ARST: Auto-Regressive Surgical Transformer for Phase Recognition from Laparoscopic Videos
arxiv(2022)
摘要
Phase recognition plays an essential role for surgical workflow analysis in computer assisted intervention. Transformer, originally proposed for sequential data modeling in natural language processing, has been successfully applied to surgical phase recognition. Existing works based on transformer mainly focus on modeling attention dependency, without introducing auto-regression. In this work, an Auto-Regressive Surgical Transformer, referred as ARST, is first proposed for on-line surgical phase recognition from laparoscopic videos, modeling the inter-phase correlation implicitly by conditional probability distribution. To reduce inference bias and to enhance phase consistency, we further develop a consistency constraint inference strategy based on auto-regression. We conduct comprehensive validations on a well-known public dataset Cholec80. Experimental results show that our method outperforms the state-of-the-art methods both quantitatively and qualitatively, and achieves an inference rate of 66 frames per second (fps).
更多查看译文
关键词
Surgical workflow analysis,surgical phase recognition,transformer,auto-regression,laparoscopic videos
AI 理解论文
溯源树
样例
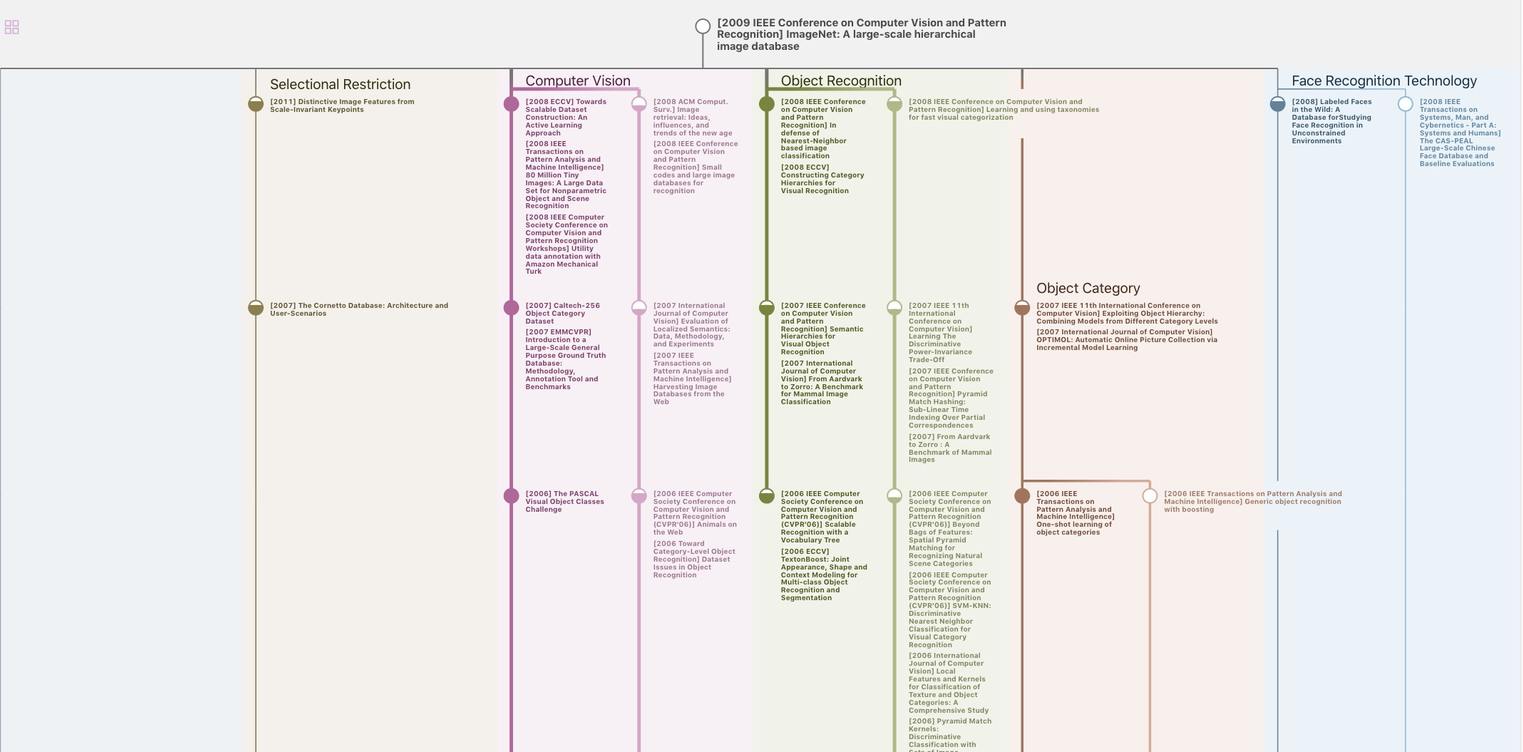
生成溯源树,研究论文发展脉络
Chat Paper
正在生成论文摘要