IoT-Cache: Caching Transient Data at the IoT Edge
2022 IEEE 47th Conference on Local Computer Networks (LCN)(2022)
摘要
Explosive traffic and service delay are bottlenecks in providing Quality of Service (QoS) to the Internet of Things (IoT) end-users. Edge caching emerged as a promising solution, but data transiency, limited caching capability, and network volatility trigger the dimensionality curse. Therefore, we propose a Deep Reinforcement Learning (DRL) approach, named IoT-Cache, to caching action optimization. An appropriate reward function is designed to increase the cache hit rate and optimize the overall data-cache allocation. A practical scenario with inconsistent requests and data item sizes is considered, and a Distributed Proximal Policy Optimization (DPPO) algorithm is proposed, enabling IoT edge nodes to learn caching policy. RLlib framework is used to scale the training in distributed Publish/Subscribe network. The performance evaluation demonstrates a significant improvement and faster convergence for IoT-Cache cost function, a trade-off between communication cost and data freshness over existing DRL and baseline caching solutions.
更多查看译文
关键词
Internet of Things,Edge Caching,Deep Reinforcement Learning,Distributed Proximal Policy Optimisation
AI 理解论文
溯源树
样例
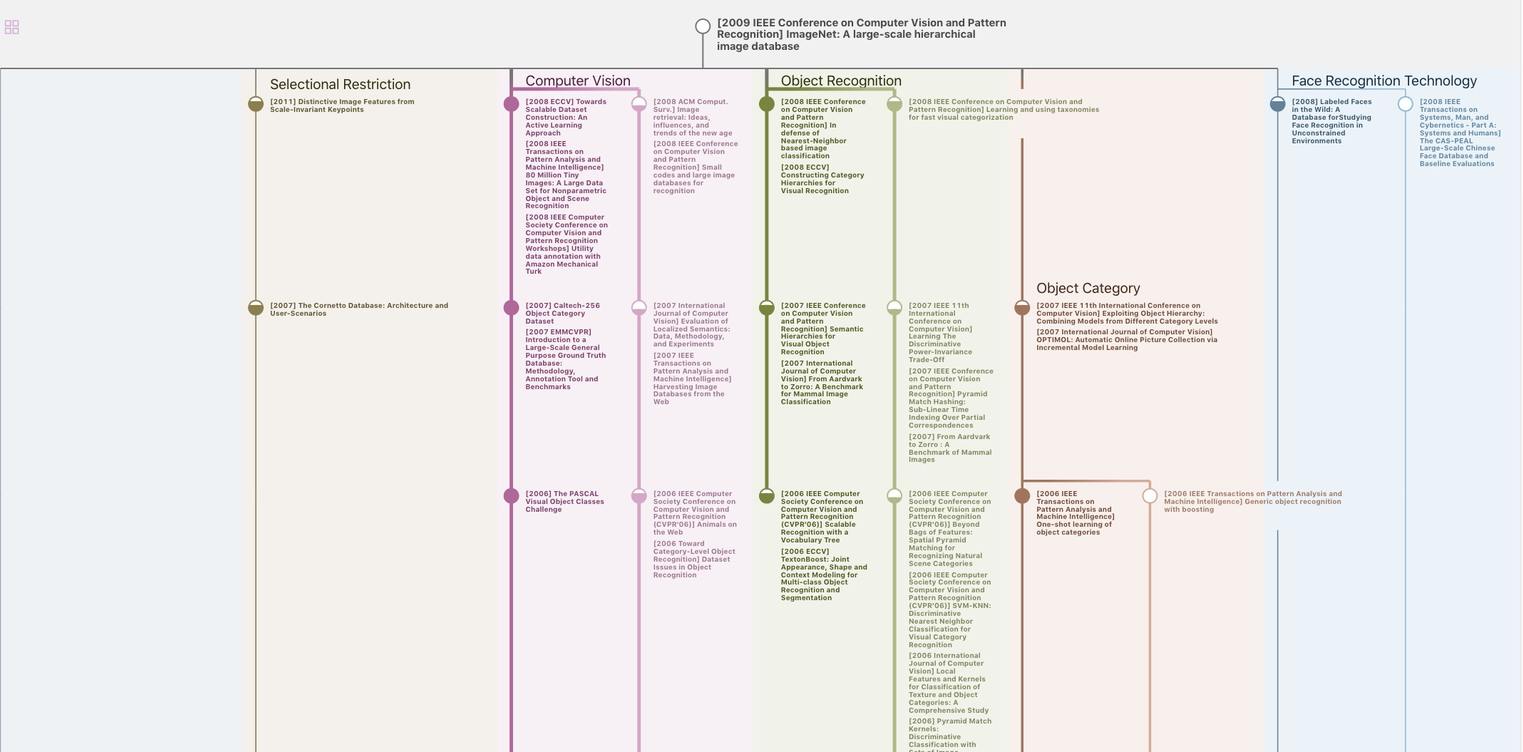
生成溯源树,研究论文发展脉络
Chat Paper
正在生成论文摘要