Accurate water quality prediction with attention-based bidirectional LSTM and encoder-decoder
EXPERT SYSTEMS WITH APPLICATIONS(2024)
摘要
Accurate prediction of water quality indicators can effectively predict sudden water pollution events and reveal them to water users for reducing the impact of water quality pollution. Neural networks, e.g., Long Short-Term Memory (LSTM) and encoder-decoder, have been widely used to predict time series data. However, as the water quality data increases, it becomes unstable and highly nonlinear, and therefore, its accurate prediction becomes a big challenge. To solve it, this work proposes a hybrid prediction method called VBAED to predict the water quality time series. VBAED combines Variational mode decomposition (VMD), a Bidirectional input Attention mechanism, an Encoder with bidirectional LSTM (BiLSTM), and a Decoder with a bidirectional temporal attention mechanism and BiLSTM. The definition of VBAED is an Encoder-Decoder model that uses VMD as mode decomposition, combining BiLSTM with a bidirectional attention mechanism. Specifically, VBAED first adopts VMD to decompose historical data of a predicted factor, and its decomposed results are adopted as the input along with other features. Then, a bidirectional input attention mechanism is adopted to add weights to input features from both directions. VBAED adopts BiLSTM as an encoder to extract hidden features from input features. Finally, the predicted result is obtained by a BiLSTM decoder with a bidirectional temporal attention mechanism. Real-life data-based experiments demonstrate that VBAED obtains the best prediction results compared with other widely used methods.
更多查看译文
关键词
Water quality prediction,Variational mode decomposition,BiLSTM,Attention mechanisms,Encoder-decoder
AI 理解论文
溯源树
样例
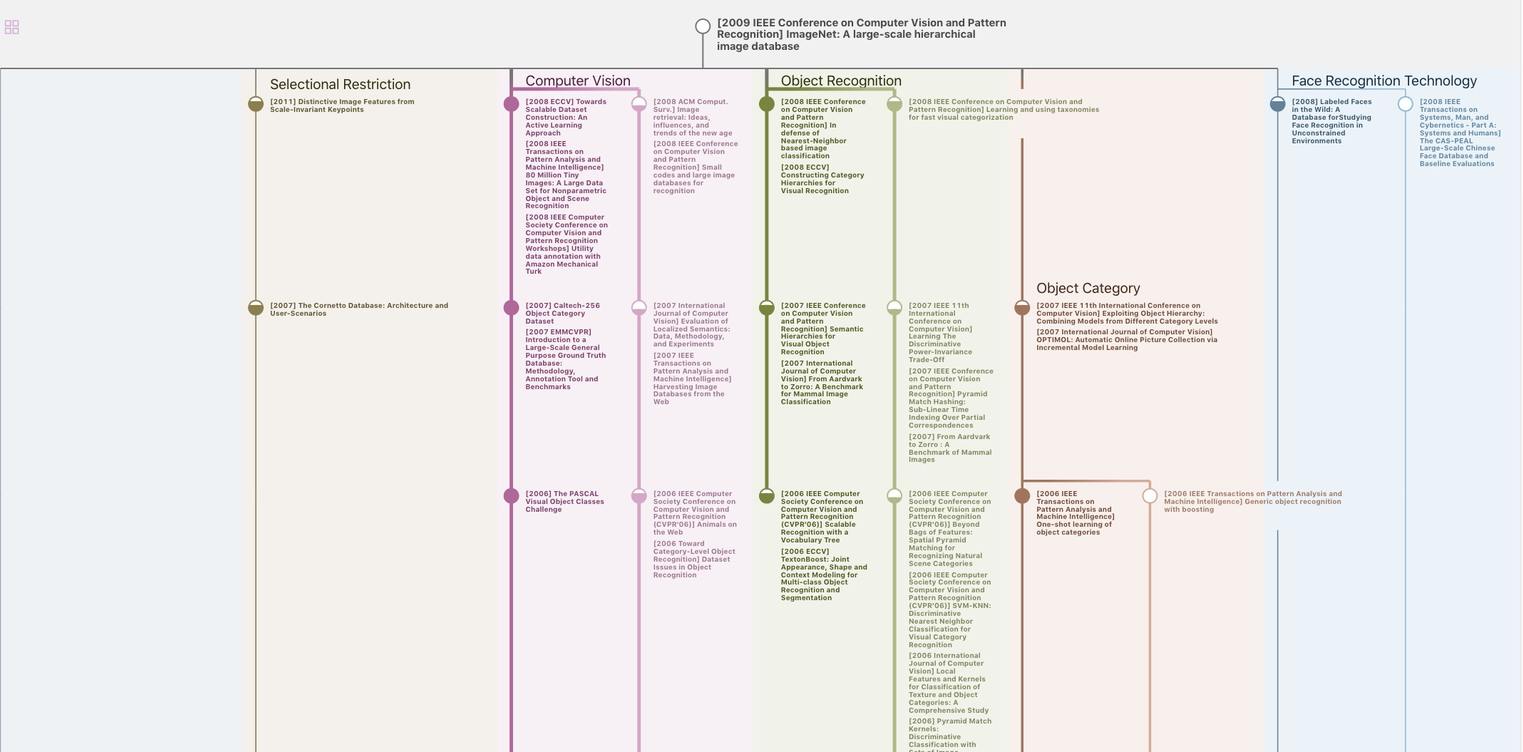
生成溯源树,研究论文发展脉络
Chat Paper
正在生成论文摘要