New Statistical Methods For Association Studies And Genomic Predictio
Proceedings of the 4th International Conference on Statistics: Theory and Applications International Conference on Statistics: Theory and Applications(2022)
摘要
Selective genotyping" is a very famous concept in genetics.It was introduced by Lebowitz et al. (1987) and was studied more in details by Lander and Botstein (1989).It consists in genotyping (collecting DNA information at specific positions) only the individuals with extreme phenotypes.Indeed, Lebowitz et al. (1987) noticed that the highest or the lowest observations contain most of the signal on Quantitative Trait Loci (QTL), i.e. genes with quantitative effect on a trait.Today, although the genotyping costs have drastically dropped, selective genotyping is still heavily used (e.g.[1]) since we can optimize the statistical experiment by focusing on extreme individuals instead of "random" individuals.Although "selective genotyping" was introduced in the eighties, biologists are still missing tools to analyze properly data sampled from this experimental design.Indeed, classical methods such as penalized regression (e.g.Lasso [2]) are not dedicated to extreme observations.As a consequence, we introduced recently the SgenoLasso [3], a new L1 penalized regression that models explicitly the extremes.SgenoLasso relies on the ``Interval Mapping" [4], a famous concept in genetics that consists in scanning the genome by testing the presence of a QTL at each location.From a statistical point of view, SgenoLasso is based on new limiting results on stochastic processes along the genome.SgenoLasso presents all the nice properties of Lasso since we have replaced the problem in a classical penalized likelihood framework.In contrast, the Lasso does not enjoy these properties in presence of extreme data.On simulated data, when only the highest (or only the lowest) individuals were genotyped, SgenoLasso and its cousins performed largely better than existing methods such as the Lasso [2], the Group Lasso [5], the Elastic Net [6], the RaLasso [7] and the BayesianLasso [8].In a second part of this talk, we will introduce the AdaptSgenoLasso a new variant of the SgenoLasso, that allows to impose more weights on some loci of interest, known to be responsible for the variation of the quantitative trait.For that, we consider a selective genotyping that varies along the genome.In other words, different amounts of selection are applied at genome locations, which was not the case for the SgenoLasso based on the classical selective genotyping.This new framework relies on two genetic maps: a dense map with a large density of markers, and a sparse map containing only a few markers.The originalty lies in the fact that we genotype extra extreme individuals at markers belonging to the sparse map.In this context, we investigate statistical properties of the so-called Likelihood Ratio Test process [9], which is the stochastic process defined by the Interval Mapping.We show that the Likelihood Ratio Test process, converges in distribution to the square of a Gaussian process described as an interpolation of two independent Gaussian processes linked to the two genetic maps.This theoretical result allows us to introduce the AdaptSgenoLasso: we give the rate of convergence for prediction and also study the consistency of the variable selection.Last, on simulated data, we will show the superiority of AdaptSgenoLasso over SgenoLasso and its cousins.
更多查看译文
关键词
association studies,statistical methods
AI 理解论文
溯源树
样例
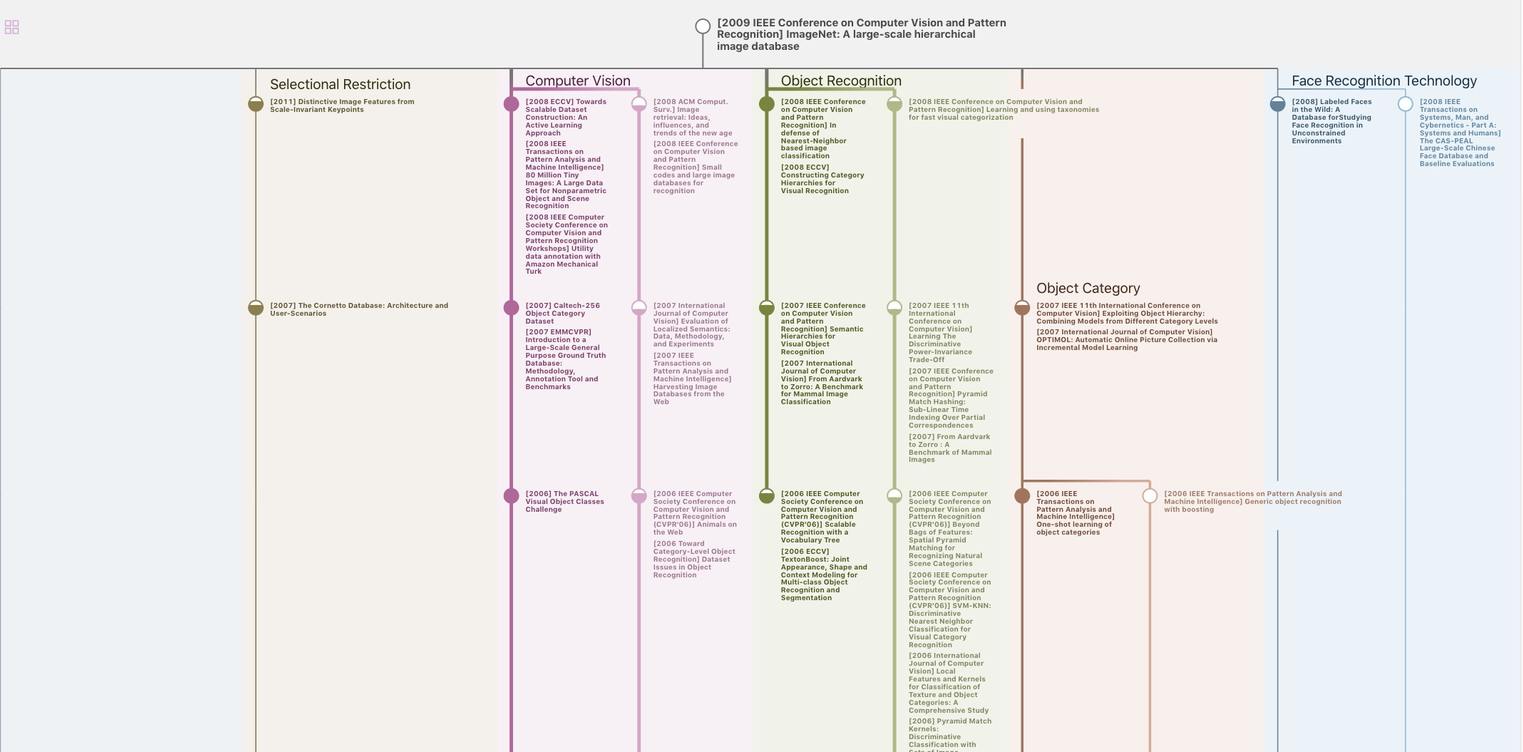
生成溯源树,研究论文发展脉络
Chat Paper
正在生成论文摘要