A semi-supervised learning approach for bladder cancer grading
Machine Learning with Applications(2022)
摘要
Recent advances in semi-supervised learning algorithms (SSL) have made great strides in reducing the training dependency on labeled datasets and requiring that only a subset of the data be labeled. The presented work explores a class of semi-supervised learning algorithms that uses consistency regularization and self-ensembling to leverage the unlabeled portion of the dataset. Labeling medical image datasets are time-consuming and prohibitively expensive, requiring hundreds of hours of effort from expert diagnosticians. This research presents an approach for building and training a deep learning model to grade medical images while requiring only a minimal number of labels. Consistency regularization has been used in SSL to great success in datasets of natural images but not for more complex images such as pathology slides where the dataset consists of cell patterns. This research successfully proposes and applies an SSL algorithm based on the VGG-16 neural network, which combines techniques introduced by the Π model and FixMatch algorithms to a cell pattern-based pathology image dataset. The results presented in this research show that using the proposed approach, it is possible to label only 3% of the samples in a dataset, use the remaining 97% of samples as unlabeled data, and achieve a 19% increase over the baseline accuracy. The second contribution of this research shows a ratio of labeled vs. unlabeled images in a dataset beyond which continuing to label the data increases the cost but offers little performance gains.
更多查看译文
关键词
Artificial intelligence,Deep learning,Semi-supervised learning,Pathology,Medical digital imaging
AI 理解论文
溯源树
样例
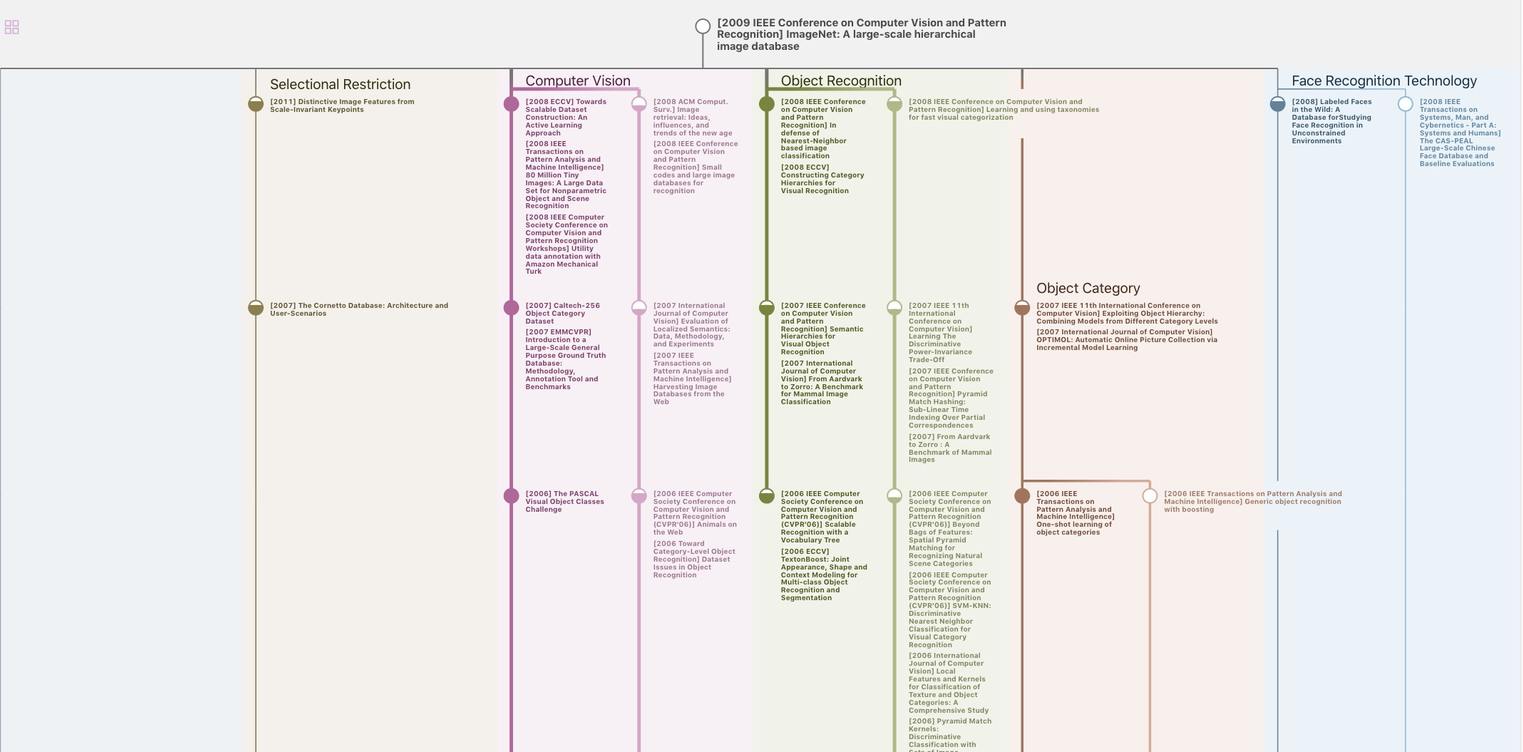
生成溯源树,研究论文发展脉络
Chat Paper
正在生成论文摘要