A Robust Local Texture Descriptor in the Parametric Space of the Weibull Distribution
IEEE TRANSACTIONS ON MULTIMEDIA(2023)
摘要
Research in texture feature approximation is still in the embryonic stage because of difficulties in developing a sound theoretical model to express the unique pattern in the intensity-variation of pixels in the neighbourhood of the pixel-of-interest so that it can sufficiently discriminate different textures. Local texture descriptors are widely used in image segmentation as they comprise pixel-wise features. The Weber local descriptor (WLD) with differential excitation and gradient orientation components, inspired by Weber's Law, has been leveraged in the state-of-the-art iterative contraction and merging (ICM) image segmentation technique. However, WLD has inherent drawbacks in the formulation of the components that limit its discriminatory capability. This paper introduces a novel texture descriptor by directly modelling the distribution of intensity-variation in the parametric space of the Weibull distribution using its shape and scale parameters. A unified 'joint scale' texture property is introduced, which can discriminate textures better than the individual parameters while keeping the length of the descriptor shorter. Additionally, the accuracy of WLD's gradient orientation component is improved by using an extended Sobel operator and expressing gradients in [-pi/2, pi/2) range. When incorporated in ICM, the proposed texture descriptor has consistently outperformed WLD and a recent enhancement with radial mean WLD (RM-WLD) on three benchmark datasets. It has also outperformed two other texture segmentation techniques and their deep learning based improvements.
更多查看译文
关键词
Local texture descriptor,the Weibull distribution,the Sobel operator,image segmentation
AI 理解论文
溯源树
样例
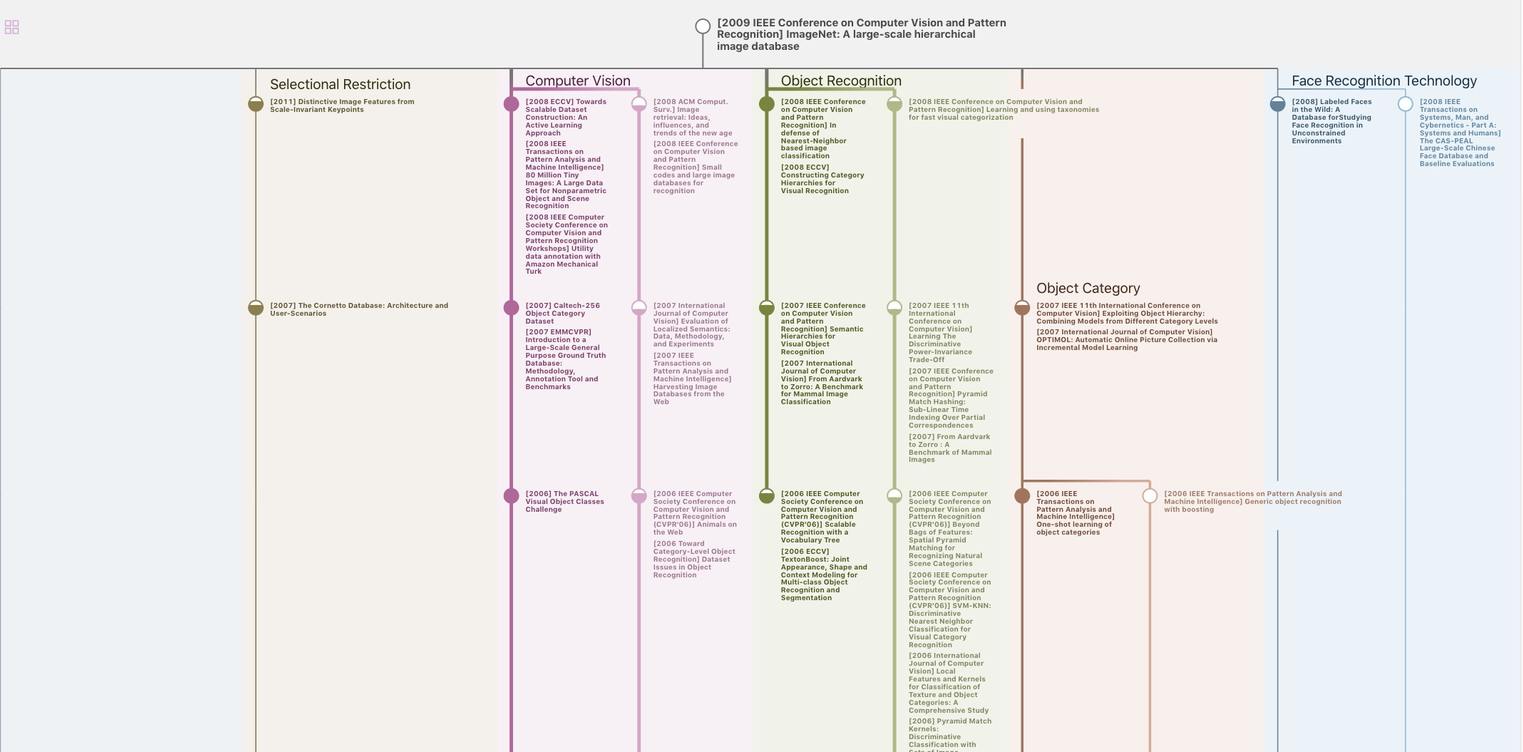
生成溯源树,研究论文发展脉络
Chat Paper
正在生成论文摘要