Point attention network for point cloud semantic segmentation
Science China Information Sciences(2022)
摘要
We address the point cloud semantic segmentation problem through modeling long-range dependencies based on the self-attention mechanism. Existing semantic segmentation models generally focus on local feature aggregation. By comparison, we propose a point attention network (PA-Net) to selectively extract local features with long-range dependencies. We specially devise two complementary attention modules for the point cloud semantic segmentation task. The attention modules adaptively integrate the semantic inter-dependencies with long-range dependencies. Our point attention module adaptively integrates local features of the last layer of the encoder with a weighted sum of the long-range dependency features. Regardless of the distance of similar features, they are all correlated with each other. Meanwhile, the feature attention module adaptively integrates inter-dependent feature maps among all local features in the last layer of the encoder. Extensive results prove that our two attention modules together improve the performance of semantic segmentation on point clouds. We achieve better semantic segmentation performance on two benchmark point cloud datasets (i.e., S3DIS and ScanNet). Particularly, the IoU on 11 semantic categories of S3DIS is significantly boosted.
更多查看译文
关键词
point cloud,semantic segmentation,self-attention mechanism,deep learning,long-range dependencies
AI 理解论文
溯源树
样例
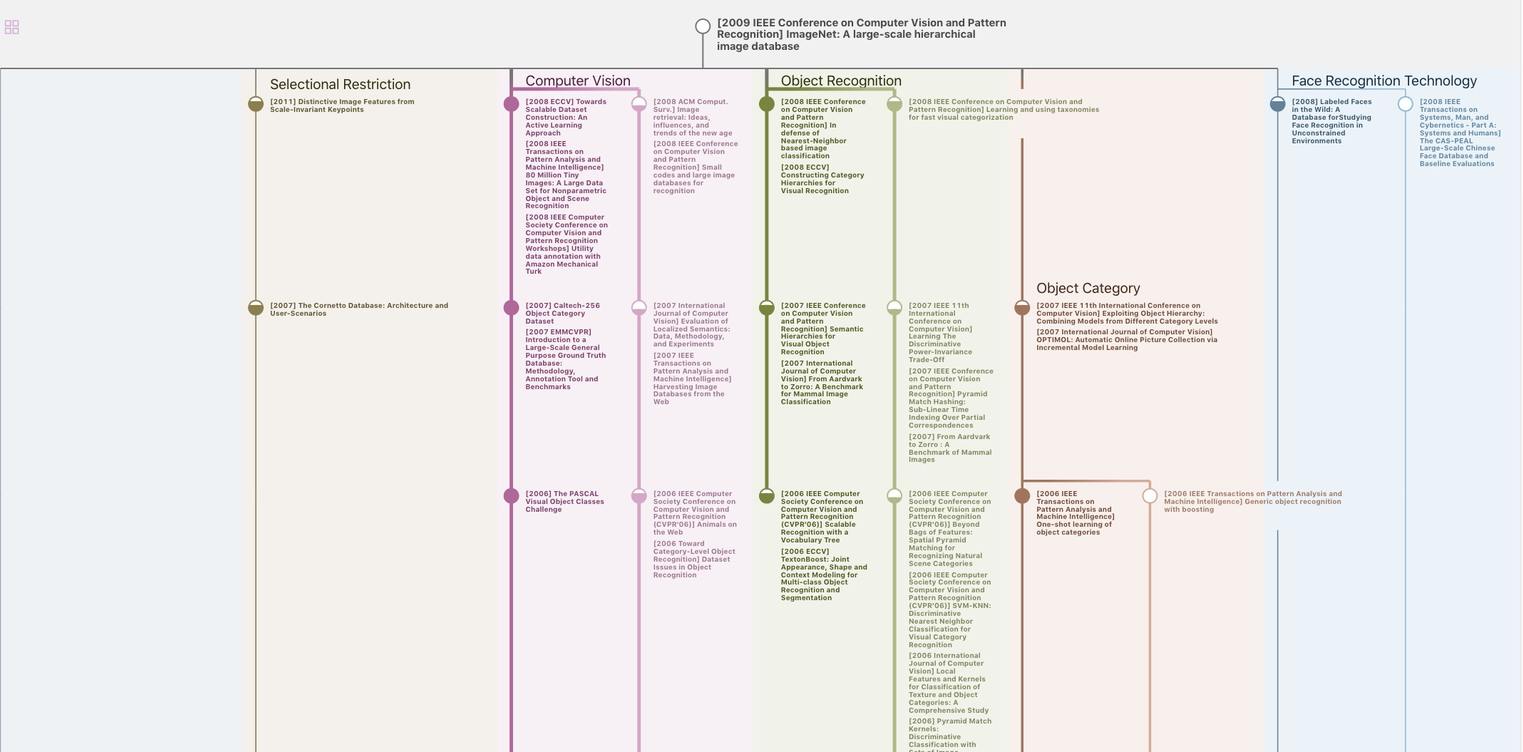
生成溯源树,研究论文发展脉络
Chat Paper
正在生成论文摘要