PECAN: A Product-Quantized Content Addressable Memory Network
2023 DESIGN, AUTOMATION & TEST IN EUROPE CONFERENCE & EXHIBITION, DATE(2023)
摘要
A novel deep neural network (DNN) architecture is proposed wherein the filtering and linear transform are realized solely with product quantization (PQ). This results in a natural implementation via content addressable memory (CAM), which transcends regular DNN layer operations and requires only simple table lookup. Two schemes are developed for the end-to-end PQ prototype training, namely, through angle- and distance-based similarities, which differ in their multiplicative and additive natures with different complexity-accuracy tradeoffs. Even more, the distance-based scheme constitutes a truly multiplier-free DNN solution. Experiments confirm the feasibility of such Product-QuantizEd Content Addressable Memory Network (PECAN), which has strong implication on hardware-efficient deployments especially for in-memory computing.
更多查看译文
关键词
product quantization,DNN compression,in-memory computing
AI 理解论文
溯源树
样例
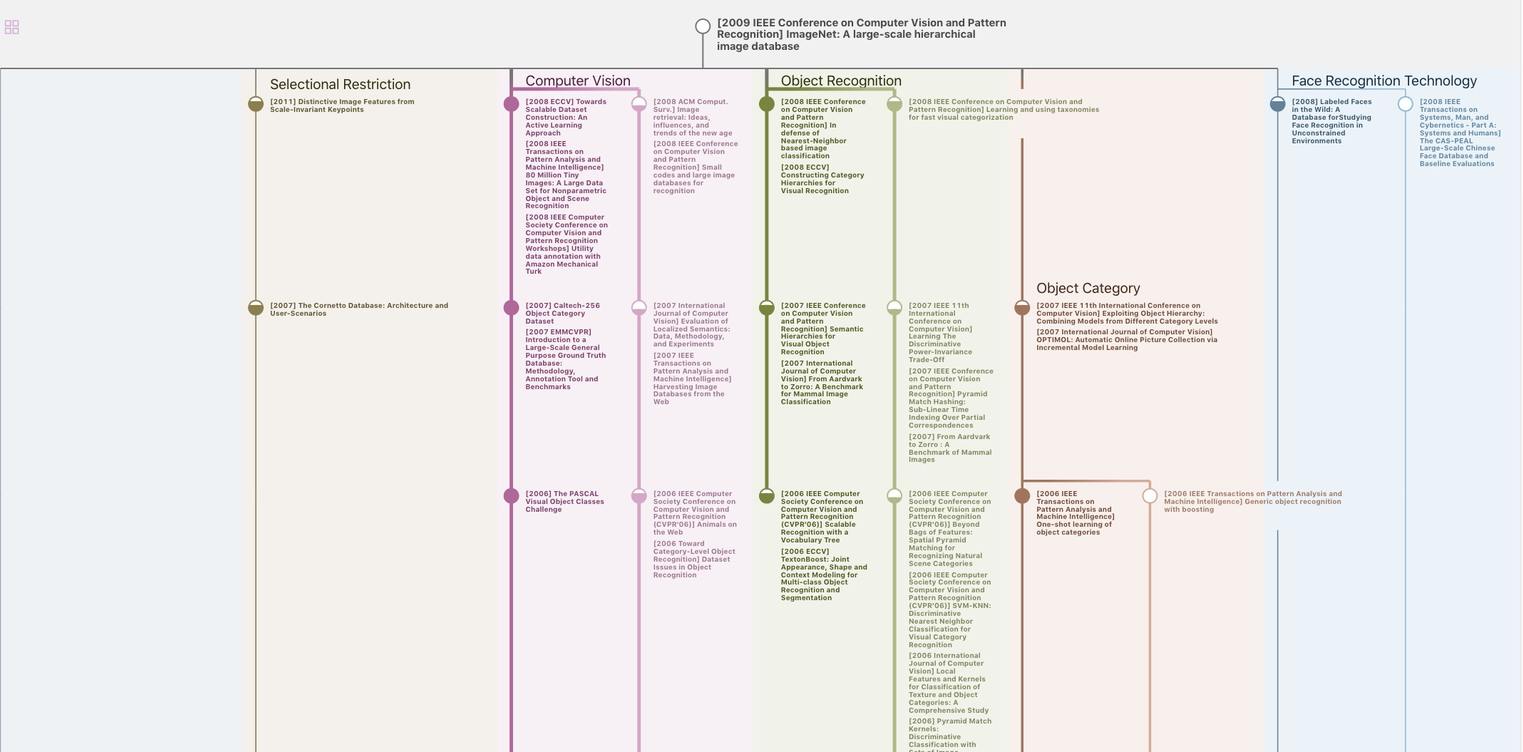
生成溯源树,研究论文发展脉络
Chat Paper
正在生成论文摘要