User Cold-start Recommendation via Inductive Heterogeneous Graph Neural Network
ACM Transactions on Information Systems(2022)
摘要
In recent years, user cold-start recommendations have attracted a lot of attention from industry and academia. In user cold-start recommendation systems, the user attribute information is often used by existing approaches to learn user preferences due to the unavailability of the user action data. However, most existing recommendation methods often ignore the sparsity of user attributes in cold-start recommendation systems. To tackle this limitation, this paper proposes a novel Inductive Heterogeneous Graph Neural Network (IHGNN) model, which utilizes the relational information in user cold-start recommendation systems to alleviate the sparsity of user attributes. Our model converts new users, items, associated multimodal information into a Modality-aware Heterogeneous Graph (M-HG), which preserves the rich and heterogeneous relationship information among them. Specifically, to utilize rich and heterogeneous relational information in a M-HG for enriching the sparse attribute information of new users, we design a strategy, which basically is based on random walk operations, to collect associated neighbors of new users by multiple times sampling operation. Then, a well-designed multiple hierarchical attention aggregation model consisting of the intra- and inter-type attention aggregating module is proposed, focusing on useful connected neighbors and neglecting meaningless and noisy connected neighbors to generate high-quality representations for user cold-start recommendations. Experimental results on three real data sets demonstrate that IHGNN outperforms the state-of-the-art baselines.
更多查看译文
关键词
Multimodal,Heterogeneous Graph,user cold-start recommendation
AI 理解论文
溯源树
样例
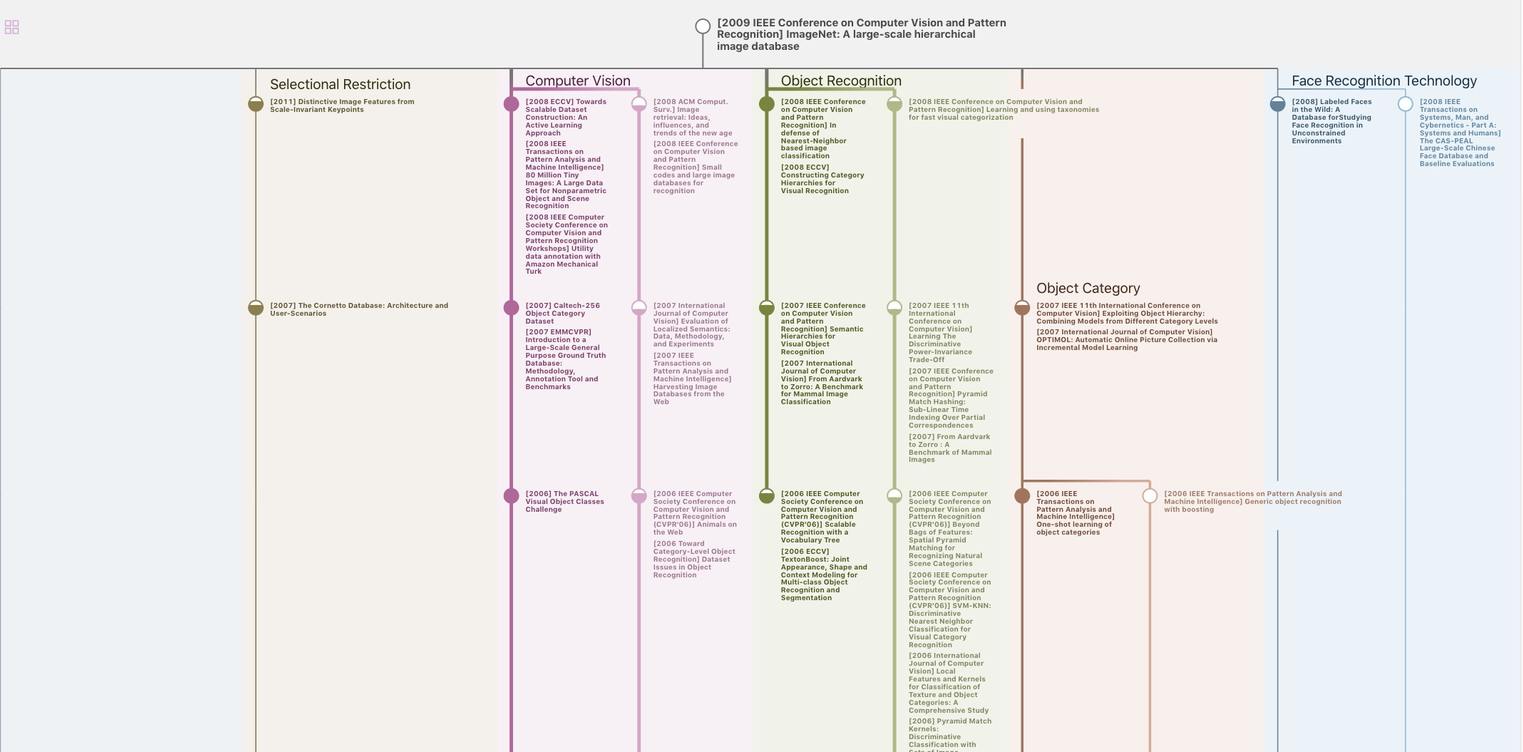
生成溯源树,研究论文发展脉络
Chat Paper
正在生成论文摘要