Reinforcement Learning based Multi-Attribute Slice Admission Control for Next-Generation Networks in a Dynamic Pricing Environment
2022 IEEE 95th Vehicular Technology Conference: (VTC2022-Spring)(2022)
摘要
Next-generation networks will provide intelligent infrastructure and management using machine learning. In real-world applications, demand for resources and performance within a service class may vary over time. Infrastructure providers choose which requests to accept with the goal of long-term profit maximization - a process known as slice admission control. In this paper, we envision a dynamic system with varying service requests attributes in urgency, duration, and amount of resources (i.e., computing, network, and storage). Further, we develop a dynamic pricing model that is responsive to demand and supply resulting in demand and supply reserve shaping. Then, we propose a solution to the slice admission control problem by using a reinforcement learning approach with Deep-Q Networks, where the state of the system is modeled using an array of parameters, similar to the input matrix in computer vision. Results show that our computer vision-inspired approach is capable of learning how the better policy to navigate this complex environment by selecting service requests that maximize the provider's long-term profit.
更多查看译文
关键词
Network slicing,reinforcement learning,slice admission control,pricing,NextG
AI 理解论文
溯源树
样例
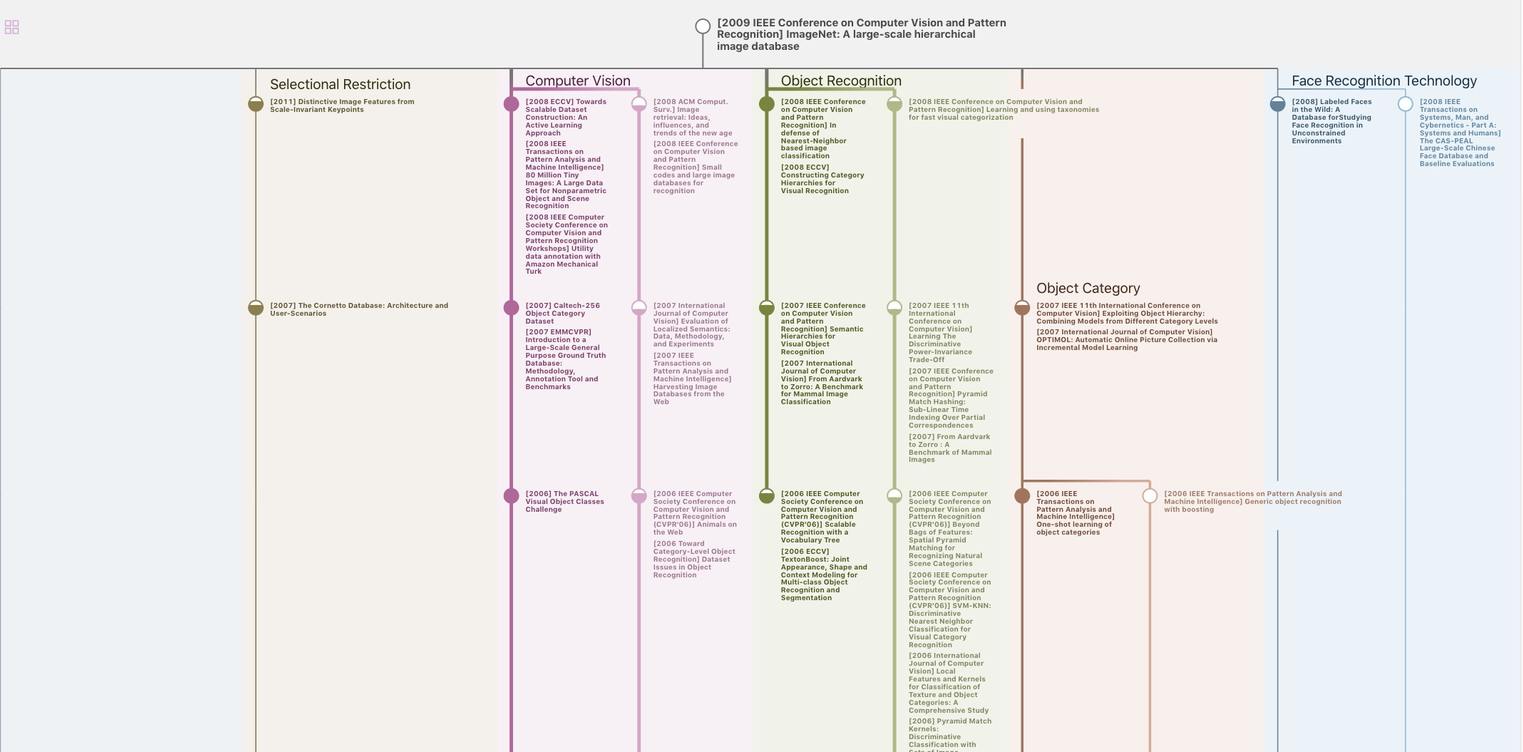
生成溯源树,研究论文发展脉络
Chat Paper
正在生成论文摘要