ID-NET: Inception deconvolutional neural network for multi-class classification in retinal fundus image
Journal of Mechanics in Medicine and Biology(2022)
摘要
Micro-aneurysms, hemorrhages, and hard exudates are the early lesions of diabetic retinopathy, with micro-aneurysms and small hemorrhages appearing as small and multi-size objects in the retinal fundus image. Though it is essentially a promising technique for object classification in images, the deep learning method needs specific investigation for dealing with retinal fundus images. To this, we propose an inception deconvolutional network, dubbed ID-Net, for multi-class object classification. The ID-Net consists of two inception modules, one with a series of convolutional filters used for extracting multi-scale features and the other with a stack of deconvolutional filters for recovering the features and thus increasing the feature representation capability. As a result, the proposed network succeeds in overcoming the under-fitting and representational bottlenecks, two challenges caused by imbalanced data of retinal fundus images. Experimental results show that the ID-Net can accurately classify early lesions, and achieves higher sensitivity and accuracy than the state-of-the-art evaluation on the public database.
更多查看译文
关键词
Inception module,deconvolution,feature sparsity,multi-size objects,retinal fundus images
AI 理解论文
溯源树
样例
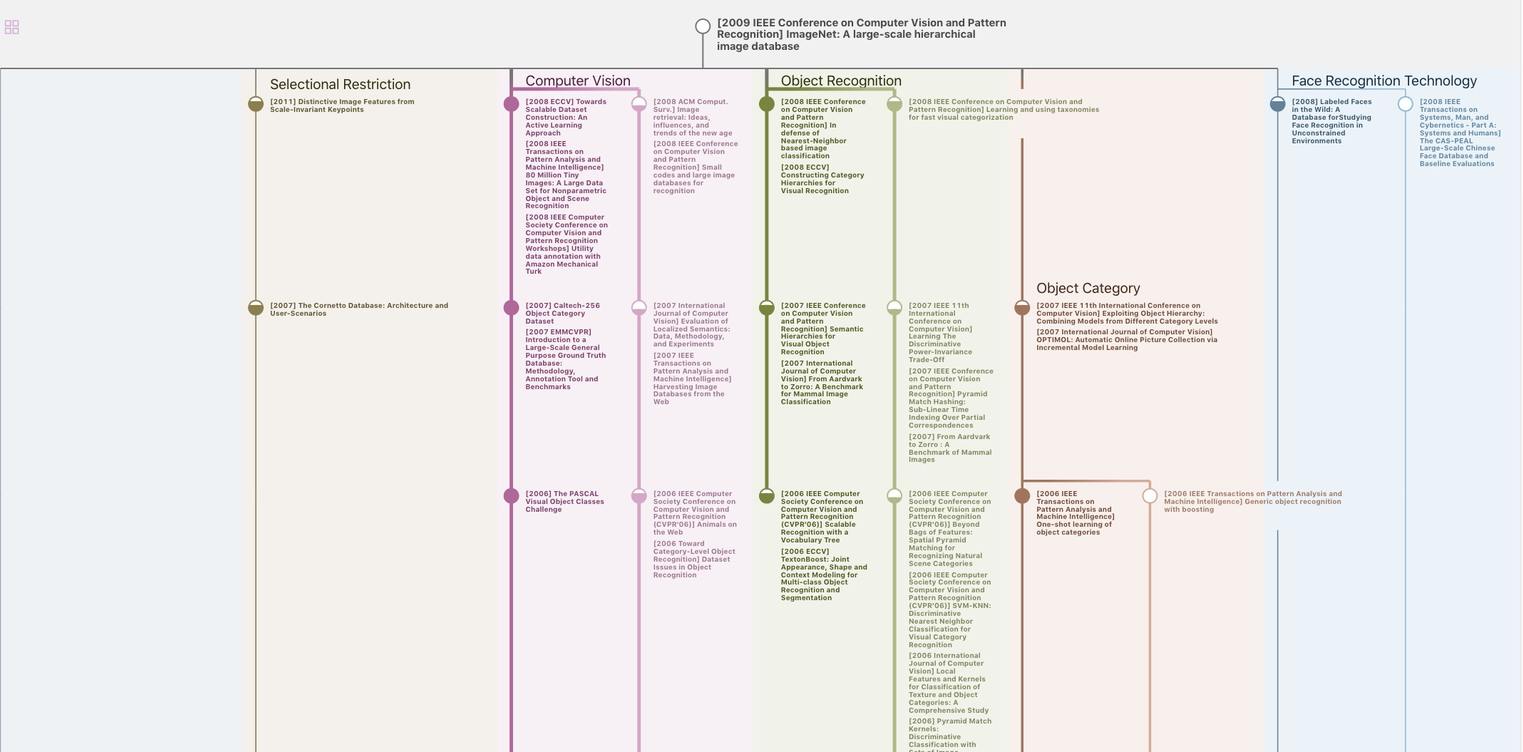
生成溯源树,研究论文发展脉络
Chat Paper
正在生成论文摘要