Improved network intrusion classification with attention-assisted bidirectional LSTM and optimized sparse contractive autoencoders
EXPERT SYSTEMS WITH APPLICATIONS(2024)
摘要
Accurately identifying network intrusion cannot only help individuals and enterprises better deal with network security problems, but also maintain the Internet environment. This work proposes a new hybrid classification method named SABD for network intrusion detection. SABD integrates Stacked sparse contractive autoencoders (SSCA), Attention-based Bidirectional long-term and short-term memory (LSTM), and Decision fusion. Specifically, SSCA is used for extracting features, which are sent to the attention-based bidirectional LSTM for the classification. Besides, an improved optimization algorithm named genetic simulated-annealing-based particle swarm optimization is designed to optimize hyperparameters of SSCA. Finally, the decision fusion algorithm is adopted to integrate classification results of multiple classifiers and yield the final results. Based on experimental results from four different types of data sets, the proposed SABD outperforms its most advanced peers in classification accuracy.
更多查看译文
关键词
Network intrusion detection,Feature extraction,Long-term and short-term memory,Autoencoders,Decision fusion
AI 理解论文
溯源树
样例
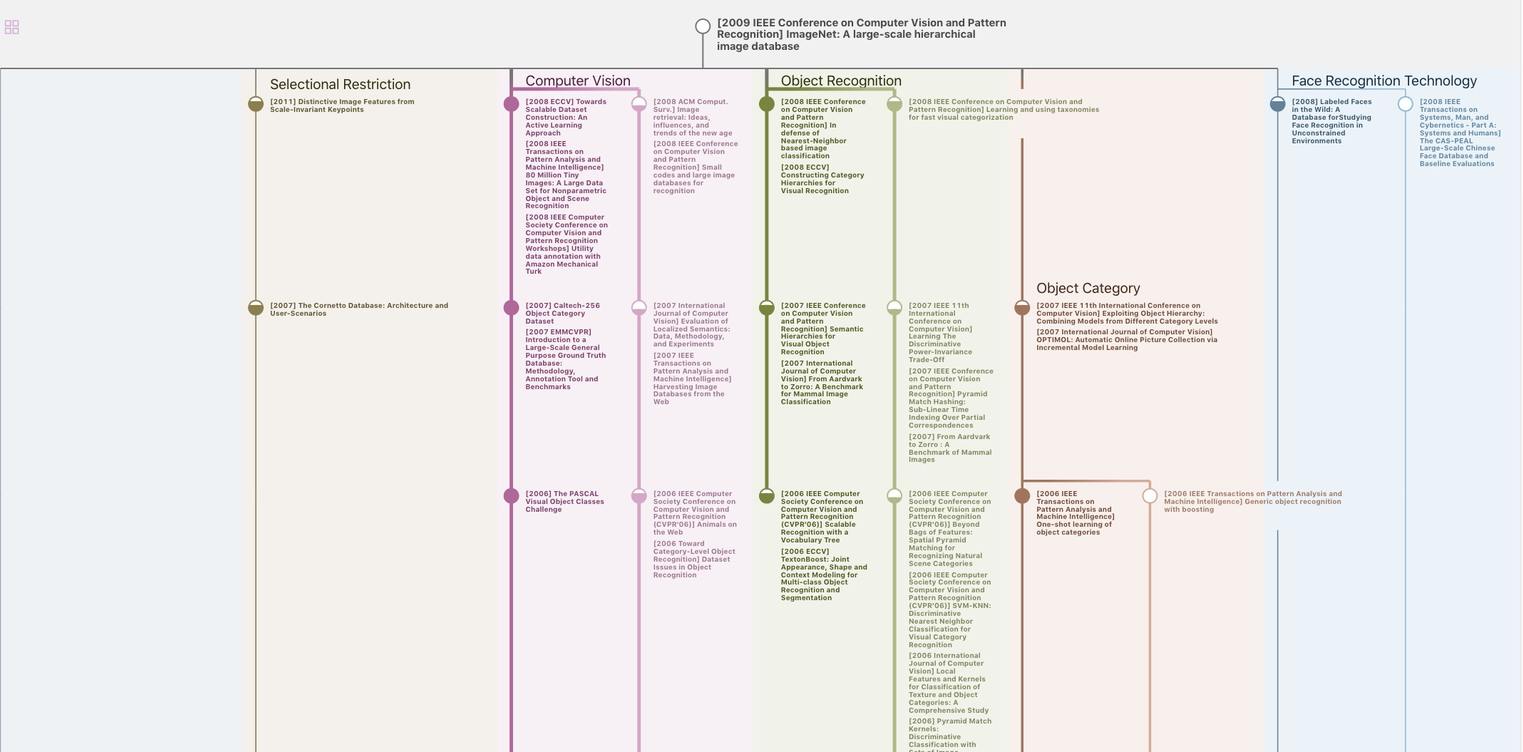
生成溯源树,研究论文发展脉络
Chat Paper
正在生成论文摘要