Batched Bayesian Optimization for Drug Design in Noisy Environments.
Journal of Chemical Information and Modeling(2022)
摘要
The early stages of the drug design process involve identifying compounds with suitable bioactivities via noisy assays. As databases of possible drugs are often very large, assays can only be performed on a subset of the candidates. Selecting which assays to perform is best done within an active learning process, such as batched Bayesian optimization, and aims to reduce the number of assays that must be performed. We compare how noise affects different batched Bayesian optimization techniques and introduce a retest policy to mitigate the effect of noise. Our experiments show that batched Bayesian optimization remains effective, even when large amounts of noise are present, and that the retest policy enables more active compounds to be identified in the same number of experiments.
更多查看译文
关键词
bayesian optimization,drug design
AI 理解论文
溯源树
样例
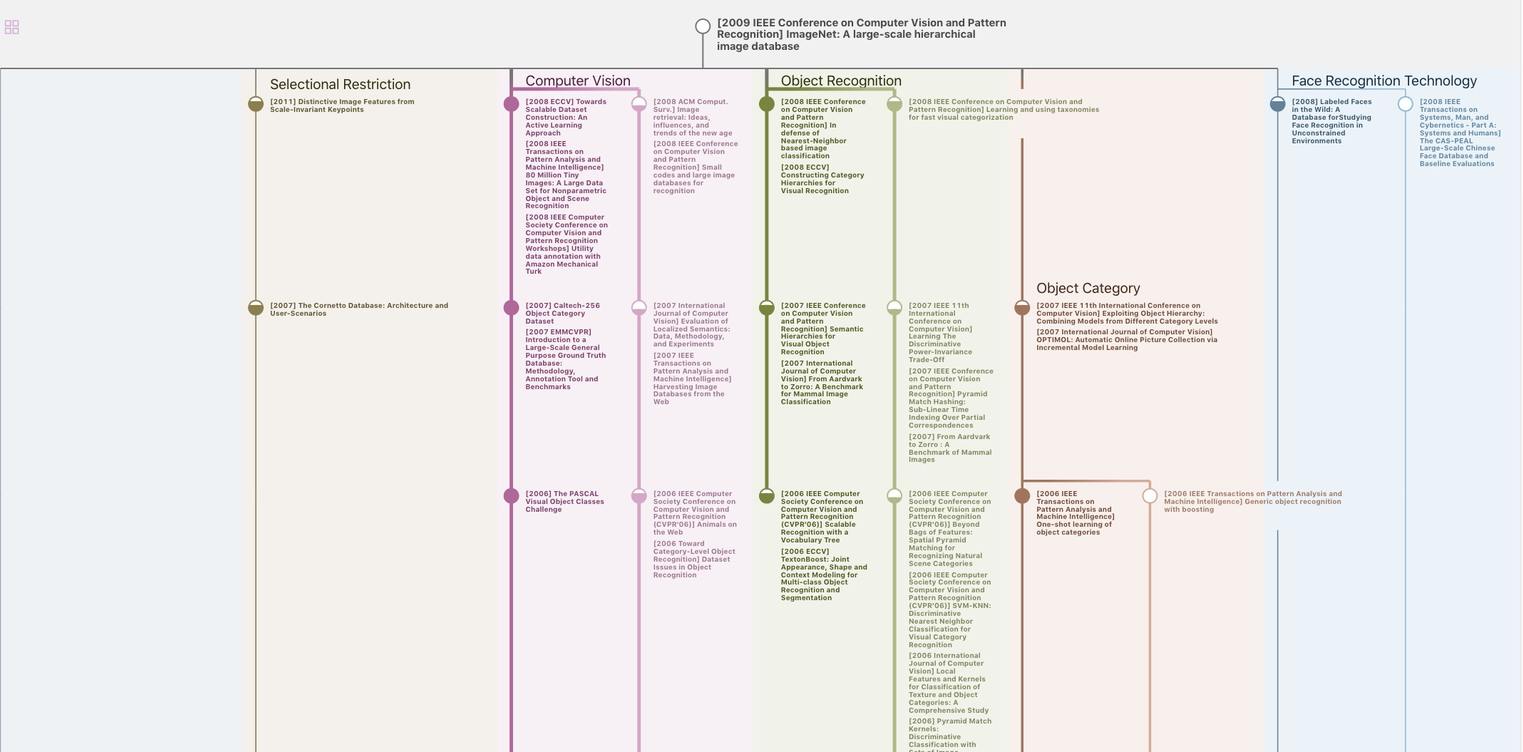
生成溯源树,研究论文发展脉络
Chat Paper
正在生成论文摘要