Angle based hand gesture recognition using graph convolutional network
COMPUTER ANIMATION AND VIRTUAL WORLDS(2024)
摘要
Hand gesture recognition has attracted huge interest in the areas of autonomous driving, human computer systems, gaming and many others. Skeleton based techniques along with graph convolutional networks (GCNs) are being popularly used in this field due to the easy estimation of joint coordinates and better representation capability of graphs. Simple hand skeleton graphs are unable to capture the finer details and complex spatial features of hand gestures. To address these challenges, this work proposes an "angle-based hand gesture graph convolutional network" (AHG-GCN). This model introduces two additional types of novel edges in the graph to connect the wrist with each fingertip and finger's base, explicitly capturing their relationship, which plays an important role in differentiating gestures. Besides, novel features for each skeleton joint are designed using the angles formed with fingertip/finger-base joints and the distance among them to extract semantic correlation and tackle the overfitting problem. Thus, an enhanced set of 25 features for each joint is obtained using these novel techniques. The proposed model achieves 90% and 88% accuracy for 14 and 28 gesture configurations for the DHG 14/28 dataset and, 94.05% and 89.4% accuracy for 14 and 28 gesture configurations for the SHREC 2017 dataset, respectively.
更多查看译文
关键词
convolutional neural network (CNN),deep learning,graph convolutional network (GCN),hand gesture recognition
AI 理解论文
溯源树
样例
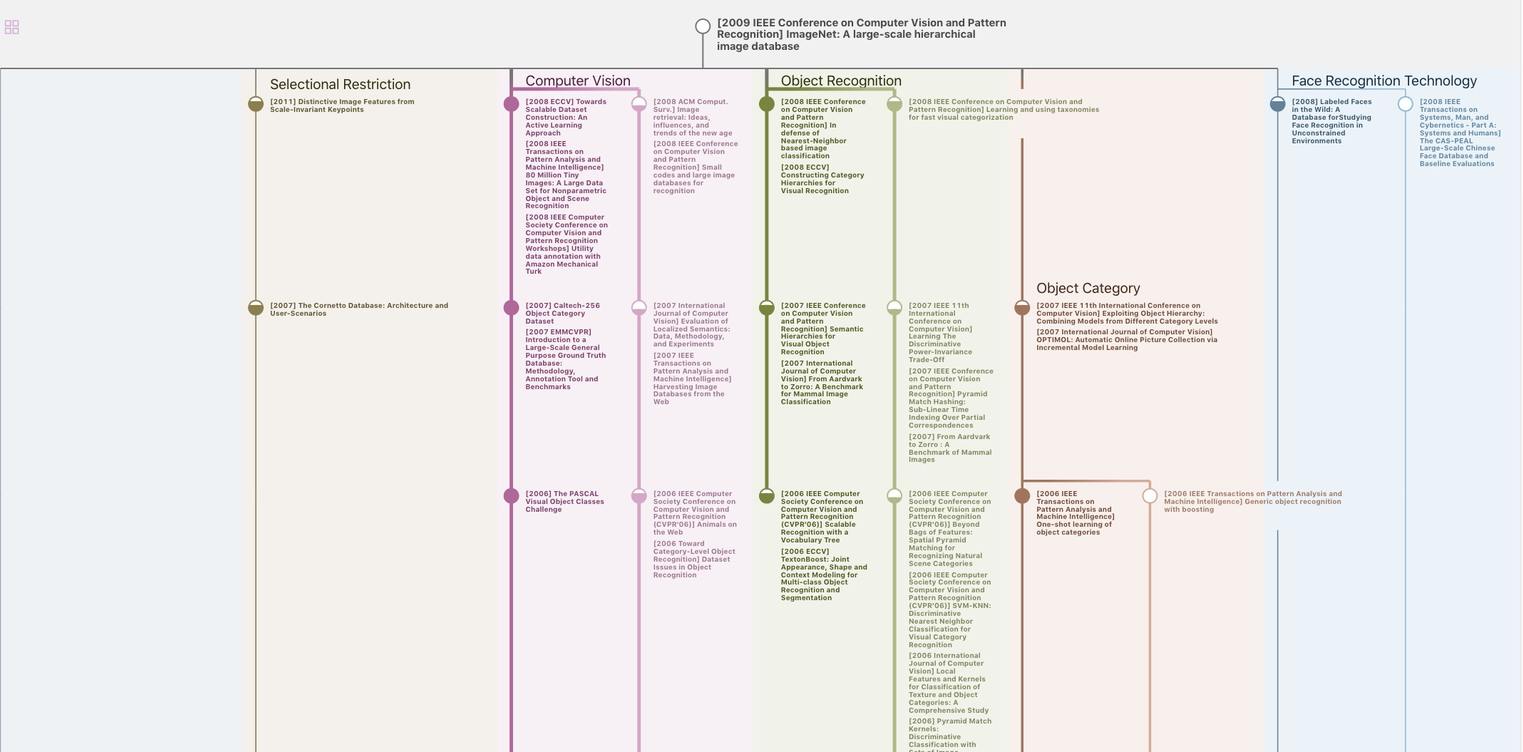
生成溯源树,研究论文发展脉络
Chat Paper
正在生成论文摘要