Beam Prediction for mmWave Massive MIMO using Adjustable Feature Fusion Learning
2022 IEEE 95th Vehicular Technology Conference: (VTC2022-Spring)(2022)
摘要
Beam training is one of the kernel problems in Millimeter-Wave(mmWave) massive multiple-input multiple-output(MIMO) systems. The beam direction explicitly relies on user location and is implicitly related to channel state information(CSI). Based on this fact, we propose a deep neural network-based novel downlink beam prediction framework to reduce the beam training overhead while achieving higher reliability. Considering that the user location and CSI are two completely different types and dimensions of information, the proposed neural network adopts adjustable feature fusion learning(AFFL) to fuse the two kinds of information. To reduce the beam training overhead, only the user location and the CSI of a minimal number of antennas are taken as the network’s inputs. In addition, when fusing, the signal-to-noise ratio(SNR) is used to adaptively adjust the weights of the two inputs on beam prediction output. Finally, simulation results corroborate that the proposed AFFL-based framework can achieve superior performance and robustness than the strategy which solely uses CSI, especially under low SNR conditions.
更多查看译文
关键词
mmwave massive mimo,adjustable feature fusion learning,feature fusion,beam,prediction
AI 理解论文
溯源树
样例
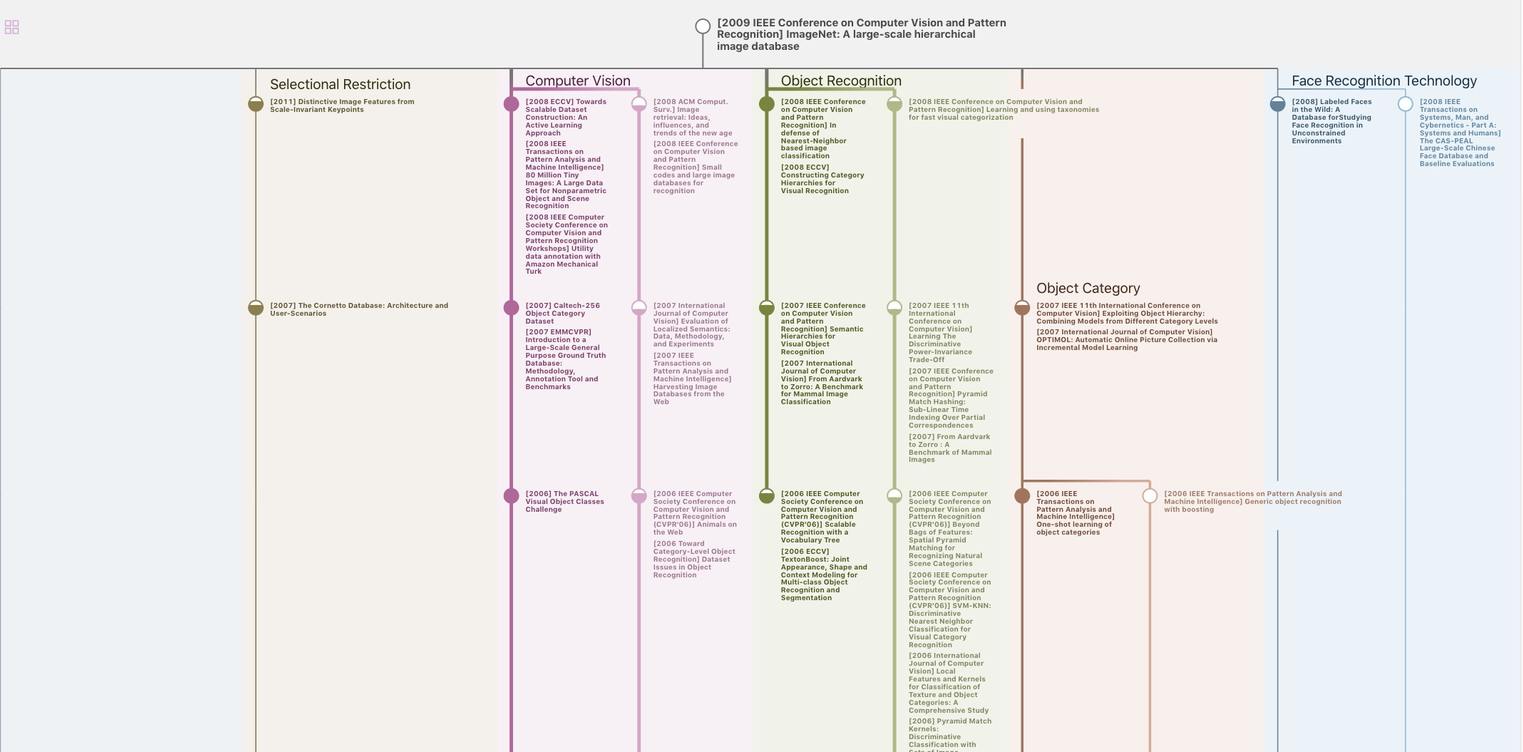
生成溯源树,研究论文发展脉络
Chat Paper
正在生成论文摘要