Accelerating Tensor Contraction Products Via Tensor-Train Decomposition [tips & Tricks].
IEEE signal processing magazine(2022)
摘要
Tensors (multiway arrays) and tensor decompositions (TDs) have recently received tremendous attention in the data analytics community, due to their ability to mitigate the curse of dimensionality associated with modern large-dimensional big data [1] , [2] . Indeed, TDs allow for data volume (e.g., the parameter complexity) to be reduced from scaling exponentially to scaling linearly in the tensor dimensions, which facilitates applications in areas including the compression and interpretability of neural networks [1] , [3] , multimodal learning [1] , and completion of knowledge graphs [4] , [5] . At the heart of TD techniques is the tensor contraction product (TCP), an operator used for representing even the most unmanageable higher-order tensors through a set of small-scale core tensors that are interconnected via TCP operations [2] .
更多查看译文
关键词
Complexity theory,Tensors,Data analysis,Software libraries,Neural networks,Market research,Mathematical models,Big Data,Machine learning,Notch filters,Computational efficiency,Approximation error,Signal processing algorithms
AI 理解论文
溯源树
样例
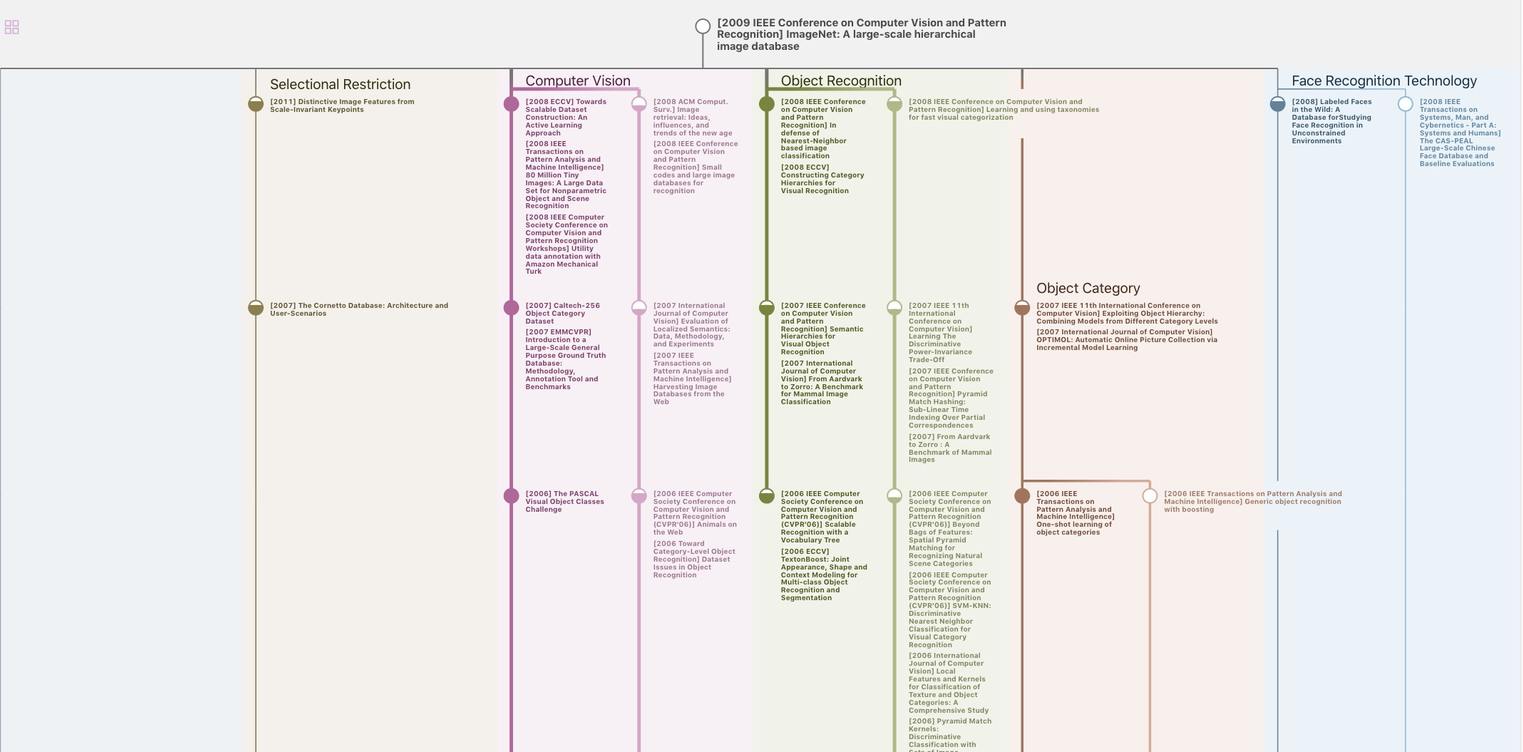
生成溯源树,研究论文发展脉络
Chat Paper
正在生成论文摘要