Associative Learning for Network Embedding
arxiv(2022)
摘要
The network embedding task is to represent the node in the network as a low-dimensional vector while incorporating the topological and structural information. Most existing approaches solve this problem by factorizing a proximity matrix, either directly or implicitly. In this work, we introduce a network embedding method from a new perspective, which leverages Modern Hopfield Networks (MHN) for associative learning. Our network learns associations between the content of each node and that node's neighbors. These associations serve as memories in the MHN. The recurrent dynamics of the network make it possible to recover the masked node, given that node's neighbors. Our proposed method is evaluated on different downstream tasks such as node classification and linkage prediction. The results show competitive performance compared to the common matrix factorization techniques and deep learning based methods.
更多查看译文
关键词
network,learning
AI 理解论文
溯源树
样例
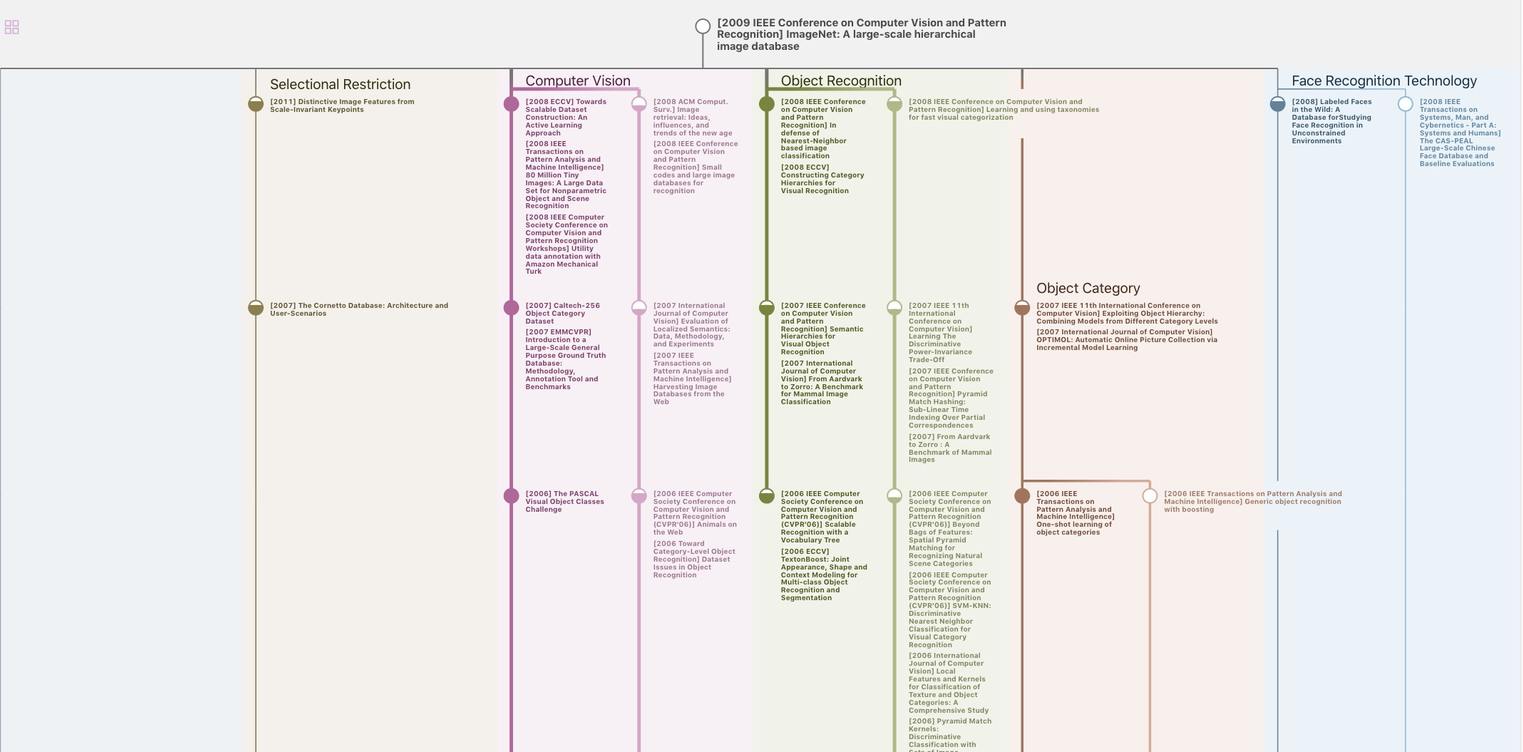
生成溯源树,研究论文发展脉络
Chat Paper
正在生成论文摘要