Optimal Model Averaging of Mixed-Data Kernel-Weighted Spline Regressions
Journal of Business & Economic Statistics(2022)
摘要
Model averaging has a rich history dating from its use for combining forecasts from time-series models (Bates and Granger) and presents a compelling alternative to model selection methods. We propose a frequentist model averaging procedure defined over categorical regression splines (Ma, Racine, and Yang) that allows for mixed-data predictors, as well as nonnested and heteroscedastic candidate models. We demonstrate the asymptotic optimality of the proposed model averaging estimator, and develop a post-averaging inference theory for it. Theoretical underpinnings are provided, finite-sample performance is evaluated, and an empirical illustration reveals that the method is capable of outperforming a range of popular model selection criteria in applied settings. An R package is available for practitioners (Racine).
更多查看译文
关键词
Categorical covariates,Cross-validation,Frequentist estimation,Nonparametric estimation
AI 理解论文
溯源树
样例
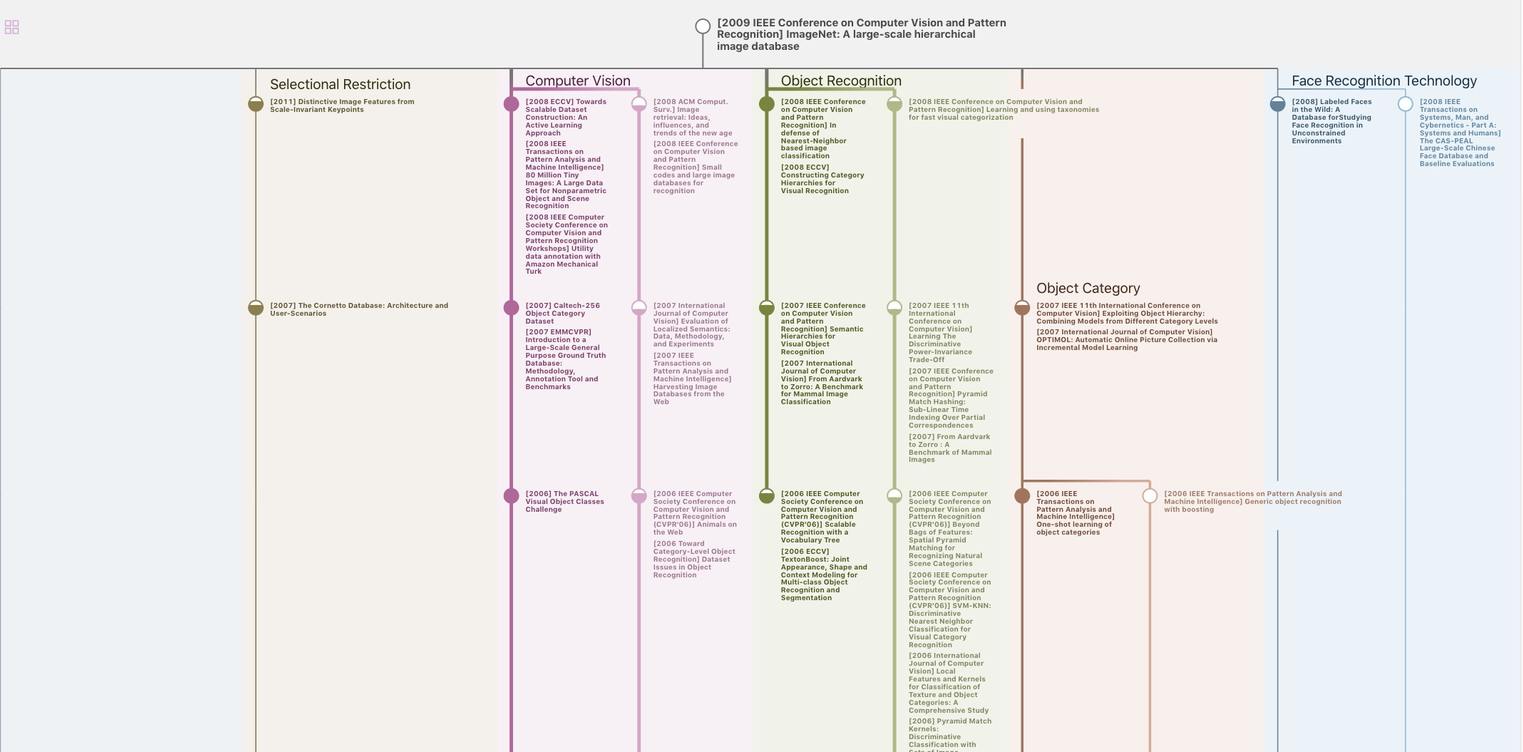
生成溯源树,研究论文发展脉络
Chat Paper
正在生成论文摘要