End-to-End Incomplete Multiview Fuzzy Clustering With Adaptive Missing View Imputation and Cooperative Learning
IEEE Transactions on Fuzzy Systems(2023)
摘要
The purpose of multiview fuzzy clustering is to integrate fuzzy partitions of multiple complete views and obtain the optimal clustering partition. However, multiview data collected in the real world are often incomplete. To address this problem, partial view-based methods and missing view imputation-based methods have been proposed in recent years and achieved some success. However, these two groups of methods still have the following issues. First, since missing view processing and clustering are handled separately, the processed data lack relevance for clustering. Second, either hidden or visible information is explored for clustering, which precludes cooperative learning between these two types of information. Third, the within view and between view information is not fully explored. This article proposes a new end-to-end incomplete multiview fuzzy clustering method to deal with the issues. Based on traditional multiview fuzzy clustering, we construct a new end-to-end clustering framework to integrate the three tasks-missing view imputation, hidden view learning and clustering-as a single process. The framework not only enables mutual coordination of the three tasks, but also cooperative learning between the hidden and visible views. Next, we explore the within and between view information and propose two enhanced learning mechanisms to improve the quality of the imputed missing views and the learned hidden views. Finally, we introduce an adaptive view weighting mechanism to further improve the robustness of the model. Experiments on real-world datasets demonstrate the superiority of the proposed method.
更多查看译文
关键词
Cooperative learning,end-to-end learning,fuzzy clustering,incomplete multiview,missing view imputation
AI 理解论文
溯源树
样例
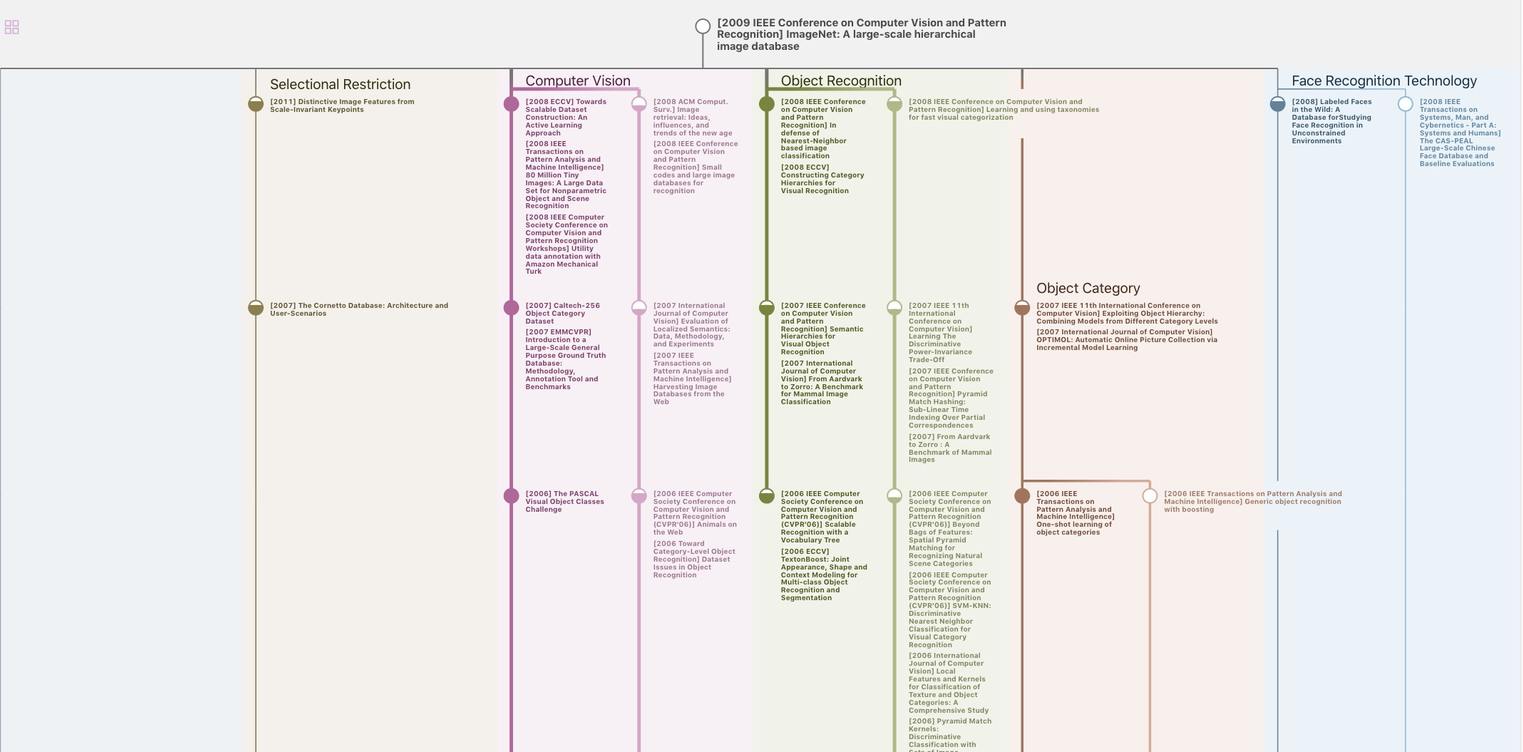
生成溯源树,研究论文发展脉络
Chat Paper
正在生成论文摘要