PFilter: Building Persistent Maps through Feature Filtering for Fast and Accurate LiDAR-based SLAM
IEEE/RJS International Conference on Intelligent RObots and Systems (IROS)(2022)
摘要
Simultaneous localization and mapping (SLAM) based on laser sensors has been widely adopted by mobile robots and autonomous vehicles. These SLAM systems are required to support accurate localization with limited computational resources. In particular, point cloud registration, i.e., the process of matching and aligning multiple LiDAR scans collected at multiple locations in a global coordinate framework, has been deemed as the bottleneck step in SLAM. In this paper, we propose a feature filtering algorithm, PFilter, that can filter out invalid features and can thus greatly alleviate this bottleneck. Meanwhile, the overall registration accuracy is also improved due to the carefully curated feature points. We integrate PFilter into the well-established scan-to-map LiDAR odometry framework, F-LOAM, and evaluate its performance on the KITTI dataset. The experimental results show that PFilter can remove about 48.4% of the points in the local feature map and reduce feature points in scan by 19.3% on average, which save 20.9% processing time per frame. In the mean time, we improve the accuracy by 9.4%.
更多查看译文
关键词
autonomous vehicles,building persistent maps,computational resources,F-LOAM,feature filtering algorithm,global coordinate framework,KITTI dataset,laser sensors,LiDAR-based SLAM,local feature map,mobile robots,PFilter,point cloud registration,registration accuracy,scan-to-map LiDAR odometry framework,SLAM systems
AI 理解论文
溯源树
样例
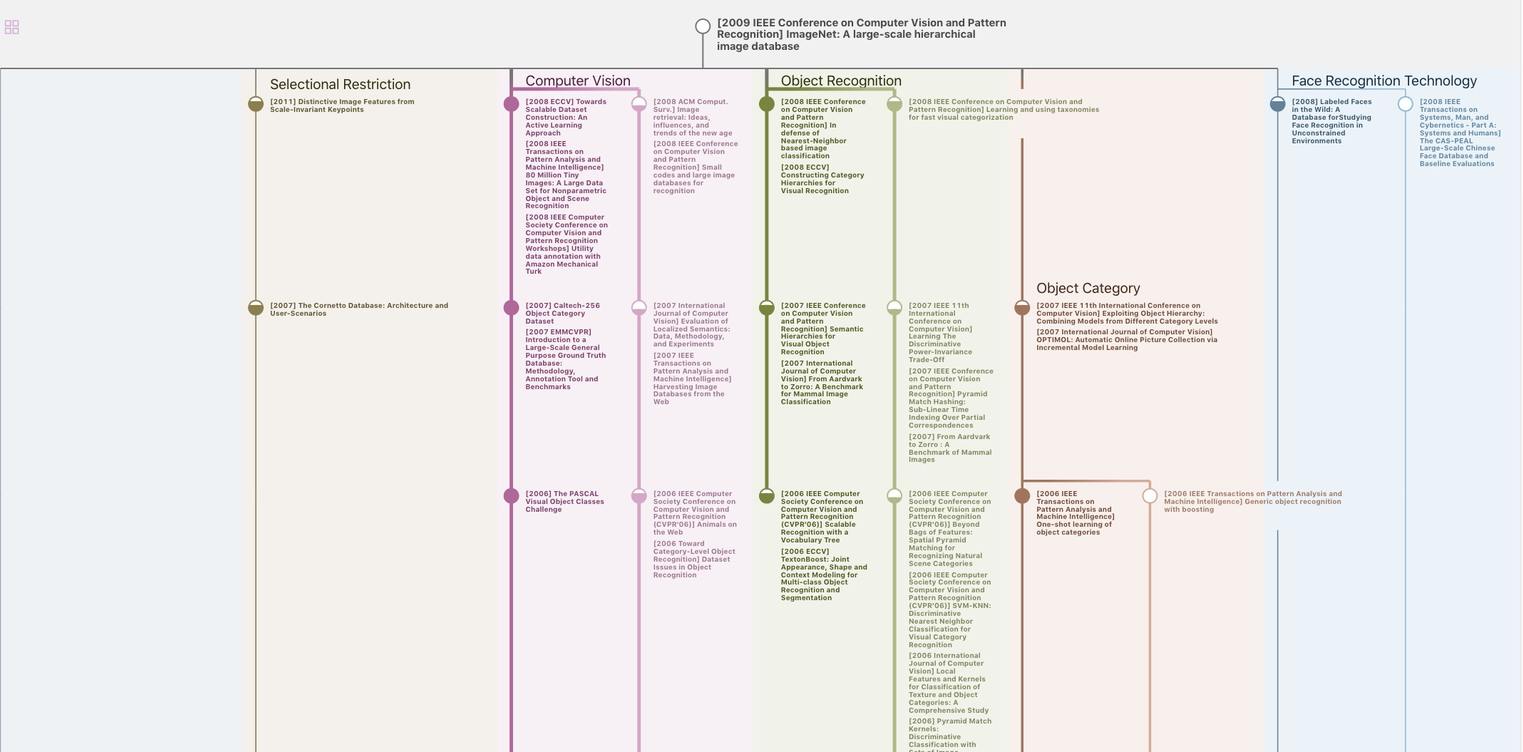
生成溯源树,研究论文发展脉络
Chat Paper
正在生成论文摘要