On LSTM Autoencoder-Based Hybrid Precoding for Reconfigurable Intelligent Surface-Aided Multiuser Millimeter-Wave Massive MIMO 6G Systems
2022 IEEE 95th Vehicular Technology Conference: (VTC2022-Spring)(2022)
摘要
The sixth-generation (6G) wireless communication networks are anticipated to combine terrestrial, aerial, and marine communications into a dependable, fast network that could handle many devices with ultra-low latency requirements. Reconfigurable intelligent surfaces (RIS)-aided millimeter-wave massive MIMO communication systems could increase wireless link quality by providing passive beamforming gain via low-cost reflecting components. On the other hand, the power consumption and cost could be reduced much by applying a hybrid precoding architecture, which combines digital and analog precoding modules. However, using RIS to solve the problem of hybrid precoding is difficult because how to figure out reflecting coefficients without beam training overhead or large channel estimation is a challenging issue. In this work, we propose a novel hybrid precoding architecture based on geometric mean decomposition (GMD) and jointly consider the design of the Long Short-Term Memory (LSTM) autoencoder scheme. That is, we adopt GMD for diminishing the computational complexity and enhancing the hybrid precoding performance. Since the encoder-decoder design of autoencoder serves as a dimensionality reduction strategy, the LSTM autoencoder are able to capture the temporal and spatial distribution of the sequential data by using the LSTM models sequential and feature extraction capabilities. Numerical results show that our proposed algorithm could significantly improve the RIS-aided millimeter-wave MIMO communication systems performance compared with previous works.
更多查看译文
关键词
sixth-generation (6G), Reconfigurable intelligent surface (RIS), Long Short-Term Memory (LSTM), hybrid precoding, Autoencoder
AI 理解论文
溯源树
样例
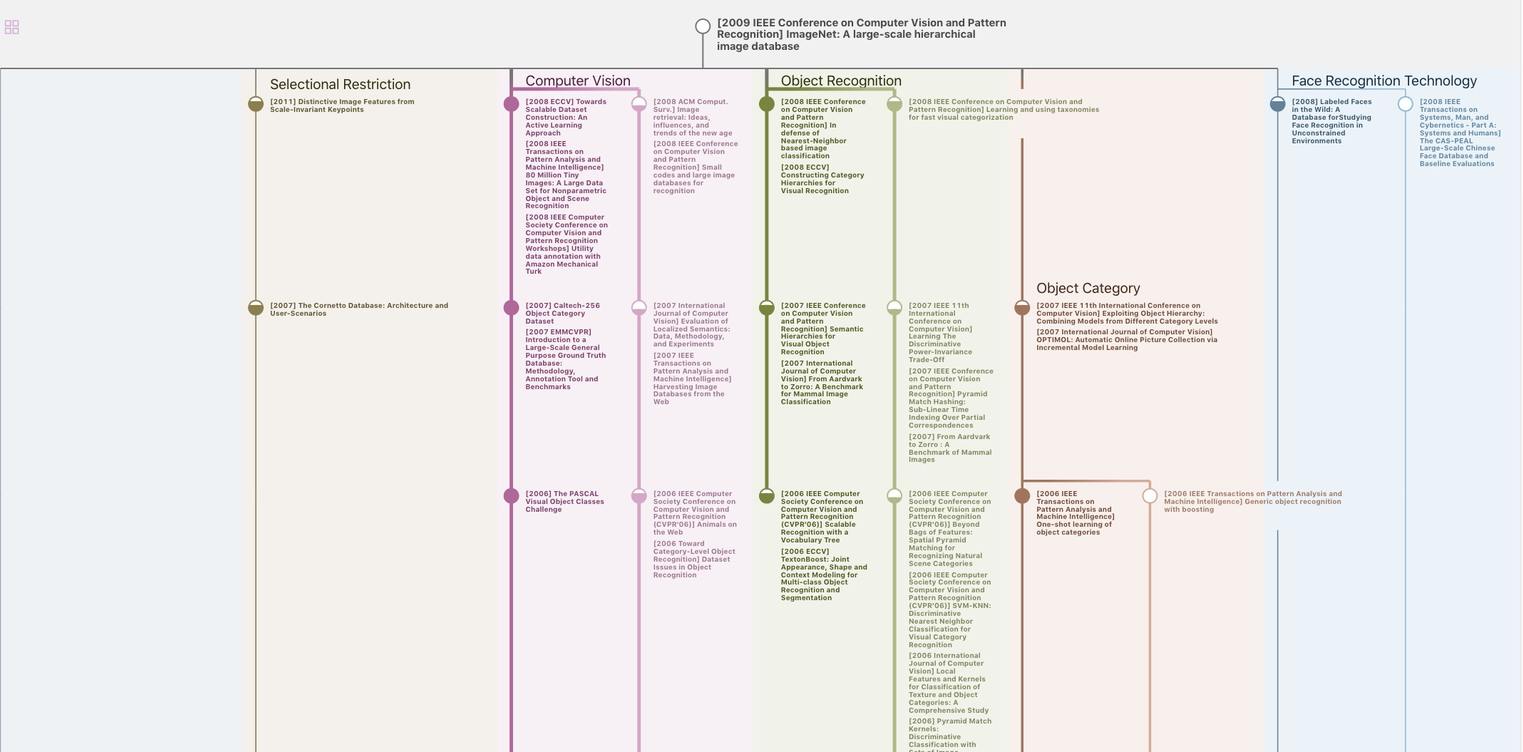
生成溯源树,研究论文发展脉络
Chat Paper
正在生成论文摘要