Dynamic Data-Free Knowledge Distillation by Easy-to-Hard Learning Strategy
arxiv(2023)
摘要
Data-free knowledge distillation (DFKD) aims at training lightweight student networks from large pretrained teacher networks without training data. Existing approaches follow the paradigm of generating informative samples and updating student models by targeting data priors, boundary samples, or memory samples. However, they don't dynamically adjust the generation strategy at different training stages, which in turn makes it DFKD difficult to achieve efficient and stable training. In this paper, we explore how to teach students the model from a dynamic perspective and propose a new approach, namely "CuDFKD", i.e., "\textbf{D}ata-\textbf{F}ree \textbf{K}nowledge \textbf{D}istillation with \textbf{Cu}rriculum". It dynamically learns from easy samples to difficult samples, which is similar to the human learning. In addition, we provide a theoretical analysis of the majorization minimization (MM) algorithm and explain the convergence of CuDFKD. Experiments conducted on benchmark datasets show that with a simple course design strategy, CuDFKD achieves the best performance over state-of-the-art DFKD methods and different benchmarks, even better than training from scratch with data. The training is fast, reaching the highest accuracy of 90\% within 15 epochs when distilling ResNet34 to ResNet18 in CIFAR10. Besides, the applicability of CuDFKD is also analyzed and discussed.
更多查看译文
关键词
Data-free knowledge distillation,Curriculum learning,Knowledge distillation,Self-paced learning
AI 理解论文
溯源树
样例
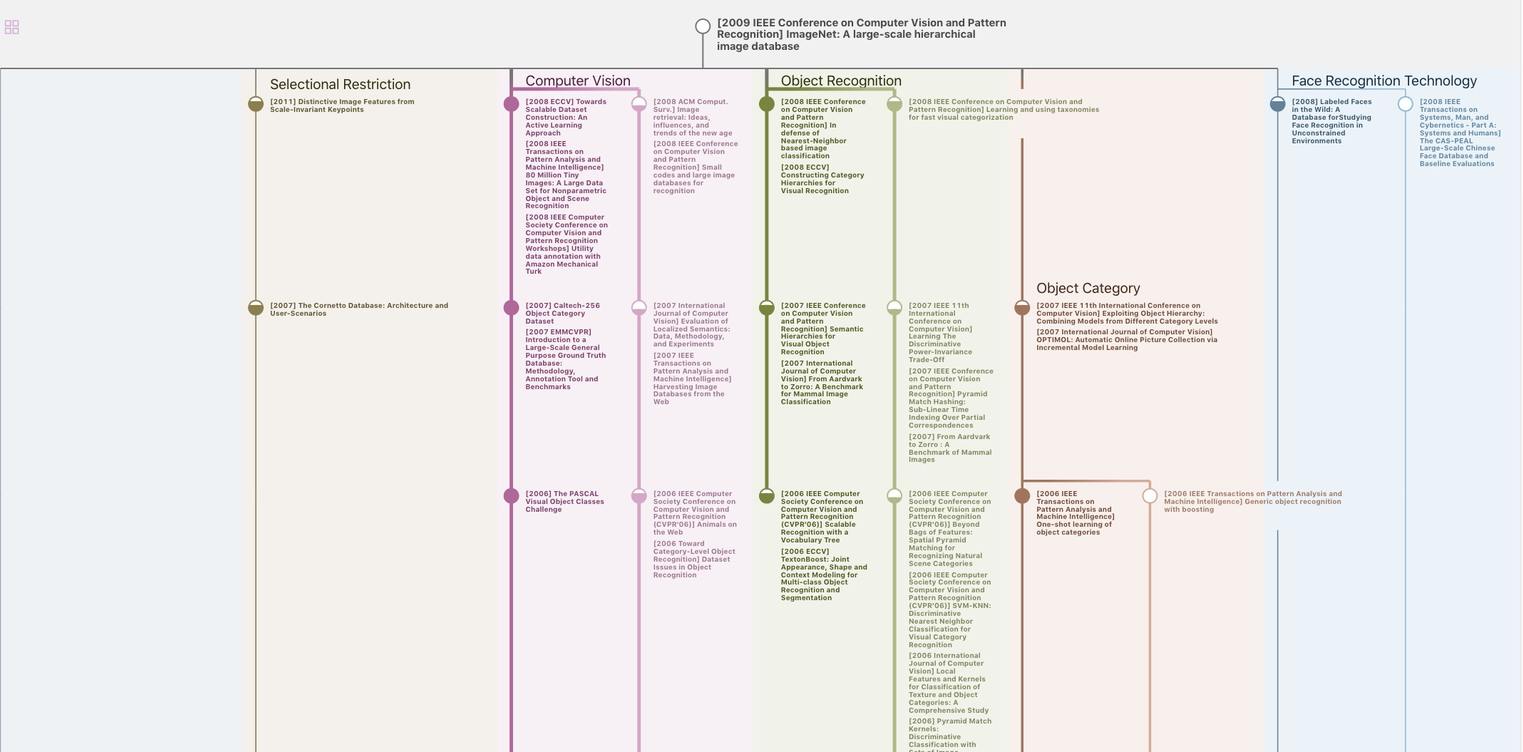
生成溯源树,研究论文发展脉络
Chat Paper
正在生成论文摘要