RNAGCN: RNA tertiary structure assessment with a graph convolutional network
Chinese Physics B(2022)
摘要
Abstract RNAs play crucial and versatile roles in cellular biochemical reactions. Since experimental approaches of determining their three-dimensional (3D) structures are costly and less efficient, it is greatly advantageous to develop computational methods to predict RNA 3D structures. For these methods, designing a model or scoring function for structure quality assessment is an essential step but this step poses challenges. In this study, we designed and trained a deep learning model to tackle this problem. The model was based on a Graph Convolutional Network (GCN) and named RNAGCN. The model provided a natural way of representing RNA structures, avoided complex algorithms to preserve atomic rotational equivalence, and was capable of extracting features automatically out of structural patterns. Testing results on two datasets convincingly demonstrated that RNAGCN performs similarly to or better than four leading scoring functions. Our approach provides an alternative way of RNA tertiary structure assessment and may facilitate RNA structure predictions. RNAGCN can be downloaded from https://gitee.com/dcw-RNAGCN/rnagcn.
更多查看译文
关键词
RNA structure predictions,scoring function,graph convolutional network,deep learning,RNA-puzzles
AI 理解论文
溯源树
样例
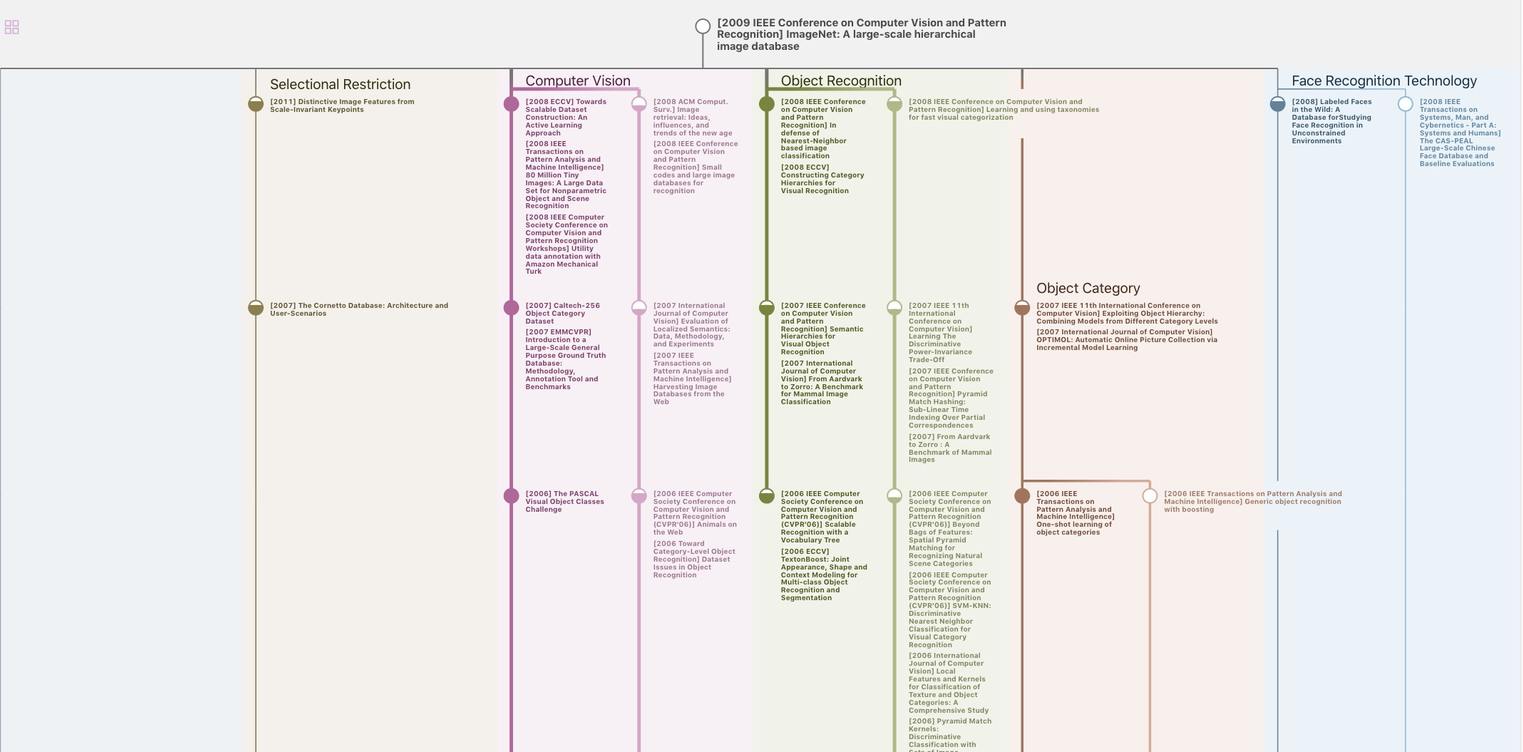
生成溯源树,研究论文发展脉络
Chat Paper
正在生成论文摘要