Semi-Supervised 3D Medical Image Segmentation Using Shape-Guided Dual Consistency Learning
2022 IEEE International Conference on Multimedia and Expo (ICME)(2022)
摘要
Popular semi-supervised image segmentation networks suf-fer from two problems: firstly, supervision is only performed on the last layer of the decoder, resulting in the network's weak generalization ability; secondly, the geometry shape constraints of targets are frequently disregarded in these net-works, leading to poor segmentation results. To address these issues, we propose a novel shape-guided dual consistency semi-supervised learning framework for 3D medical image segmentation. The proposed framework makes two contri-butions. Initially, we introduce a shape constraint to learn the shape representation, which converts the difference be-tween two networks into an unsupervised loss and lets the model learn the boundary information of targets. Addition-ally, we develop a deep-supervised knowledge transfer strat-egy that improves the generalization ability of the network without increasing extra computation costs. Experiments demonstrate that the proposed framework outperforms state-of-the-art semi-supervised methods due to the strong ability of knowledge mining on unlabeled data.
更多查看译文
关键词
3D medical image segmentation,semi-supervised learning,shape constraint,deep supervision
AI 理解论文
溯源树
样例
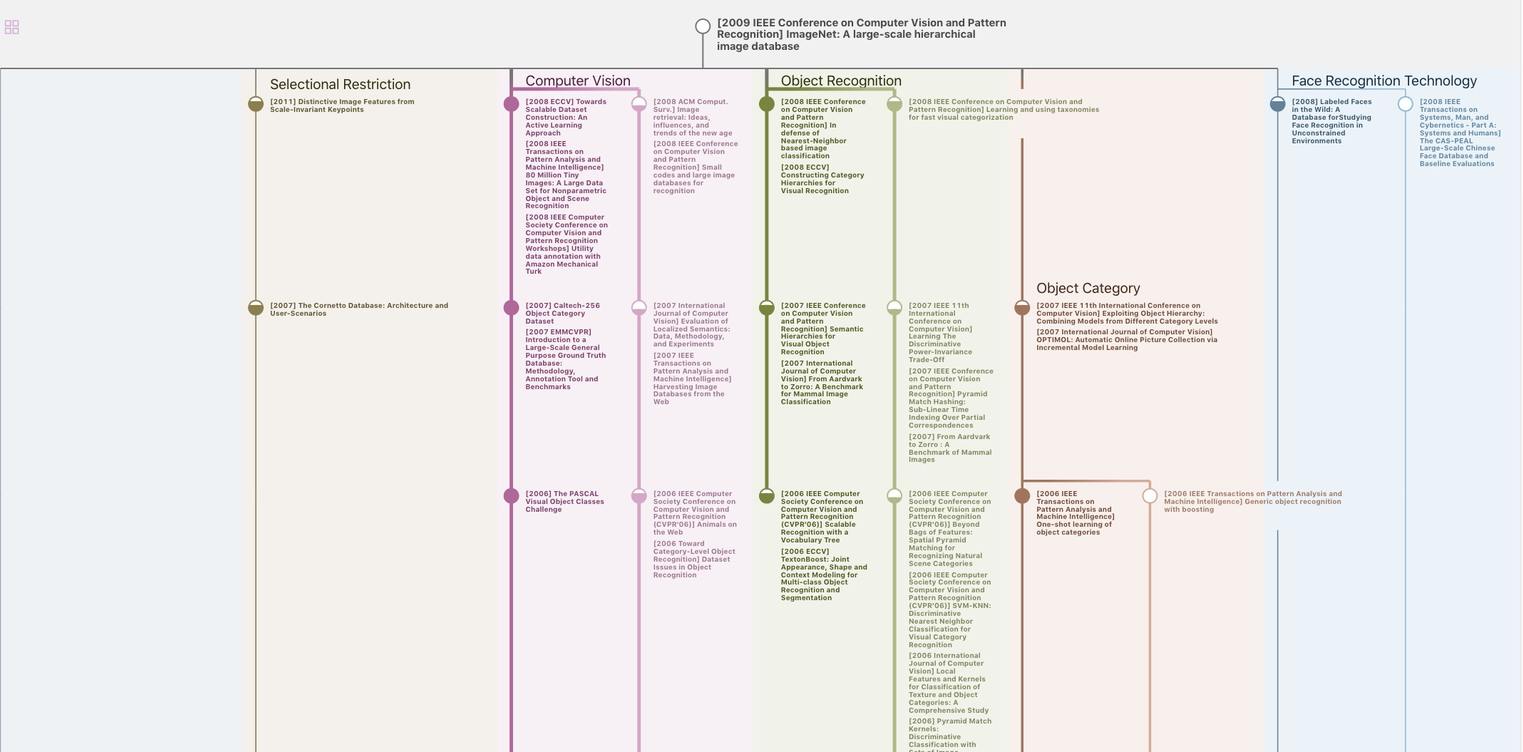
生成溯源树,研究论文发展脉络
Chat Paper
正在生成论文摘要