Machine learning-based radiomics for multiple primary prostate cancer biological characteristics prediction with 18 F-PSMA-1007 PET: comparison among different volume segmentation thresholds
La radiologia medica(2022)
摘要
Background PET-based radiomics features could predict the biological characteristics of primary prostate cancer (PCa). However, the optimal thresholds to predict the biological characteristics of PCa are unknown. This study aimed to compare the predictive power of 18 F-PSMA-1007 PET radiomics features at different thresholds for predicting multiple biological characteristics. Methods One hundred and seventy-three PCa patients with complete preoperative 18 F-PSMA-1007 PET examination and clinical data before surgery were collected. The prostate lesions' volumes of interest were semi-automatically sketched with thresholds of 30%, 40%, 50%, and 60% maximum standardized uptake value (SUVmax). The radiomics features were respectively extracted. The prediction models of Gleason score (GS), extracapsular extension (ECE), and vascular invasion (VI) were established using the support vector machine. The performance of models from different thresholding regions was assessed using receiver operating characteristic curve and confusion matrix-derived indexes. Results For predicting GS, the 50% SUVmax model showed the best predictive performance in training (AUC, 0.82 [95%CI 0.74–0.88]) and testing cohorts (AUC, 0.80 [95%CI 0.66–0.90]). For predicting ECE, the 40% SUVmax model exhibit the best predictive performance (AUC, 0.77 [95%CI 0.68–0.84] and 0.77 [95%CI 0.63–0.88]). As for VI, the 50% SUVmax model had the best predictive performance (AUC, 0.74 [95%CI 0.65–0.82] and 0.74 [95%CI 0.56–0.82]). Conclusion The 18 F-1007-PSMA PET-based radiomics features at 40–50% SUVmax showed the best predictive performance for multiple PCa biological characteristics evaluation. Compared to the single PSA model, radiomics features may provide additional benefits in predicting the biological characteristics of PCa.
更多查看译文
关键词
Prostate cancer,Radiomics,Machine learning,PET,PSMA-1007
AI 理解论文
溯源树
样例
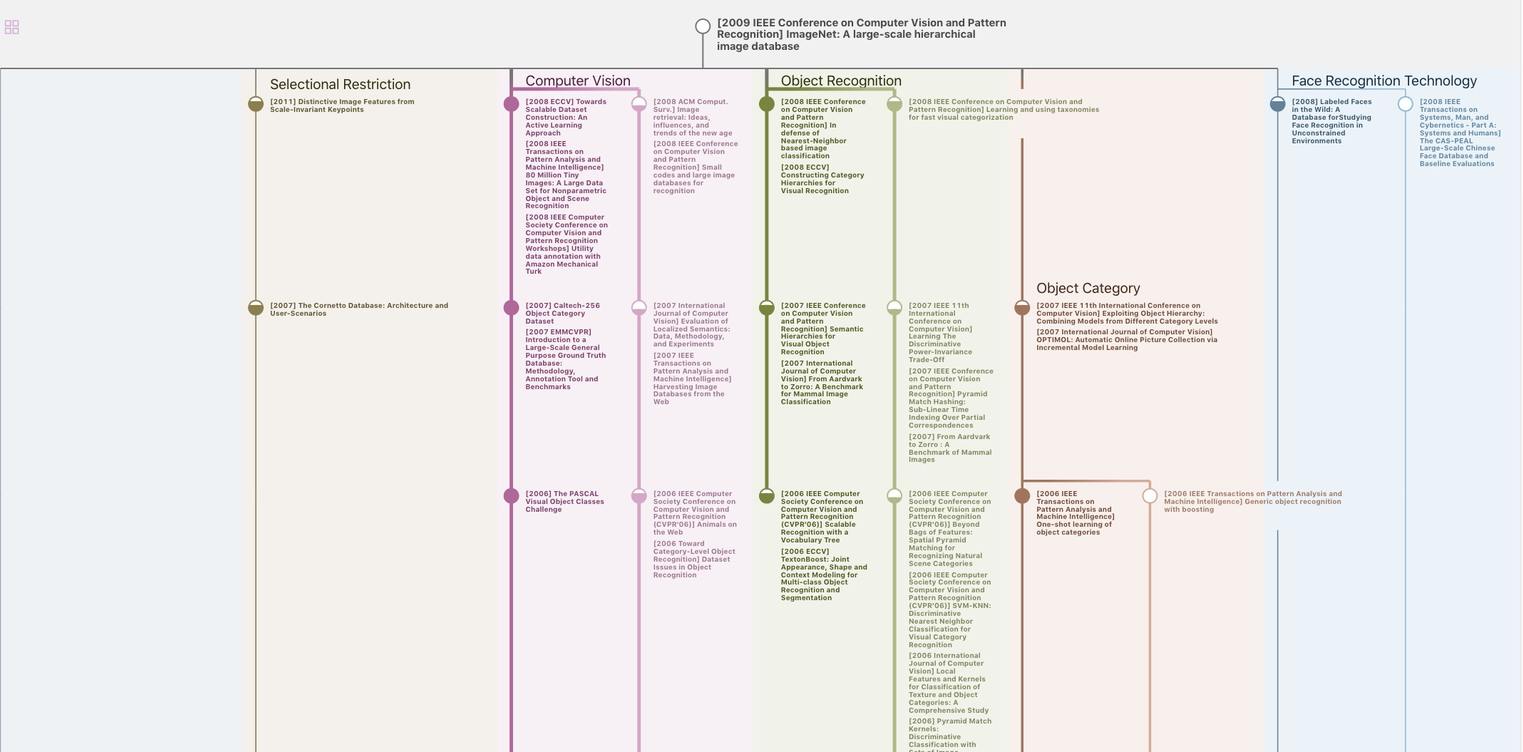
生成溯源树,研究论文发展脉络
Chat Paper
正在生成论文摘要