Event Related Potentials Extraction using Low-rank Tensor Decomposition
2022 30th International Conference on Electrical Engineering (ICEE)(2022)
摘要
Event-related potential (ERP) extraction from ongoing electroencephalograph (EEG) and its enhancement is one of the long-established problems in EEG signal processing. Most of the previous studies have focused mainly on the ERP enhancement without considering the multi-dimentional structure of the signal. In order to take advantage of this property, we propose a tensor-based solution with trial-by-trial concatenated ERP data. Then we develop an algorithm based on low-rank Tucker decomposition to detect single trial ERP component with maximized signal to noise ratio (SNR). In other words, by using tensor algebra we consider both self-similarity in intratrials and global correlation in spatial mode. Applicability of the algorithm is demonstrated in the context of P300 detection with synthetic data and the real EEG data collected from 8 subjects during a visual oddball experiment. Performance of the proposed method is evaluated with approximation errors and classification accuracy.
更多查看译文
关键词
Event Related Potentials (ERPs),Tucker Decomposition,Low-Rank Restoration
AI 理解论文
溯源树
样例
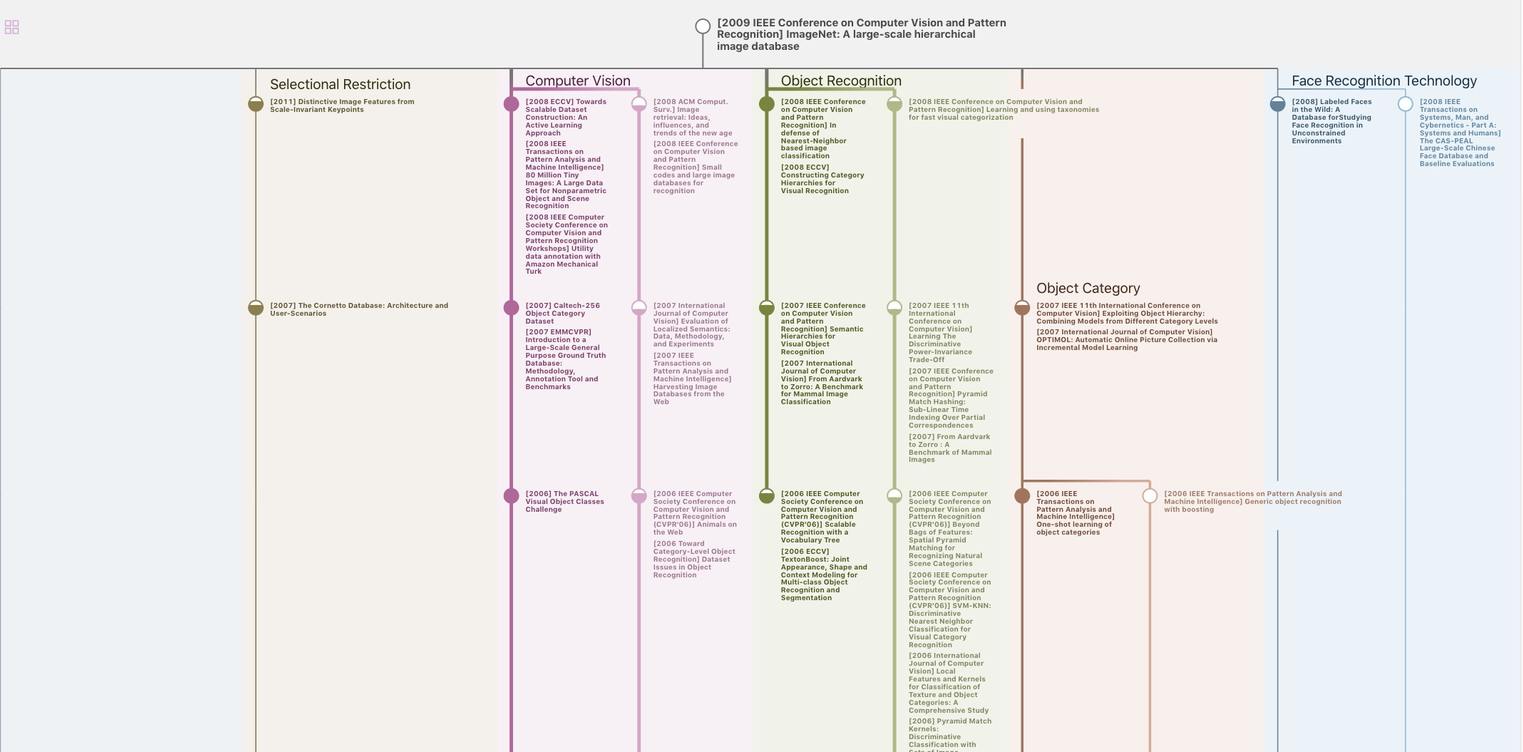
生成溯源树,研究论文发展脉络
Chat Paper
正在生成论文摘要