Supervised Classification of Sound Speed Profiles Via Dictionary Learning
Journal of Atmospheric and Oceanic Technology(2022)
摘要
Abstract The presence of internal waves (IWs) in the ocean alters the isotropic properties of sound speed profiles (SSPs) in the water column. Changes in the SSPs affect underwater acoustics since most of the energy is dissipated into the seabed due to the downward refraction of sound waves. In consequence, variations in the SSP must be considered when modeling acoustic propagation in the ocean. Regularly, empirical orthogonal functions (EOFs) are employed to model and represent SSPs using a linear combination of basis functions that capture the sound speed variability. A different approach is to use dictionary learning (DL) to obtain a learned dictionary (LD) that generates a non-orthogonal set of basis functions (atoms) that generate a better sparse representation. In this paper, the performance of EOFs and LDs are evaluated for sparse representation of SSPs affected by the passing of IWs. In addition, an LD-based supervised framework is presented for SSP classification and is compared with classical learning models. The algorithms presented in this work are trained and tested on data collected from the shallow water experiment 2006. Results show that LDs yield lower reconstruction error than EOFs when using the same number of basis. In addition, overcomplete LDs demonstrate to be a robust method to classify SSPs during low, medium, and high IW activity, reporting comparable and sometimes higher accuracy than standard supervised classification methods.
更多查看译文
关键词
Artificial intelligence,Classification,Machine learning
AI 理解论文
溯源树
样例
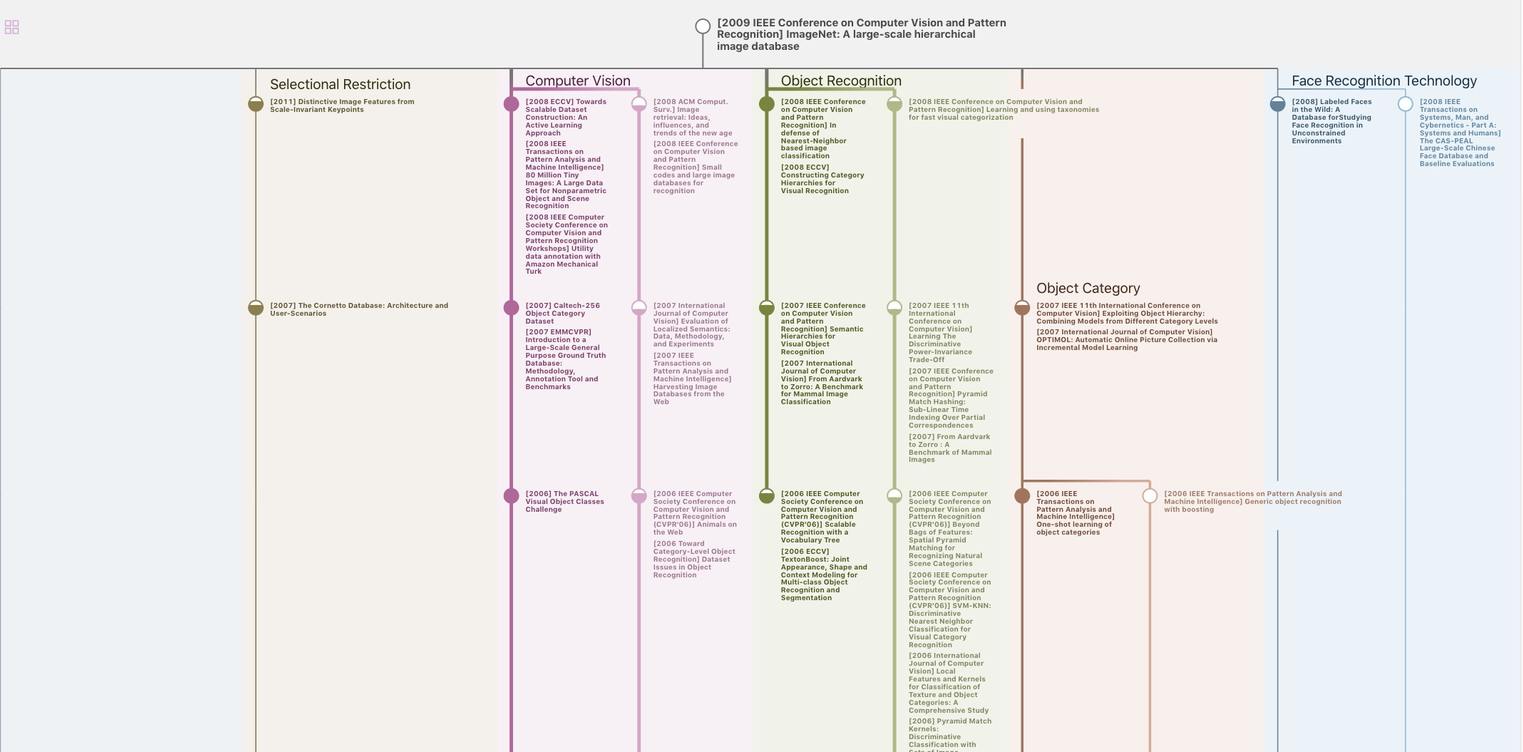
生成溯源树,研究论文发展脉络
Chat Paper
正在生成论文摘要