Toward selecting optimal predictive multiscale models
Computer Methods in Applied Mechanics and Engineering(2022)
摘要
This work presents a systematic strategy for selecting an "optimal" predictive computational model among a set of possible mechanistic models of a physical system. To this end, the Occam-Plausibility Algorithm (Farrell-Maupin et al., 2015) is extended by introducing a method for the design of model-specific validation experiments to provide data that portray the features of the prediction quantities of interest. Leveraging Bayesian inference and model plausibility, the framework adaptively balances the trade-off between complexity and validity of the models while taking into account the uncertainty in data, model parameters, and the choice of the model itself for making reliable computational predictions. An application of this framework is demonstrated in discrete-continuum multiscale modeling of size-dependent plasticity in polycrystalline materials, involving dislocation dynamics simulations and strain gradient plasticity models.This study suggests that the effectiveness of validation experiments relies upon the choice of the model; thus, a validation data set that adequately informs a predictive computational model may not be effective for validating other models. Additionally, the multiscale modeling results show that, given the discrete dislocation dynamics data, the optimal strain gradient plasticity model for predicting the deformation of a microelectromechanical system is obtained by excluding the isotropic hardening mechanism.(c) 2022 Elsevier B.V. All rights reserved.
更多查看译文
关键词
Bayesian inference, Model selection, Uncertainty quantification, Discrete dislocation dynamics, Strain gradient plasticity
AI 理解论文
溯源树
样例
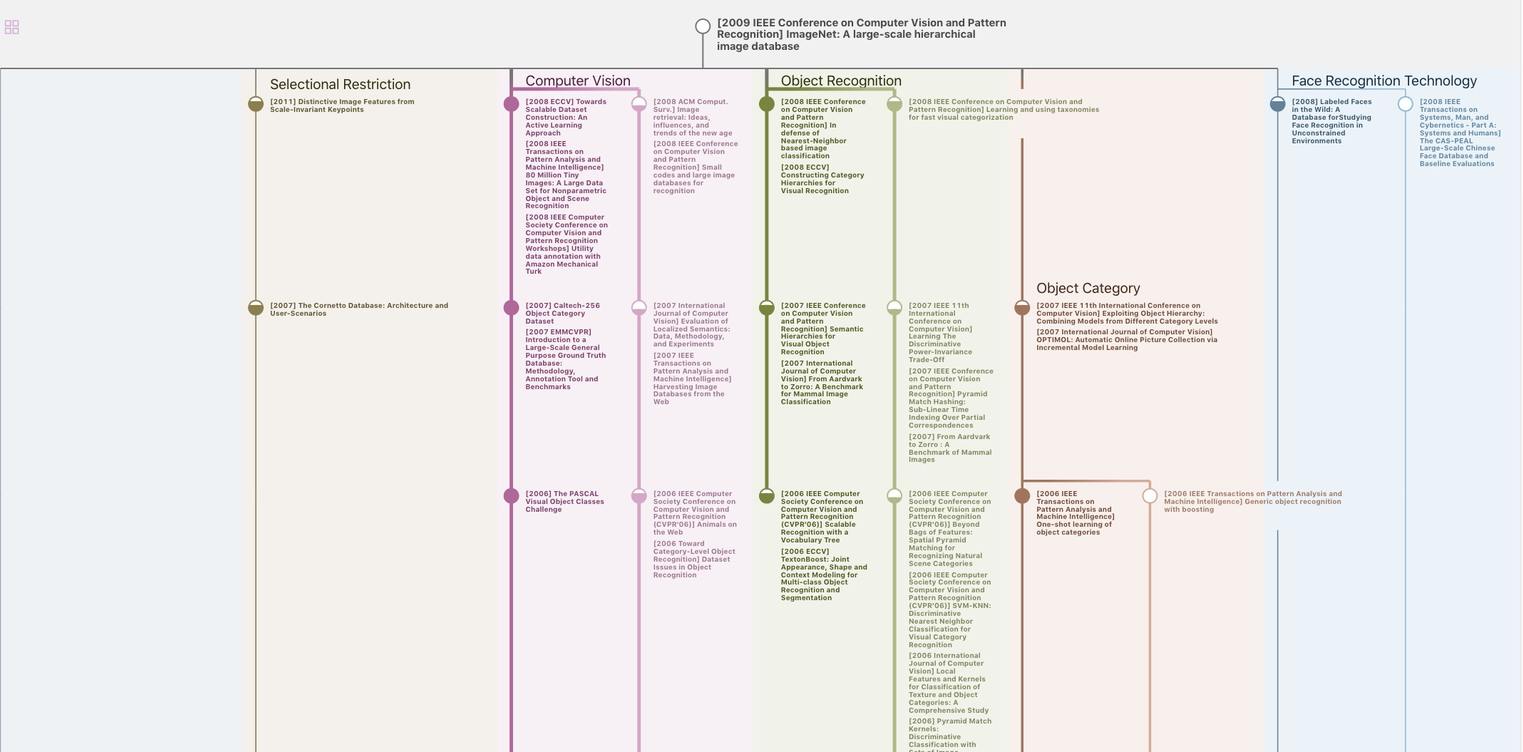
生成溯源树,研究论文发展脉络
Chat Paper
正在生成论文摘要