Learning energy-efficient driving behaviors by imitating experts
International Conference on Intelligent Transportation Systems (ITSC)(2022)
摘要
The rise of vehicle automation has generated significant interest in the potential role of future automated vehicles (AVs). In particular, in highly dense traffic settings, AVs are expected to serve as congestion-dampeners, mitigating the presence of instabilities that arise from various sources. However, in many applications, such maneuvers rely heavily on non-local sensing or coordination by interacting AVs, thereby rendering their adaptation to real-world settings a particularly difficult challenge. To address this challenge, this paper examines the role of imitation learning in bridging the gap between such control strategies and realistic limitations in communication and sensing. Treating one such controller as an "expert", we demonstrate that imitation learning can succeed in deriving policies that, if adopted by 5% of vehicles, may boost the energy-efficiency of networks with varying traffic conditions by 15% using only local observations. Results and code are available online at https://sites.google.com/ view/il- traffic/home.
更多查看译文
关键词
energy-efficient driving,vehicle automation,automated vehicles,congestion-dampeners,imitation learning,control strategies,realistic limitations,traffic conditions,local observations,interacting AV,dense traffic settings
AI 理解论文
溯源树
样例
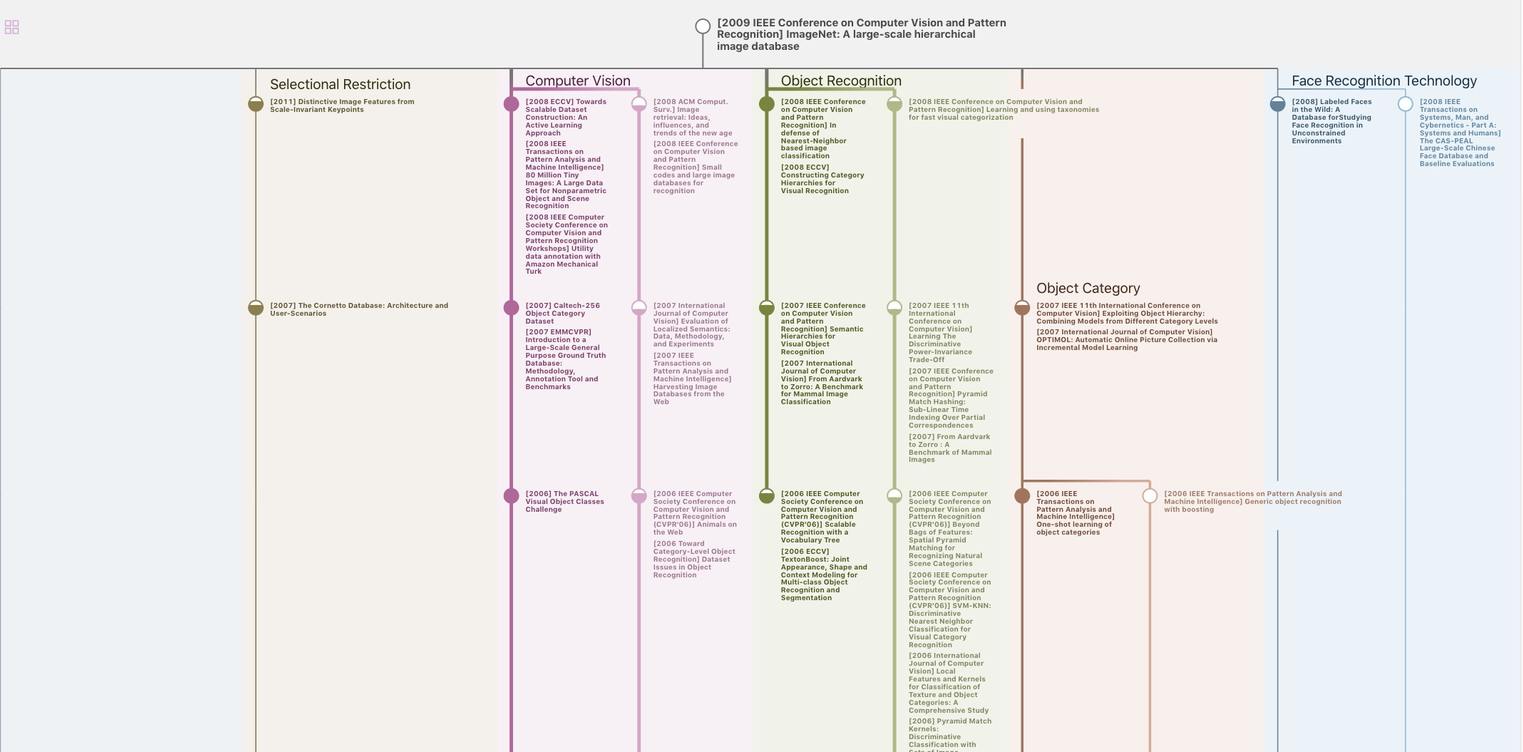
生成溯源树,研究论文发展脉络
Chat Paper
正在生成论文摘要