A comprehensive social matrix factorization for recommendations with prediction and feedback mechanisms by fusing trust relationships and social tags
Soft Computing(2022)
摘要
Social relationships play an important role in improving the quality of recommender systems (RSs). A large number of experimental results show that social relationship-based recommendation methods alleviate the problems of data sparseness and cold start in RSs to some extent. However, existing recommendation methods have difficulty in accurately obtaining user features and item features, which seriously affects recommendation system performance. To accurately model social relationships and improve recommendation quality, we use both explicit (e.g. user-item ratings, trust relationships) and implicit (e.g. social tags) social relationships to mine users’ potential interest preferences; thus, we propose a social recommendation method incorporating trust relationships and social tags. The method maps user features and item features to a shared feature space using the above social relationship, obtains user similarity and item similarity through potential feature vectors of users and items, and continuously trains them to obtain accurate similarity relationships to improve recommendation performance. The experimental results demonstrate that our proposed approach achieves superior performance over the other social recommendation approaches.
更多查看译文
关键词
Recommender systems,Collaborative filtering,Matrix factorization,Social relationships,Social networks
AI 理解论文
溯源树
样例
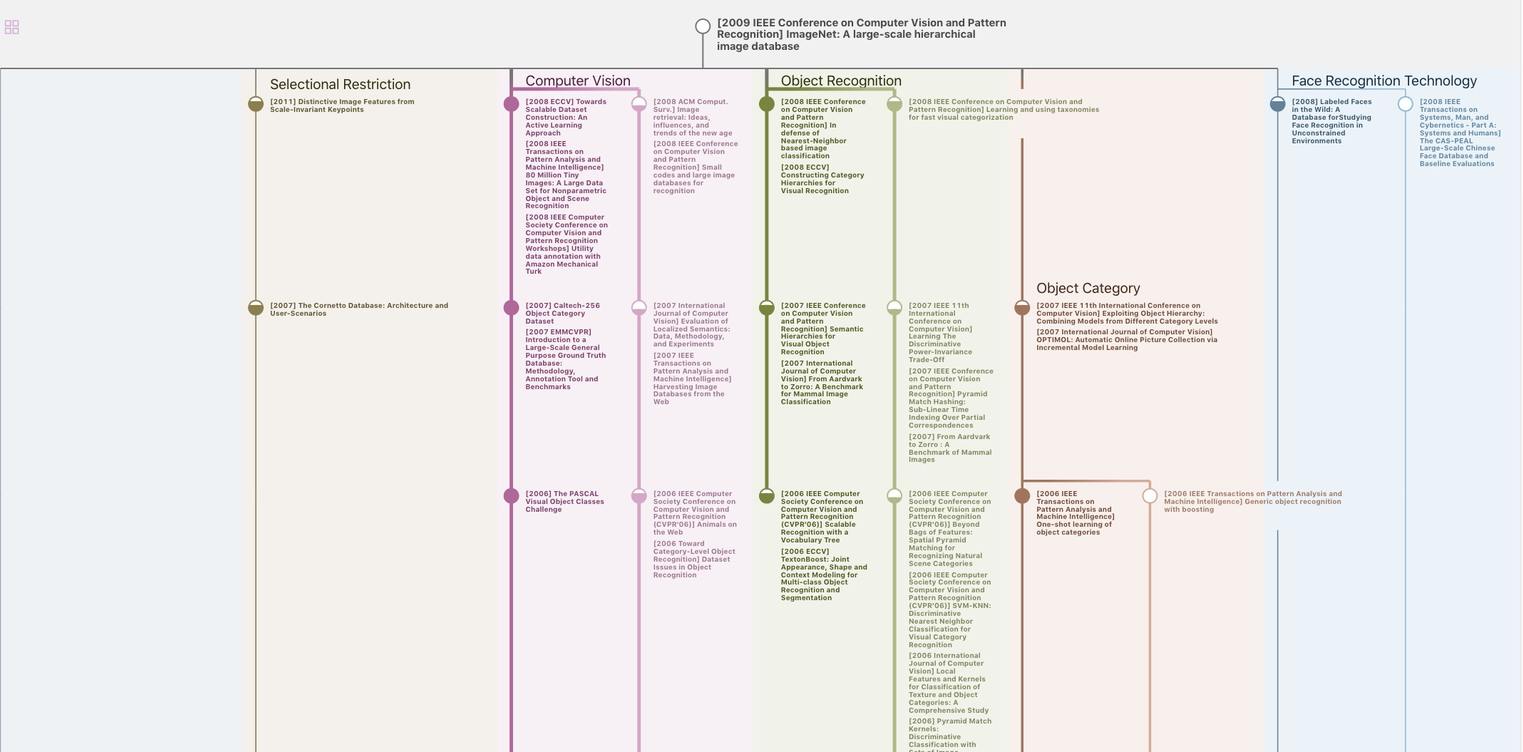
生成溯源树,研究论文发展脉络
Chat Paper
正在生成论文摘要