Training and Tuning Generative Neural Radiance Fields for Attribute-Conditional 3D-Aware Face Generation
arxiv(2022)
摘要
3D-aware GANs based on generative neural radiance fields (GNeRF) have achieved impressive high-quality image generation, while preserving strong 3D consistency. The most notable achievements are made in the face generation domain. However, most of these models focus on improving view consistency but neglect a disentanglement aspect, thus these models cannot provide high-quality semantic/attribute control over generation. To this end, we introduce a conditional GNeRF model that uses specific attribute labels as input in order to improve the controllabilities and disentangling abilities of 3D-aware generative models. We utilize the pre-trained 3D-aware model as the basis and integrate a dual-branches attribute-editing module (DAEM), that utilize attribute labels to provide control over generation. Moreover, we propose a TRIOT (TRaining as Init, and Optimizing for Tuning) method to optimize the latent vector to improve the precision of the attribute-editing further. Extensive experiments on the widely used FFHQ show that our model yields high-quality editing with better view consistency while preserving the non-target regions. The code is available at https://github.com/zhangqianhui/TT-GNeRF.
更多查看译文
AI 理解论文
溯源树
样例
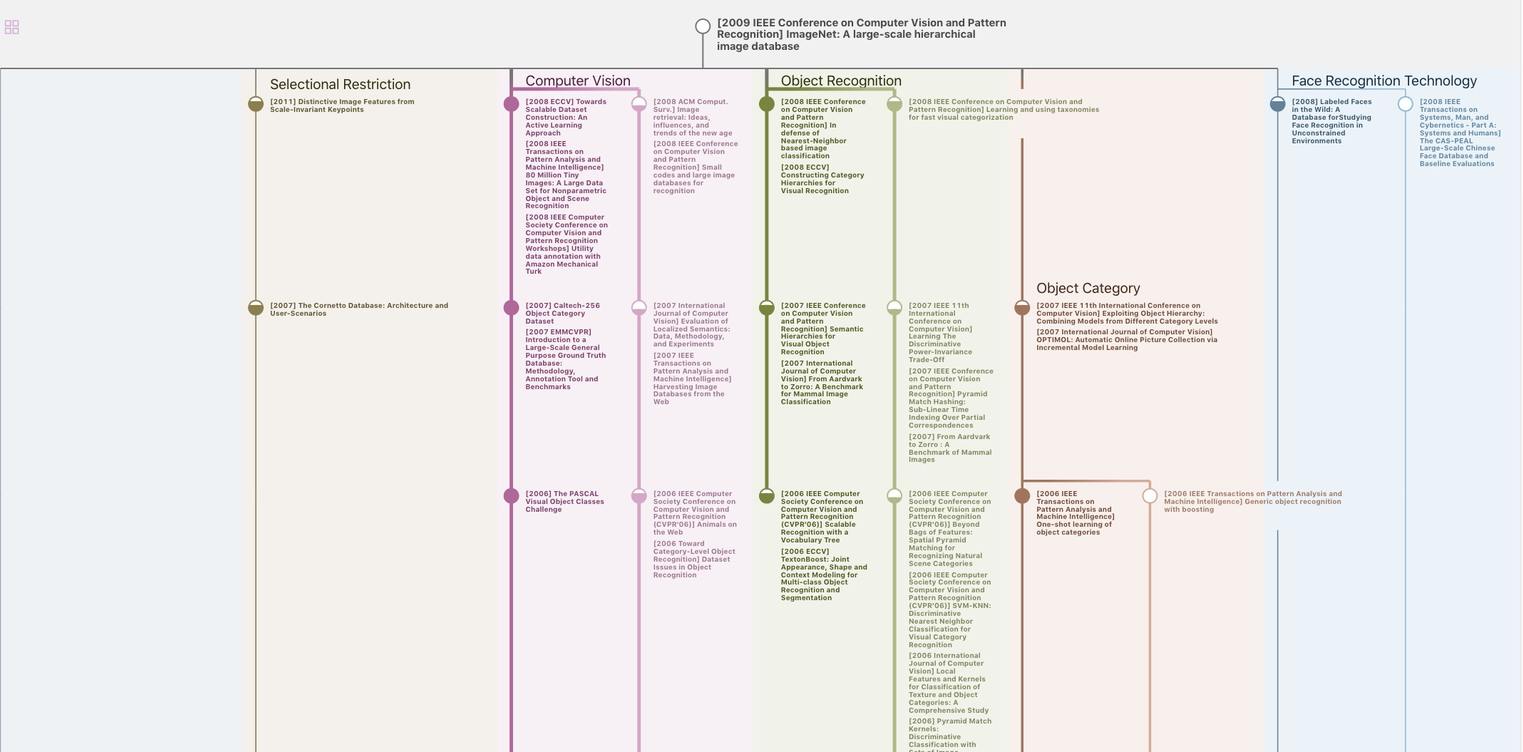
生成溯源树,研究论文发展脉络
Chat Paper
正在生成论文摘要