DeepQueueNet: towards scalable and generalized network performance estimation with packet-level visibility
SIGCOMM '22: Proceedings of the ACM SIGCOMM 2022 Conference(2022)
摘要
Network simulators are an essential tool for network operators, and can assist important tasks such as capacity planning, topology design, and parameter tuning. Popular simulators are all based on discrete event simulation, and their performance does not scale with the size of modern networks. Recently, deep-learning-based techniques are introduced to solve the scalability problem, but, as we show with experiments, they have poor visibility in their simulation results, and cannot generalize to diverse scenarios. In this work, we combine scalable and generalized continuous simulation techniques with discrete event simulation to achieve high scalability, while providing packet-level visibility. We start from a solid queueing-theoretic modeling of modern networks, and carefully identify the mathematically-intractable or computationally-expensive parts, only which are then modeled using deep neural networks (DNN). Dubbed DeepQueueNet , our approach combines prior knowledge of networks, and supports arbitrary topology and device traffic management mechanisms (given sufficient training data). Our extensive experiments show that DeepQueueNet achieves near-linear speedup in the number of GPUs, and its estimation accuracy for average and 99th percentile round-trip time outperforms existing end-to-end DNN-based performance estimators in all scenarios.
更多查看译文
关键词
Network simulation, Network performance estimation, Machine learning, Queueing theory, Network modeling
AI 理解论文
溯源树
样例
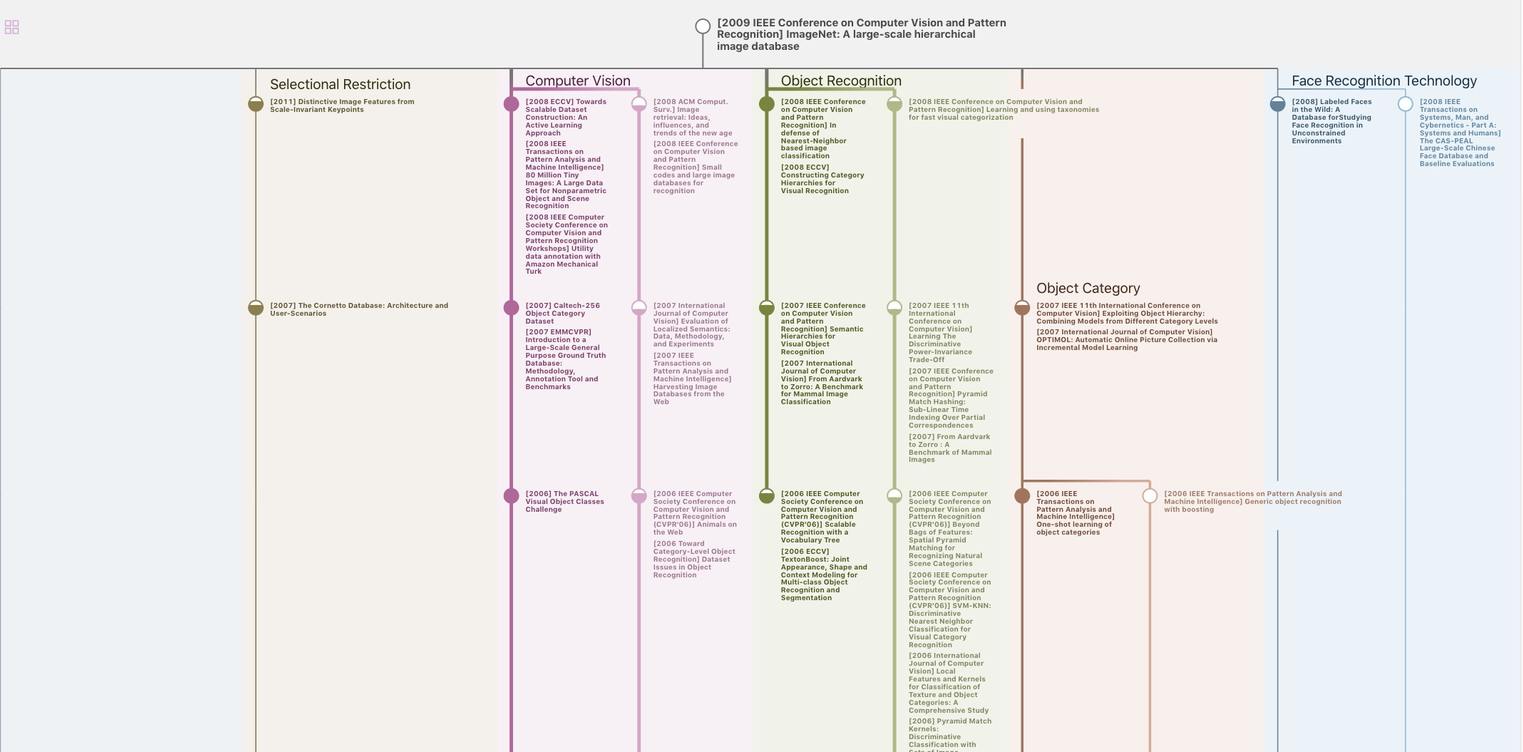
生成溯源树,研究论文发展脉络
Chat Paper
正在生成论文摘要