learning‐based classification model using an open‐access database focusing on functional magnetic resonance imaging (fMRI). We propose a novel multi‐model ensemble classifier (MMEC) and multisite ensemble classifier (MSEC) with transfer learning (TL) for ASD classification to improve the prediction accuracy. The MMEC utilizes four base classifiers, Inception V3, ResNet50, MobileNet, and DenseNet to boost the performance of the individual convolutional neural network (CNN) models. The MSEC combined the base classifiers trained from different data sites. We evaluate the two models with ensemble averaging, weighted averaging, and stacking methods. The proposed MMEC with stacking shows the state of art performance compared to MSEC, improving the prediction accuracy by 3.25%. The obtained results have shown an accuracy of 97.82%, 97.82%, and 97.78% for ensemble averaging, weighted averaging, and stacking methods, respectively, on multi‐site datasets. The ensemble classifier MMEC performed better than a single classifier on the multi‐site dataset. The proposed MMEC opens a new paradigm to design a universal ASD classification framework.
更多查看译文
AI 理解论文
溯源树
样例
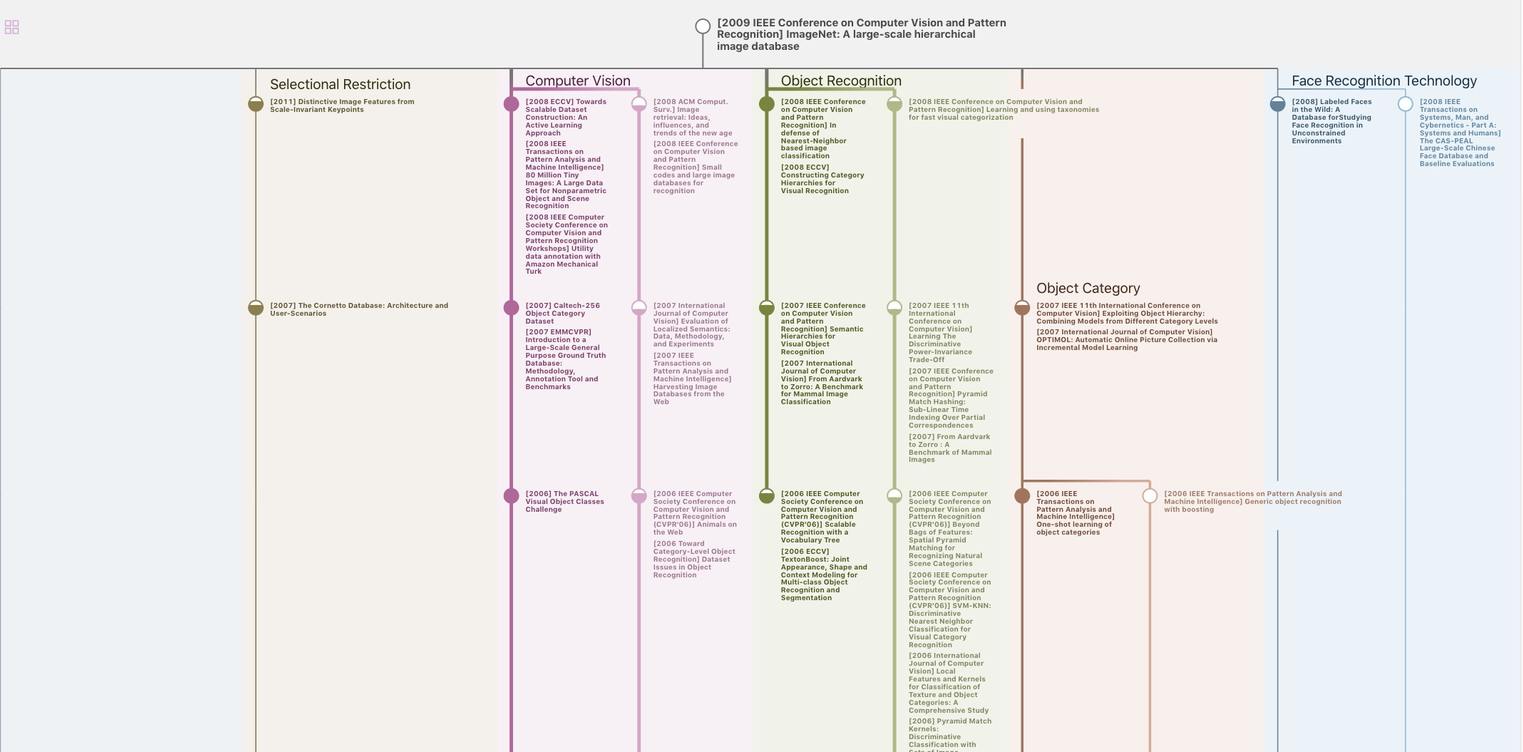
生成溯源树,研究论文发展脉络
Chat Paper
正在生成论文摘要