Precipitation Retrieval From Fengyun-3D MWHTS and MWRI Data Using Deep Learning
IEEE JOURNAL OF SELECTED TOPICS IN APPLIED EARTH OBSERVATIONS AND REMOTE SENSING(2022)
摘要
In this article, two multitask deep learning models, multilayer perceptron (MLP) and convolutional neural networks (CNNs) are constructed to detect precipitation flags and retrieve precipitation rates simultaneously over the Northwest Pacific area. The retrieval results are verified by integrated multisatellite retrievals for GPM. Compared with using a single payload, the results show the retrieval advantages of incorporating two microwave spaceborne payloads (microwave humidity and temperature sounder and microwave radiation imager). In addition, each separated task in multitasking deep learning models can outperform the single-task models. The MAE values of MLP and CNN are decreased by 0.05 and 0.04 mm/h, respectively. Nevertheless, it is still a challenge to extend the application of these models, which demands extracting the features learned from a certain area to other areas characterized by different precipitation features. A flexible network framework is developed with two transfer learning (TL) methods (freeze and fine-tuning) to tackle this problem. The pretrained models (MLP and CNN) are trained from the Northwest Pacific area, whereas the transferred models are applied to the Northeast Pacific area. The encouraging results show that the performance of the TL model using the fine-tune method has exceeded that of the comparison model without TL, which is trained by four times more data. The main advantage of using TL is the time reduction and efficiency improvements.
更多查看译文
关键词
Data models,Task analysis,Satellites,Transfer learning,Microwave theory and techniques,Feature extraction,Deep learning,Deep learning (DL),microwave humidity and temperature sounder (MWHTS),microwave radiation imager (MWRI),precipitation retrieval,transfer learning (TL)
AI 理解论文
溯源树
样例
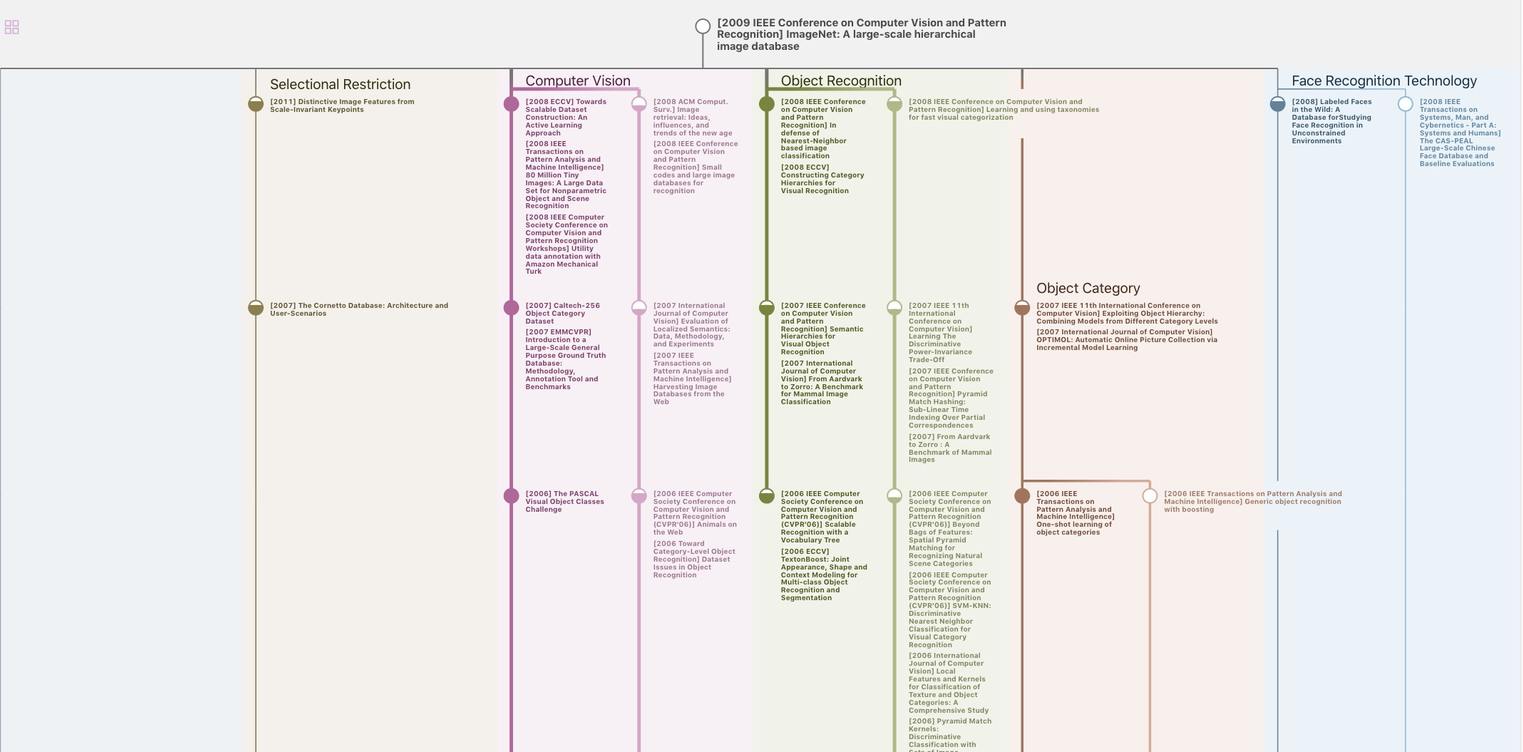
生成溯源树,研究论文发展脉络
Chat Paper
正在生成论文摘要