Air quality prediction using spatio-temporal deep learning
Atmospheric Pollution Research(2022)
摘要
With the rapid urbanization, many developing countries are suffering from heavy air pollution. Predicting air quality accurately is critical for people’s outside planning and governments’ policy-making in these countries. This paper studies the air quality dataset collected in 22 stations of Beijing city, China, and reveals the spatial correlations, the temporal dependencies and feature correlations hidden in the dataset. Different from existing shallow or deep learning models that do not fully consider these correlations, we propose a spatio-temporal deep learning model called Conv1D-LSTM, which unifies 1-dimensional convolutional neural network (1D CNN) and long short-term memory (LSTM) for spatial and temporal correlation feature extraction, and uses a fully connected network (FCN) to exploit these features for air quality prediction. Moreover, this study investigates the problem of data missing in the air quality dataset and impute the missing values from spatial and temporal views. Extensive experiments are conducted by applying the proposed model to hourly PM2.5 and PM10 with single and multi-step predictions over the study area during 2014–2015. The results show the effectiveness of the proposed model and its superiority over other baseline models.
更多查看译文
关键词
Spatio-temporal,1D CNN,LSTM,Air quality prediction
AI 理解论文
溯源树
样例
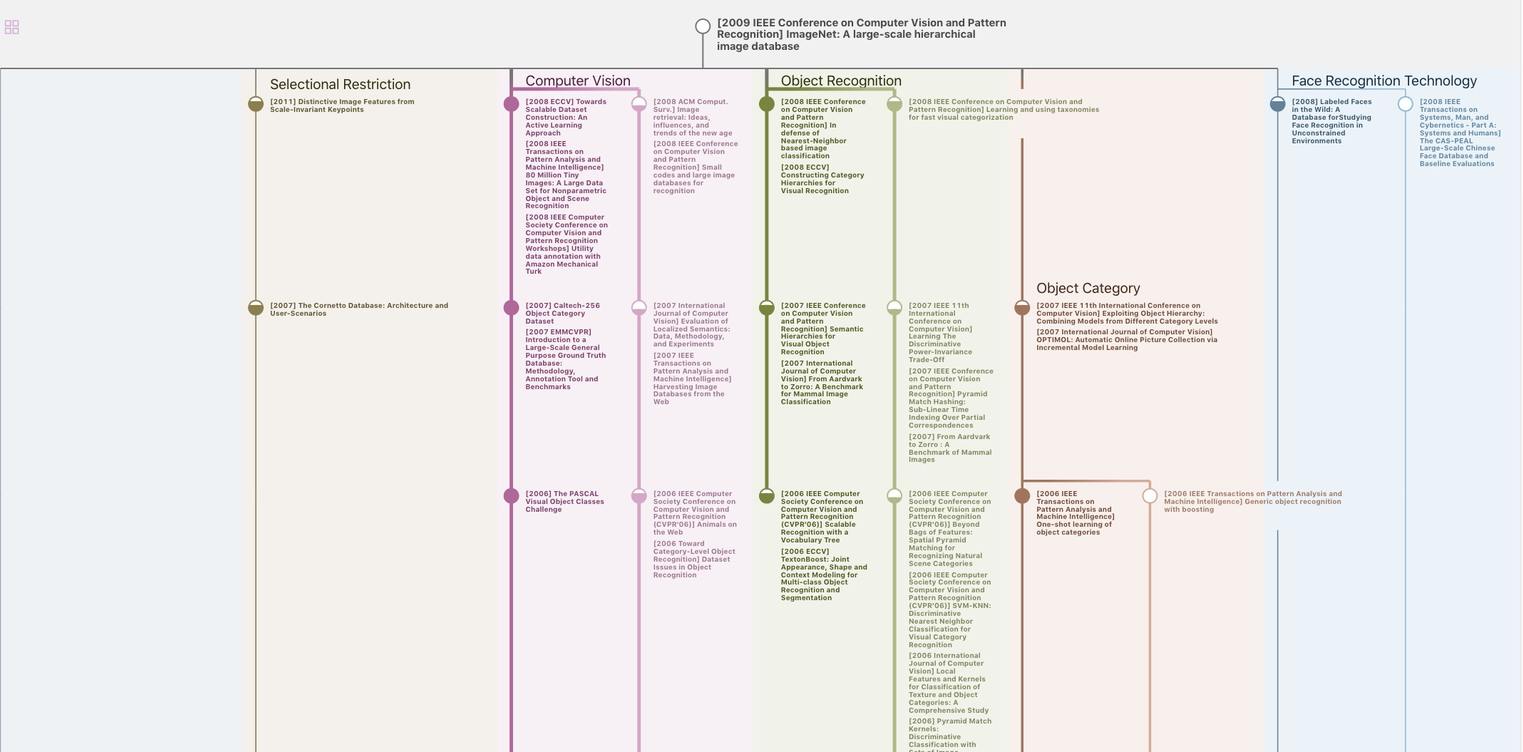
生成溯源树,研究论文发展脉络
Chat Paper
正在生成论文摘要