A Novel Two-Stage Knowledge Distillation Framework for Skeleton-Based Action Prediction
IEEE SIGNAL PROCESSING LETTERS(2022)
摘要
This letter addresses the challenging problem of action prediction with partially observed sequences of skeletons. Towards this goal, we propose a novel two-stage knowledge distillation framework, which transfers prior knowledge to assist the early prediction of ongoing actions. In the first stage, the action prediction model (also referred to as the student) learns from a couple of teachers to adaptively distill action knowledge at different progress levels for partial sequences. Then the learned student acts as a teacher in the next stage, with the objective of optimizing a better action prediction model in a self-training manner. We design an adaptive self-training strategy from the perspective of undermining the supervision from the annotated labels, since this hard supervision is actually too strict for partial sequences without enough discriminative information. Finally, the action prediction models trained in the two stages jointly constitute a two-stream architecture for action prediction. Extensive experiments on the large-scale NTU RGB+D dataset validate the effectiveness of the proposed method.
更多查看译文
关键词
Adaptation models,Predictive models,Skeleton,Training,Probability distribution,Three-dimensional displays,Writing,Action prediction,adaptive self-training strategy,knowledge distillation,skeletons
AI 理解论文
溯源树
样例
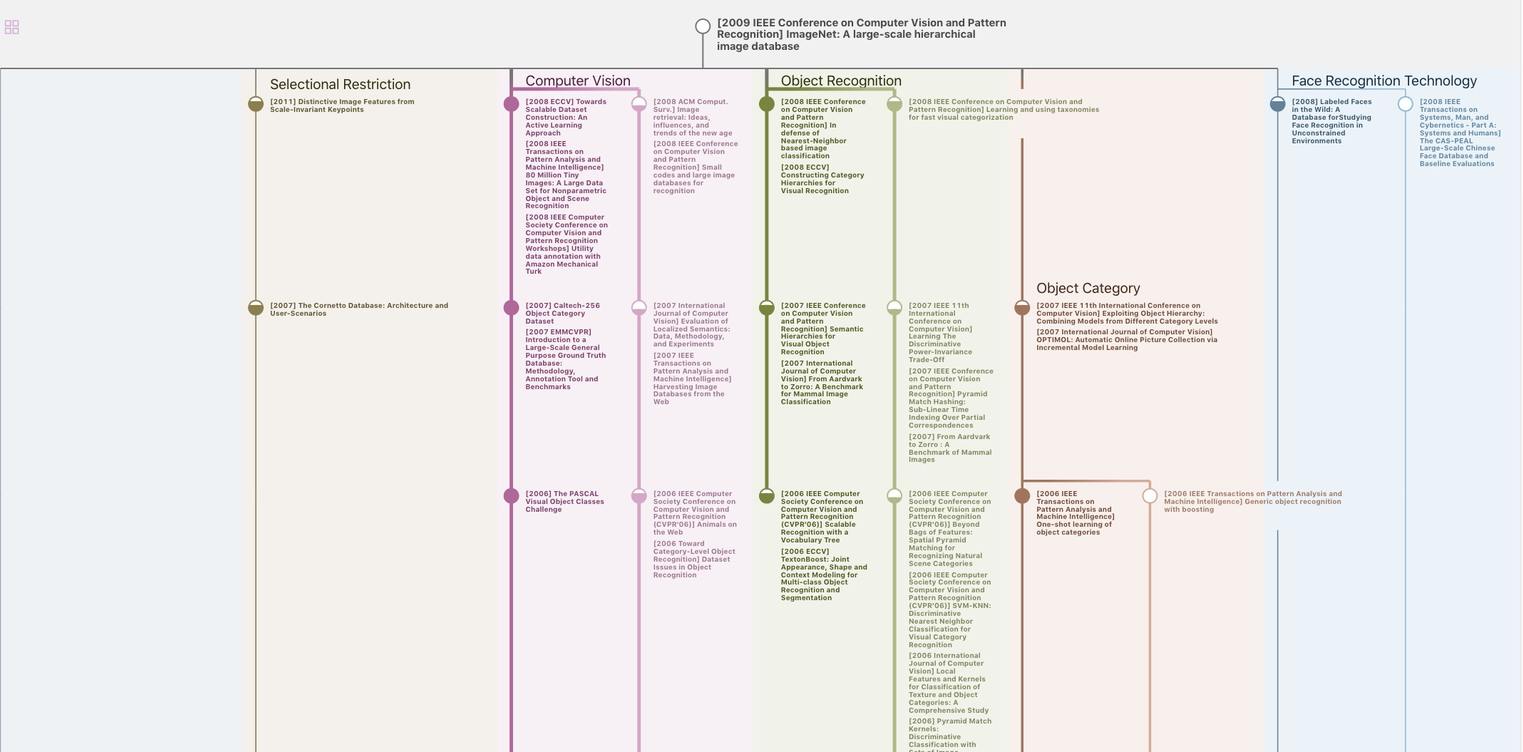
生成溯源树,研究论文发展脉络
Chat Paper
正在生成论文摘要